Collaborative Vs. Conflicting Learning, Evolution And Argumentation
Studies in computational intelligence(2008)
摘要
We discuss the adoption of a three-valued setting for inductive concept learning. Distinguishing between what is true, what is false and what is unknown can be useful in situations where decisions have to be taken on the basis of scarce, ambiguous, or downright contradictory information. In a three-valued setting, we learn a definition for both the target concept and its opposite, considering positive and negative examples as instances of two disjoint classes. Explicit negation is used to represent the opposite concept, while default negation is used to ensure consistency and to handle exceptions to general rules. Exceptions are represented by examples covered by the definition for a concept that belong to the training set for the opposite concept.After obtaining the knowledge resulting from this learning process, an agent can then interact with the environment by perceiving it and acting upon it. However, in order to know what is the best course of action to take the agent must know the causes or explanations of the observed phenomena.Abduction, or abductive reasoning, is the process of reasoning to the best explanations. It is the reasoning process that starts from a set of observations or conclusions and derives their most likely explanations. The term abduction is sometimes used to mean just the generation of hypotheses to explain observations or conclusions, given a theory. Upon observing changes in the environment or in some artifact of which we have a theory, several possible explanations (abductive ones) might come to mind. We say we have several alternative arguments to explain the observations.One single agent exploring an environment may gather only so much information about it and that may not suffice to find the fight explanations. In such case, a collaborative multi-agent strategy, where each agent explores a part of the environment and shares with the others its findings, might provide better results. We describe one such framework based on a distributed genetic algorithm enhanced by a Lamarckian operator for belief revision. The agents communicate their candidate explanations - coded as chromosomes of beliefs - by sharing them in a common pool. Another way of interpreting this communication is in the context of argumentation.We often encounter situations in which someone is trying to persuade us of a point of view by presenting reasons for it. This is called "arguing a case" or "presenting an argument". We can also argue with ourselves. Sometimes it is easy to see what the issues and conclusions are, and the reasons presented, but sometimes not. In the process of taking all the arguments and trying to find a common ground or consensus we might have to change, or review, some of assumptions of each argument. Belief revision is the process of changing beliefs to take into account a new piece of information. The logical formalization of belief revision is researched in philosophy, in databases, and in artificial intelligence for the design of rational agents.The resulting framework we present is a collaborative perspective of argumentation where arguments are put together at work in order to find the possible 2-valued consensus of opposing positions of learnt concepts.
更多查看译文
关键词
conflicting learning,evolution
AI 理解论文
溯源树
样例
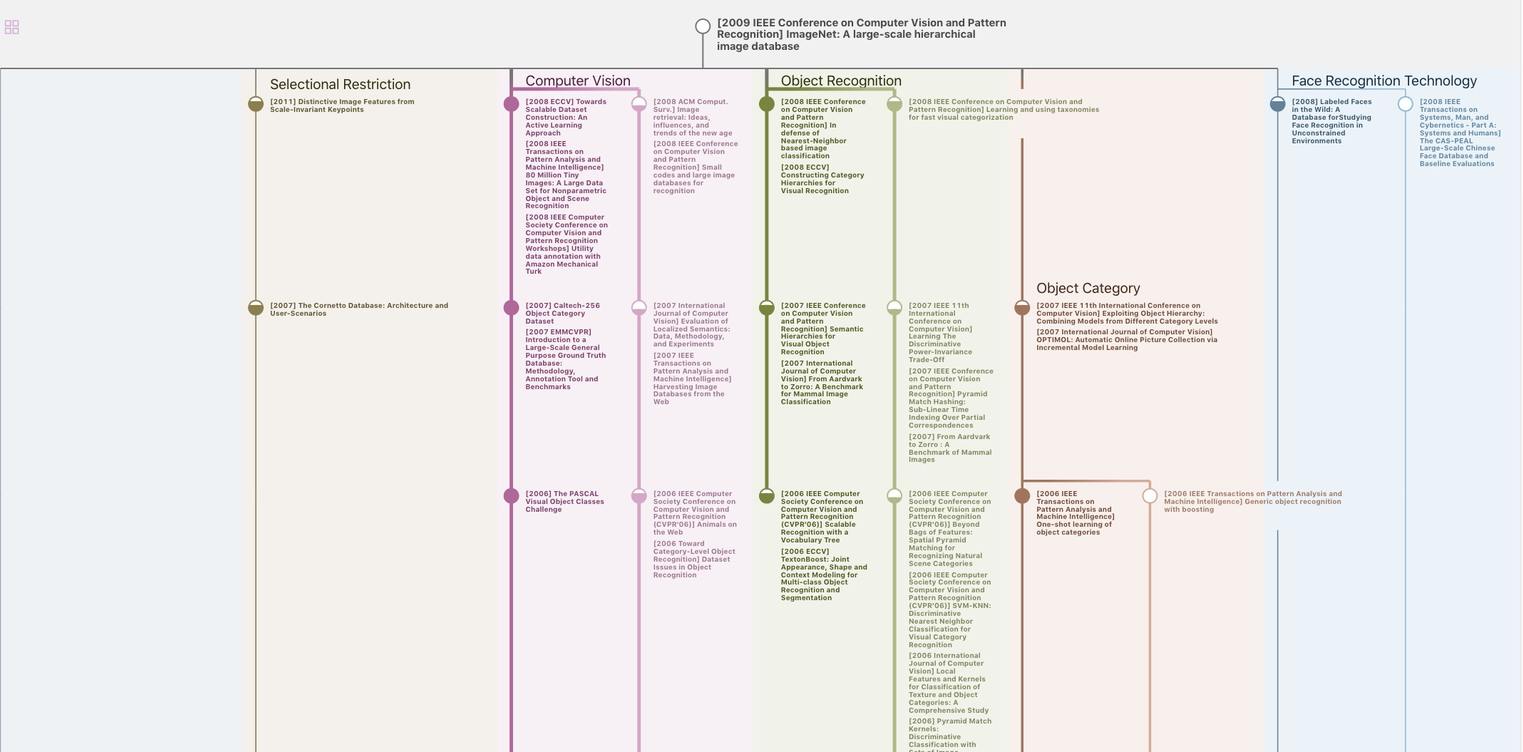
生成溯源树,研究论文发展脉络
Chat Paper
正在生成论文摘要