An geometrically intuitive marginal discriminant analysis method with application to face recognition
ICIP(2009)
摘要
This paper presents a new nonparametric linear feature extraction method coined geometrically intuitive marginal discriminant analysis (IMDA). Motivated by the law of cosines in trigonometry, we characterize the square local margin by a weighted difference of the square between-class distance and the square within-class distance. Based on this characterization, we design a class margin criterion which is used to determine an optimal transform matrix such that the class margin is maximized in the transformed space. The proposed method was applied to face recognition and evaluated on the Yale and the FERET databases. Experimental results demonstrate the effectiveness of the proposed method.
更多查看译文
关键词
feret database,square between- class distance,extraction method,geometrically intuitive marginal discriminant analysis method,face recognition,discriminant analysis,feret databases,visual databases,geometrically intuitive marginal discriminant,yale database,nonparametric linear feature extraction method,dimensionality reduction,optimal transform matrix,class margin criterion,feature extraction,square between-class distance,analysis method,square within-class distance,class margin,square local margin,trigonometry,databases,image recognition,principal component analysis
AI 理解论文
溯源树
样例
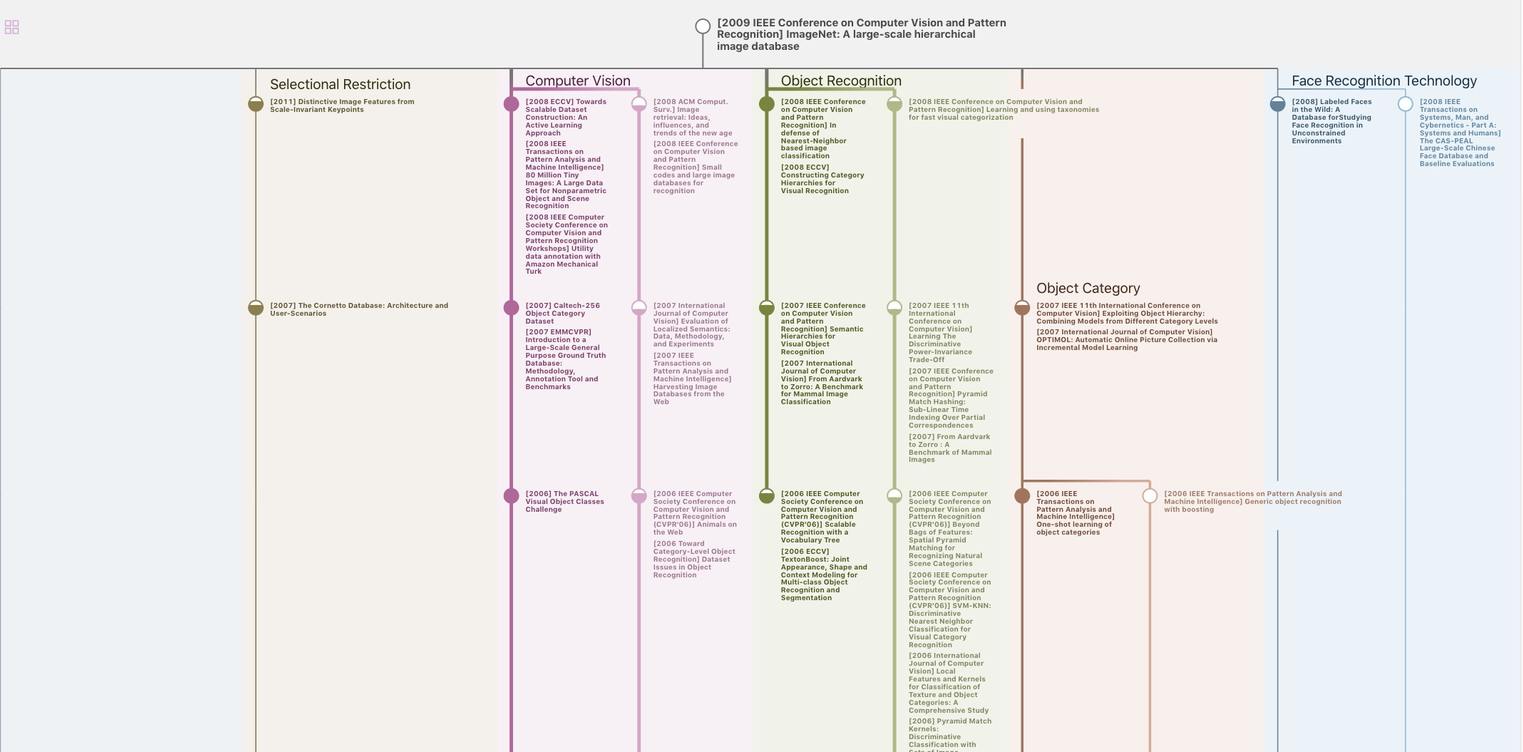
生成溯源树,研究论文发展脉络
Chat Paper
正在生成论文摘要