A New Neural Implementation of Exploratory Projection Pursuit
IDEAL(2002)
摘要
We investigate an extension of the learning rules in a Principal Component Analysis network which has been derived to be optimal for a specific probability density function(pdf). We note that this probability density function is one of a family of pdfs and investigate the learning rules formed in order to be optimal for several members of this family. We show that, whereas previous authors [5] have viewed the single member of the family as an extension of PCA, it is more appropriate to view the whole family of learning rules as methods of performing Exploratory Projection Pursuit(EPP). We explore the performance of our method first in response to an artificial data type, then to a real data set.
更多查看译文
关键词
single member,principal component analysis network,artificial data type,whole family,previous author,specific probability density function,new neural implementation,exploratory projection pursuit,probability density function,principal component analysis,data type,projection pursuit
AI 理解论文
溯源树
样例
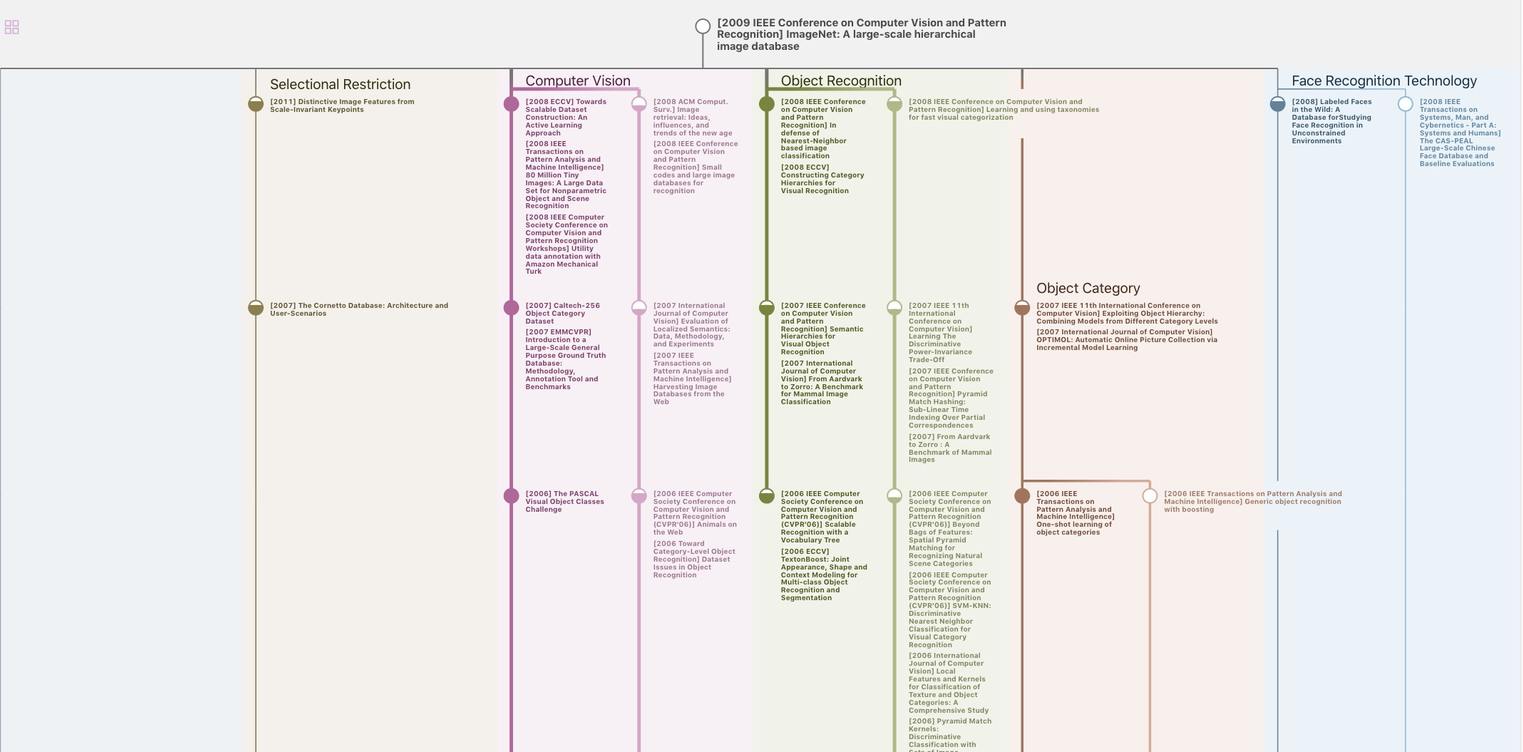
生成溯源树,研究论文发展脉络
Chat Paper
正在生成论文摘要