A Novel Multilevel Statistical Method for the Study of the Relationships Between Multireceptorial Binding Affinity Profiles and in Vivo Endpoints
Molecular Pharmacology(2010)
摘要
The present work introduces a novel method for drug research based on the sequential building of linked multivariate statistical models, each one introducing a different level of drug description. The use of multivariate methods allows us to overcome the traditional one-target assumption and to link in vivo endpoints with drug binding profiles, involving multiple receptors. The method starts with a set of drugs, for which in vivo or clinical observations and binding affinities for potentially relevant receptors are known, and allows obtaining predictions of the in vivo endpoints highlighting the most influential receptors. Moreover, provided that the structure of the receptor binding sites is known (experimentally or by homology modeling), the proposed method also highlights receptor regions and ligand-receptor interactions that are more likely to be linked to the in vivo endpoints, which is information of high interest for the design of novel compounds. The method is illustrated by a practical application dealing with the study of the metabolic side effects of antipsychotic drugs. Herein, the method detects related receptors confirmed by experimental results. Moreover, the use of structural models of the receptor binding sites allows identifying regions and ligand-receptor interactions that are involved in the discrimination between antipsychotic drugs that show metabolic side effects and those that do not. The structural results suggest that the topology of a hydrophobic sandwich involving residues in transmembrane helices (TM) 3, 5, and 6, as well as the assembling of polar residues in TM5, are important discriminators between target/antitarget receptors. Ultimately, this will provide useful information for the design of safer compounds inducing fewer side effects.
更多查看译文
关键词
Drug Target Identification,Network Pharmacology,Enantioselective Analysis
AI 理解论文
溯源树
样例
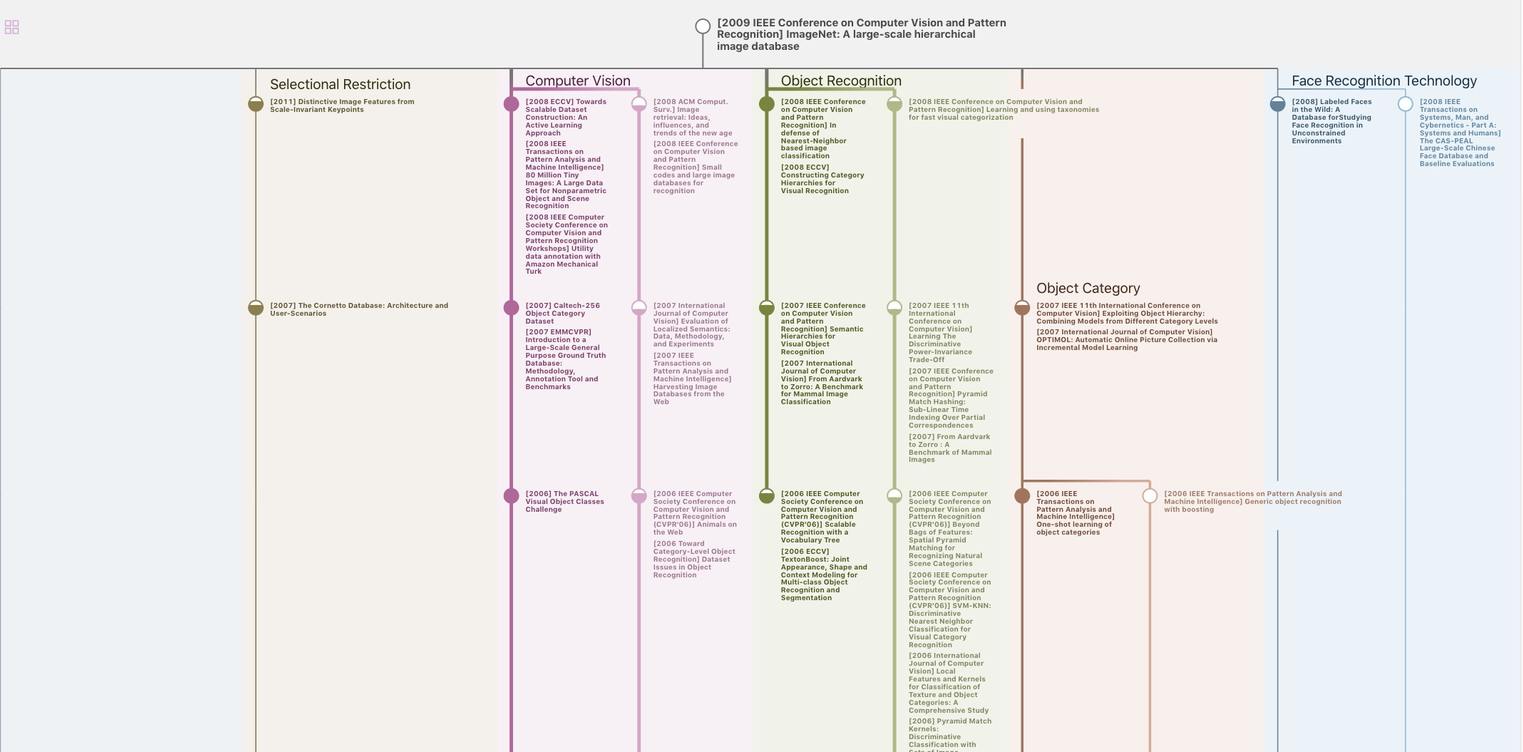
生成溯源树,研究论文发展脉络
Chat Paper
正在生成论文摘要