Doubly Sparse Factor Models For Unifying Feature Transformation And Feature Selection
INTERNATIONAL WORKSHOP ON STATISTICAL-MECHANICAL INFORMATICS 2010 (IW-SMI 2010)(2010)
摘要
A number of unsupervised learning methods for high-dimensional data are largely divided into two groups based on their procedures, i.e., (1) feature selection, which discards irrelevant dimensions of the data, and (2) feature transformation, which constructs new variables by transforming and mixing over all dimensions. We propose a method that both selects and transforms features in a common Bayesian inference procedure. Our method imposes a doubly automatic relevance determination (ARD) prior on the factor loading matrix. We propose a variational Bayesian inference for our model and demonstrate the performance of our method on both synthetic and real data.
更多查看译文
关键词
bayesian inference,feature selection,unsupervised learning,high dimensional data,factor model
AI 理解论文
溯源树
样例
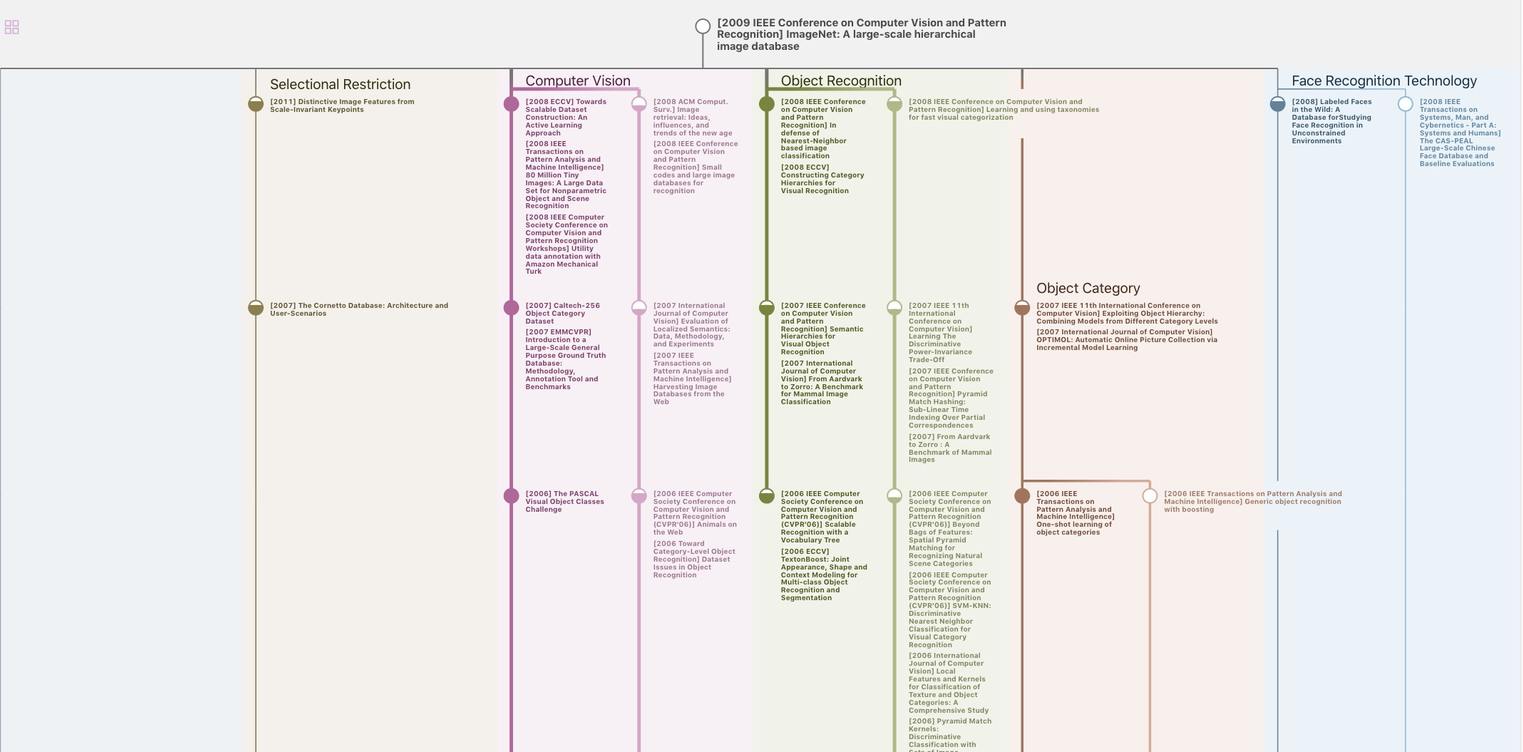
生成溯源树,研究论文发展脉络
Chat Paper
正在生成论文摘要