A neuro-fuzzy approach to classification of human non-synonymous snps based upon computational geometry
A neuro-fuzzy approach to classification of human non-synonymous snps based upon computational geometry(2005)
摘要
The ability to predict the effect of non-synonymous SNPS (nsSNPs) on protein function is important for the success of disease-association studies. Accepting that most diseases are caused by variations in protein expression, folding and/or stability, nsSNPs are the most likely candidates to affect proteins. Sequence-based methods use changes at well-conserved positions to predicted deleterious SNPs, but require a set of not always available orthologous sequences. On the other hand, current structure-based rules strongly rely upon empirical observations. Further, current tools for nsSNP classification using methods such as decision trees, support vector machine and artificial neural network (ANN) provide the user with binary Boolean logic outcome, which is not always sufficient for assessment of nsSNP impacts. Thus there is a need for more comprehensive SNP classification tools. We propose a statistical geometry approach based on Delaunay tessellation to classify disease-associated (daSNPs) and neutral (ntSNPs). Delaunay tessellation provides an objective definition of the nearest neighbors for analysis of protein structure. The composition of simplices generated as a result of tessellation is analyzed in terms of statistical likelihood of occurrence of the four nearest neighbor amino acid residues for all observed quadruplet combinations of the twenty natural amino acids. With this approach, an objective set of characteristics which differentiate daSNPs from ntSNPs have been identified. The most powerful classification characteristic is the difference in total potential between the native protein and its polymorphic variant. To be able to predict the effect of non-synonymous SNPs on protein function we constructed neuro-fuzzy inference system. As an input vector we use the characteristics obtained through Delaunay tessellation and conservation assessment. The merger of ANN with fuzzy logic (FL) yields a system that can learn and is amenable to human perception. In the case of nsSNPs, we show that the FL approach built upon rules derived from statistical geometry leads to a marked improvement in the accuracy of prediction for disease alleles, and provides a comprehensible linguistic determination of output membership. This approach allows us to assess the disease potential of nsSNPs and to select the most promising nsSNPs for further investigation.
更多查看译文
关键词
promising nssnps,statistical geometry approach,computational geometry,protein structure,protein expression,human non-synonymous,delaunay tessellation,protein function,native protein,fl approach,neuro-fuzzy approach,nearest neighbor,non-synonymous snps
AI 理解论文
溯源树
样例
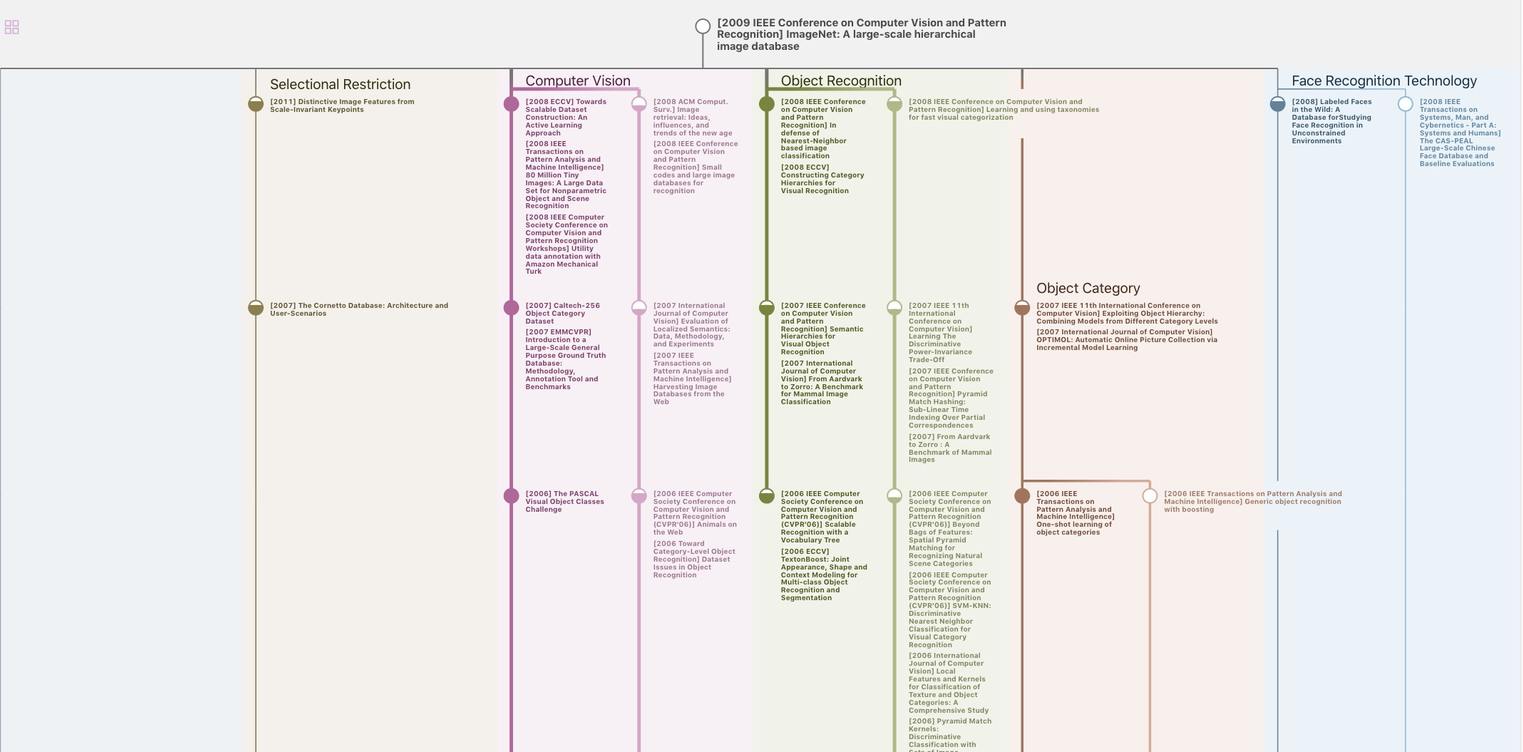
生成溯源树,研究论文发展脉络
Chat Paper
正在生成论文摘要