High Dimensional Correspondences From Low Dimensional Manifolds - An Empirical Comparison Of Graph-Based Dimensionality Reduction Algorithms
ACCV'10: Proceedings of the 2010 international conference on Computer vision - Volume part II(2011)
摘要
We discuss the utility of dimensionality reduction algorithms to put data points in high dimensional spaces into correspondence by learning a transformation between assigned data points on a lower dimensional structure. We assume that similar high dimensional feature spaces are characterized by a similar underlying low dimensional structure. To enable the determination of an affine transformation between two data sets we make use of well-known dimensional reduction algorithms. We demonstrate this procedure for applications like classification and assignments between two given data sets and evaluate six well-known algorithms during several experiments with different objectives. We show that with these algorithms and our transformation approach high dimensional data sets can be related to each other. We also show that linear methods turn out to be more suitable for assignment tasks, whereas graph-based methods appear to be superior for classification tasks.
更多查看译文
关键词
data set,high dimensional space,lower dimensional structure,similar high dimensional feature,similar underlying low dimensional,transformation approach high dimensional,well-known dimensional reduction algorithm,assigned data point,data point,affine transformation,high dimensional correspondence,low dimensional manifold,empirical comparison,graph-based dimensionality reduction algorithm
AI 理解论文
溯源树
样例
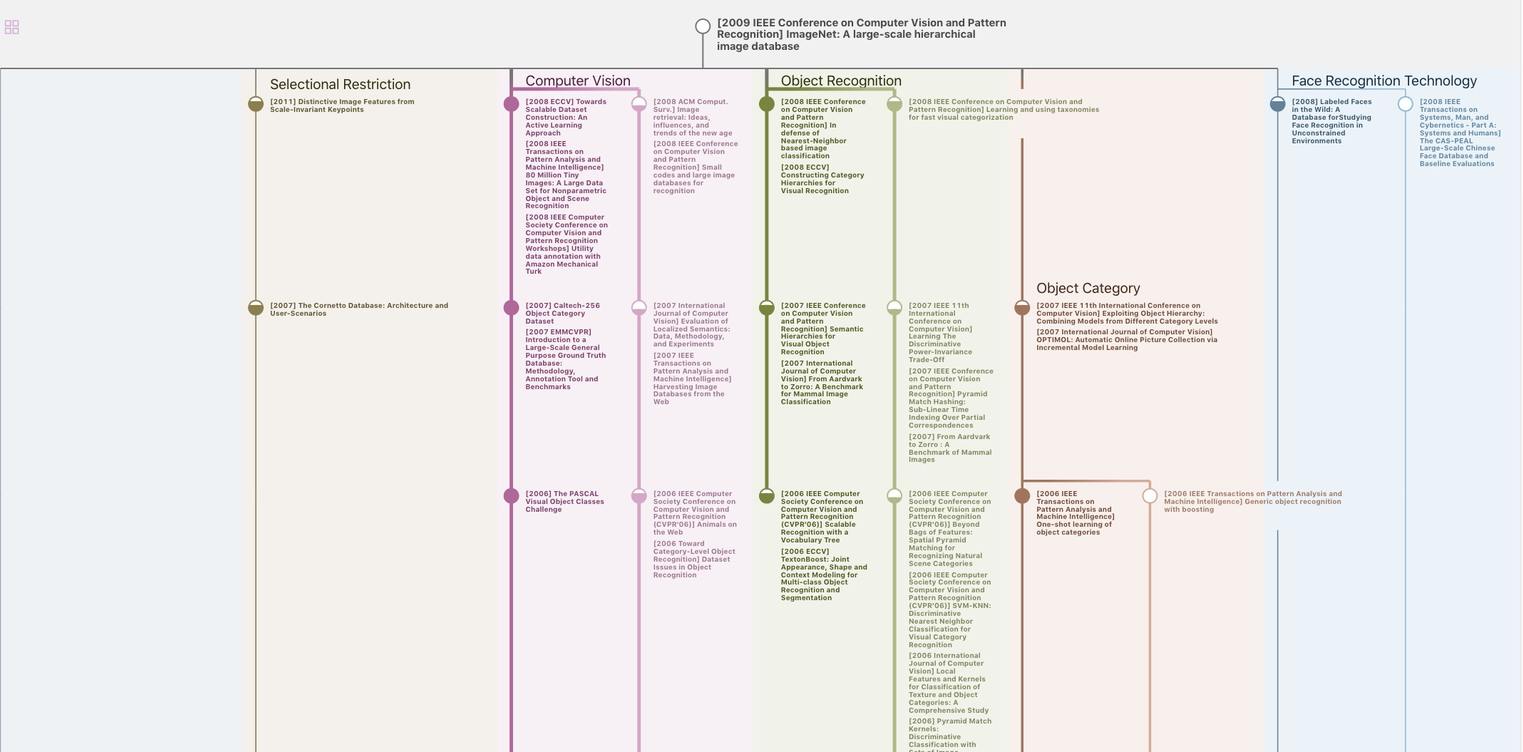
生成溯源树,研究论文发展脉络
Chat Paper
正在生成论文摘要