Bayesian nonparametric regression analysis of data with random effects covariates from longitudinal measurements.
BIOMETRICS(2011)
摘要
We consider nonparametric regression analysis in a generalized linear model (GLM) framework for data with covariates that are the subject-specific random effects of longitudinal measurements. The usual assumption that the effects of the longitudinal covariate processes are linear in the GLM may be unrealistic and if this happens it can cast doubt on the inference of observed covariate effects. Allowing the regression functions to be unknown, we propose to apply Bayesian nonparametric methods including cubic smoothing splines or P-splines for the possible nonlinearity and use an additive model in this complex setting. To improve computational efficiency, we propose the use of data-augmentation schemes. The approach allows flexible covariance structures for the random effects and within-subject measurement errors of the longitudinal processes. The posterior model space is explored through a Markov chain Monte Carlo (MCMC) sampler. The proposed methods are illustrated and compared to other approaches, the "naive" approach and the regression calibration, via simulations and by an application that investigates the relationship between obesity in adulthood and childhood growth curves.
更多查看译文
关键词
Bayesian nonparametric regression,Data augmentation,Generalized additive model,Longitudinal data,Measurement error
AI 理解论文
溯源树
样例
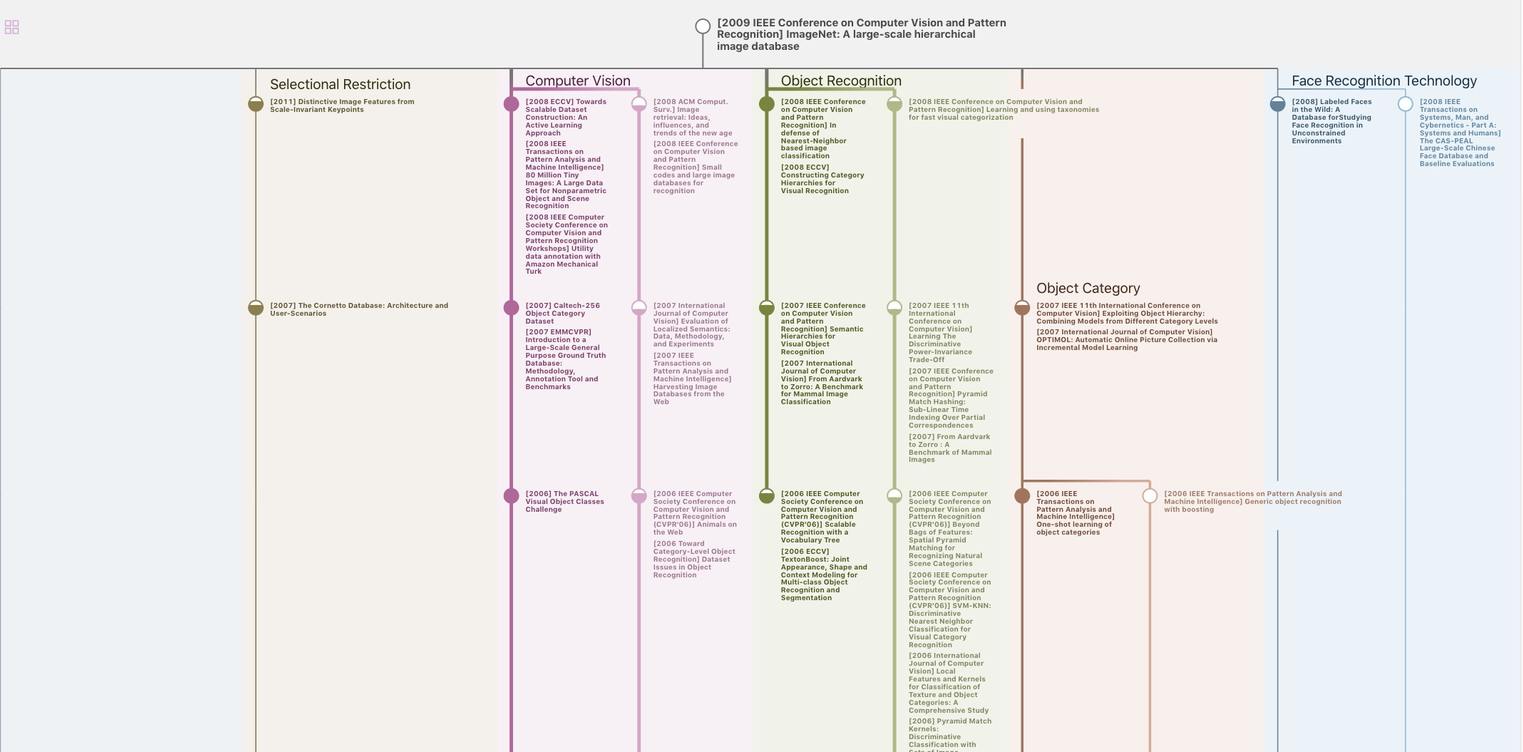
生成溯源树,研究论文发展脉络
Chat Paper
正在生成论文摘要