Cost Model for Near Real-time Recommender Systems
msra
摘要
In this paper, we propose computational foundations of near real-time recommender systems by discussing how computational resources and limits on latency and response time requirements meet in a recommender system. A range of online applications use recommendation engines to help customers find what they need or are interested in, e.g. web shops, news services, and even computer assisted project planning. Such applications focus on slowly changing needs, and give at least locally time-invariant, non-concurrent (or at least non-correlated), or even offline calculated recommendation; hence, they need no real-time modelling of customers' behaviour. Nevertheless, well-known recommendation engines fail whenever large amount of rapid and continuous changes are made over time, e.g. in handling economic events. While offline recommendation engines are widely discussed, characterization of cost components, and modelling of real-time recommendations are missing from the literature. In this paper, we discuss end-to-end data flow, i.e. how to transform and flow data from the source system through a near real-time data warehouse to end in a recommender system. We identify cost components of continuous flow execution in a multidimensional cost space. We determine criteria on how much computational resources are needed based on input data size, latency, and response time requirements. We also discuss the limits of latency and response time requirements given the computational resource constraints.
更多查看译文
关键词
near real-time recommender system,cost model
AI 理解论文
溯源树
样例
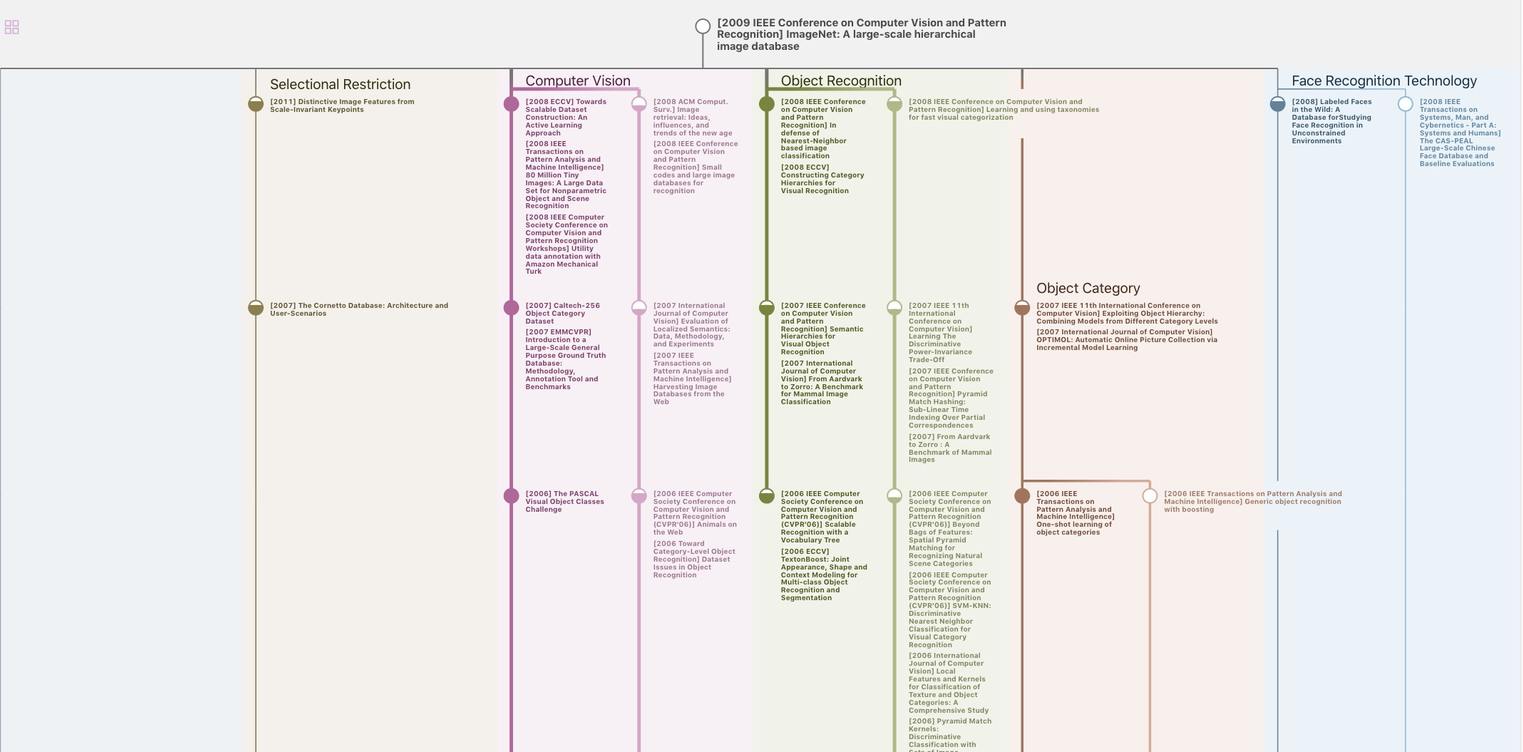
生成溯源树,研究论文发展脉络
Chat Paper
正在生成论文摘要