Application set approximation in optimal input design for model predictive control
Control Conference(2014)
摘要
This contribution considers one central aspect of experiment design in system identification, namely application set approximation. When a control design is based on an estimated model, the achievable performance is related to the quality of the estimate. The degradation in control performance due to plant-modeling missmatch is quantified by an application cost function. A convex approximation of the set of models that satisfy the control specification is typically required in optimal input design. The standard approach is to use a quadratic approximation of the application cost function, where the main computational effort is to find the corresponding Hessian matrix. Our main contribution is an alternative approach for this problem, which uses the structure of the underlying optimal control problem to considerably reduce the computations needed to find the application set. This technique allows the use of applications oriented input design for MPC on much more complex plants. The approach is numerically evaluated on a distillation control problem.
更多查看译文
关键词
Hessian matrices,approximation theory,control system synthesis,costing,distillation,identification,predictive control,Hessian matrix,MPC,application cost function,application set approximation,computational effort,control design,control specification,convex approximation,distillation control problem,experiment design,model predictive control,optimal control problem,optimal input design,plant-modeling missmatch,quadratic approximation,system identification
AI 理解论文
溯源树
样例
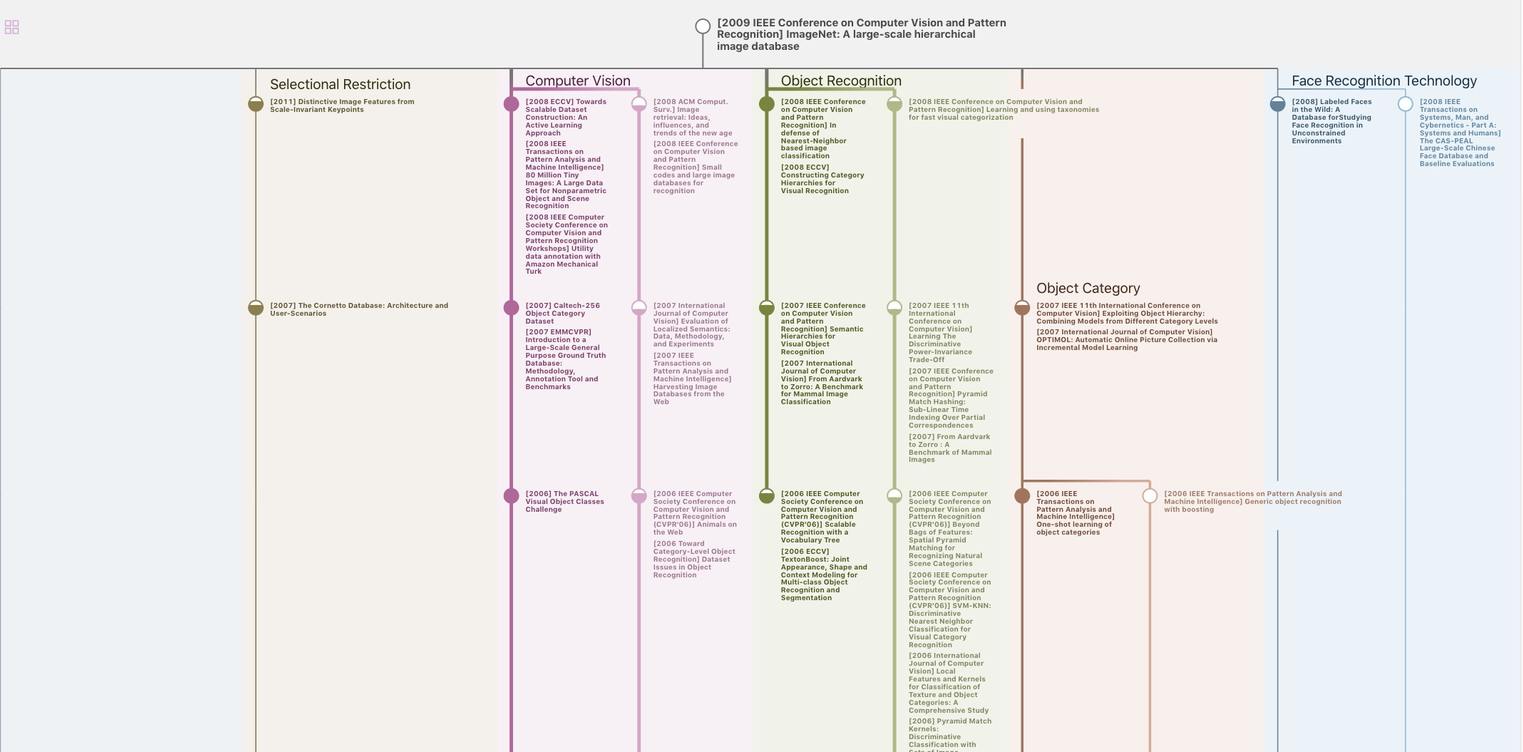
生成溯源树,研究论文发展脉络
Chat Paper
正在生成论文摘要