How important are specialized transforms in Neural Operators?
CoRR(2023)
摘要
Simulating physical systems using Partial Differential Equations (PDEs) has become an indispensible part of modern industrial process optimization. Traditionally, numerical solvers have been used to solve the associated PDEs, however recently Transform-based Neural Operators such as the Fourier Neural Operator and Wavelet Neural Operator have received a lot of attention for their potential to provide fast solutions for systems of PDEs. In this work, we investigate the importance of the transform layers to the reported success of transform based neural operators. In particular, we record the cost in terms of performance, if all the transform layers are replaced by learnable linear layers. Surprisingly, we observe that linear layers suffice to provide performance comparable to the best-known transform-based layers and seem to do so with a compute time advantage as well. We believe that this observation can have significant implications for future work on Neural Operators, and might point to other sources of efficiencies for these architectures.
更多查看译文
关键词
neural operators,transforms
AI 理解论文
溯源树
样例
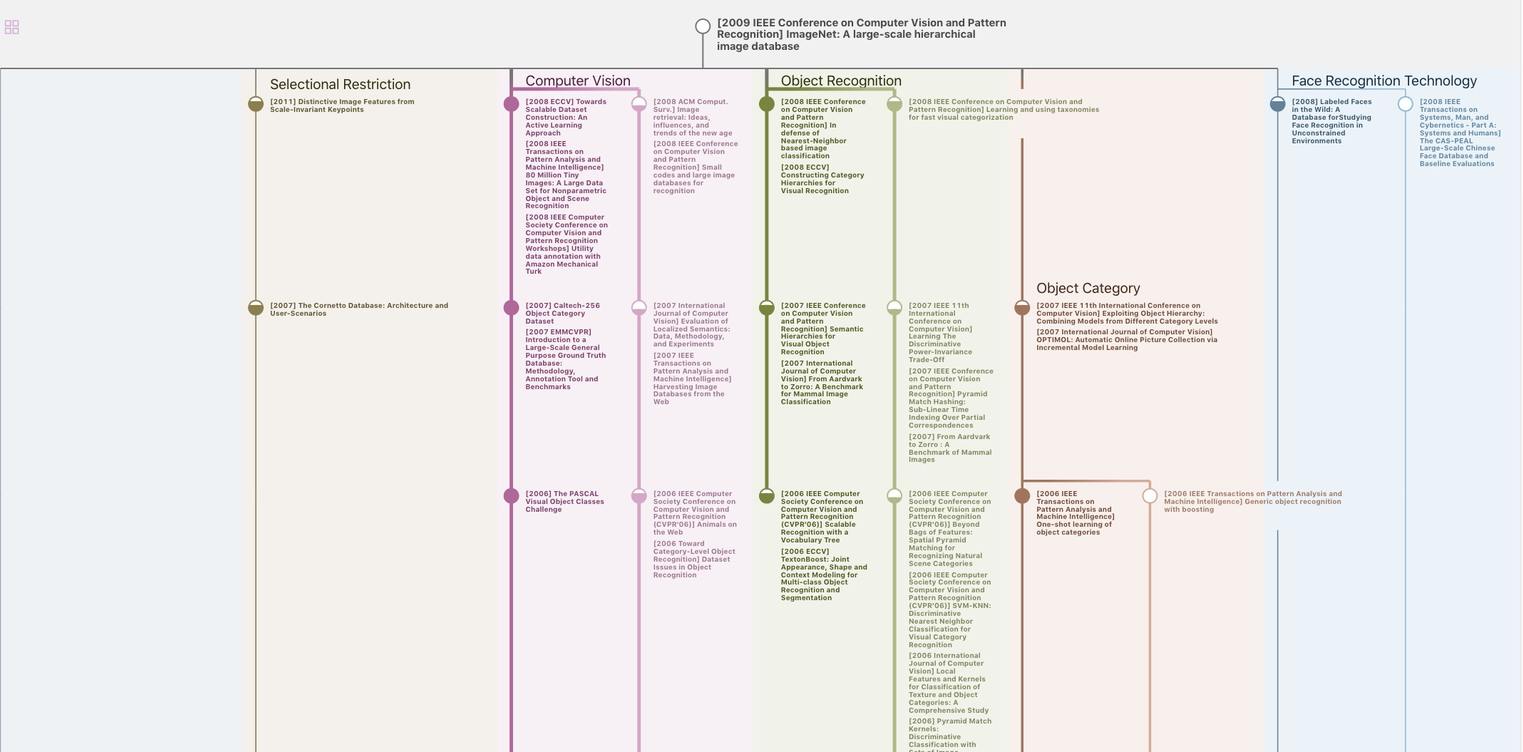
生成溯源树,研究论文发展脉络
Chat Paper
正在生成论文摘要