You've Got Answers: Towards Personalized Models for Predicting Success in Community Question Answering.
HLT-Short '08: Proceedings of the 46th Annual Meeting of the Association for Computational Linguistics on Human Language Technologies: Short Papers(2008)
摘要
Question answering communities such as Yahoo! Answers have emerged as a popular alternative to general-purpose web search. By directly interacting with other participants, information seekers can obtain specific answers to their questions. However, user success in obtaining satisfactory answers varies greatly. We hypothesize that satisfaction with the contributed answers is largely determined by the asker's prior experience, expectations, and personal preferences. Hence, we begin to develop personalized models of asker satisfaction to predict whether a particular question author will be satisfied with the answers contributed by the community participants. We formalize this problem, and explore a variety of content, structure, and interaction features for this task using standard machine learning techniques. Our experimental evaluation over thousands of real questions indicates that indeed it is beneficial to personalize satisfaction predictions when sufficient prior user history exists, significantly improving accuracy over a "one-size-fits-all" prediction model.
更多查看译文
关键词
asker satisfaction,satisfaction prediction,particular question author,prior experience,question answering community,real question,sufficient prior user history,user success,community participant,experimental evaluation,community question answering,personalized model
AI 理解论文
溯源树
样例
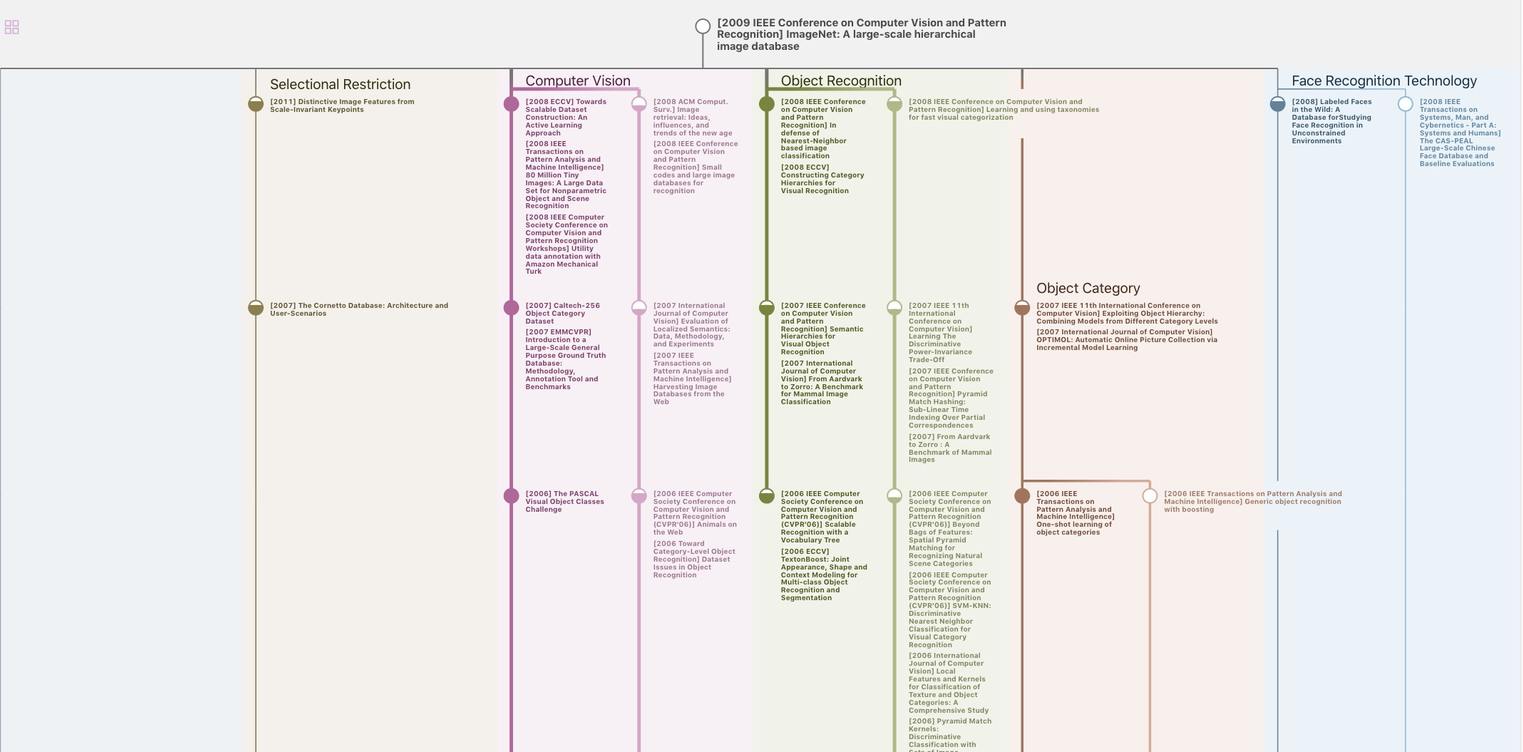
生成溯源树,研究论文发展脉络
Chat Paper
正在生成论文摘要