Sampling-Based Relative Landmarks: Systematically Test-Driving Algorithms Before Choosing.
EPIA '01: Proceedings of the10th Portuguese Conference on Artificial Intelligence on Progress in Artificial Intelligence, Knowledge Extraction, Multi-agent Systems, Logic Programming and Constraint Solving(2001)
摘要
When facing the need to select the most appropriate algorithm to apply on a new data set, data analysts often follow an approach which can be related to test-driving cars to decide which one to buy: apply the algorithms on a sample of the data to quickly obtain rough estimates of their performance. These estimates are used to select one or a few of those algorithms to be tried out on the full data set. We describe sampling-based landmarks (SL), a systematization of this approach, building on earlier work on landmarking and sampling. SL are estimates of the performance of algorithms on a small sample of the data that are used as predictors of the performance of those algorithms on the full set. We also describe relative landmarks (RL), that address the inability of earlier landmarks to assess relative performance of algorithms. RL aggregate landmarks to obtain predictors of relative performance. Our experiments indicate that the combination of these two improvements, which we call Sampling-based Relative Landmarks, are better for ranking than traditional data characterization measures.
更多查看译文
关键词
relative performance,data analyst,full data,new data,traditional data characterization measure,full set,relative landmark,RL aggregate landmark,earlier landmark,earlier work,Sampling-Based Relative Landmarks,Systematically Test-Driving Algorithms
AI 理解论文
溯源树
样例
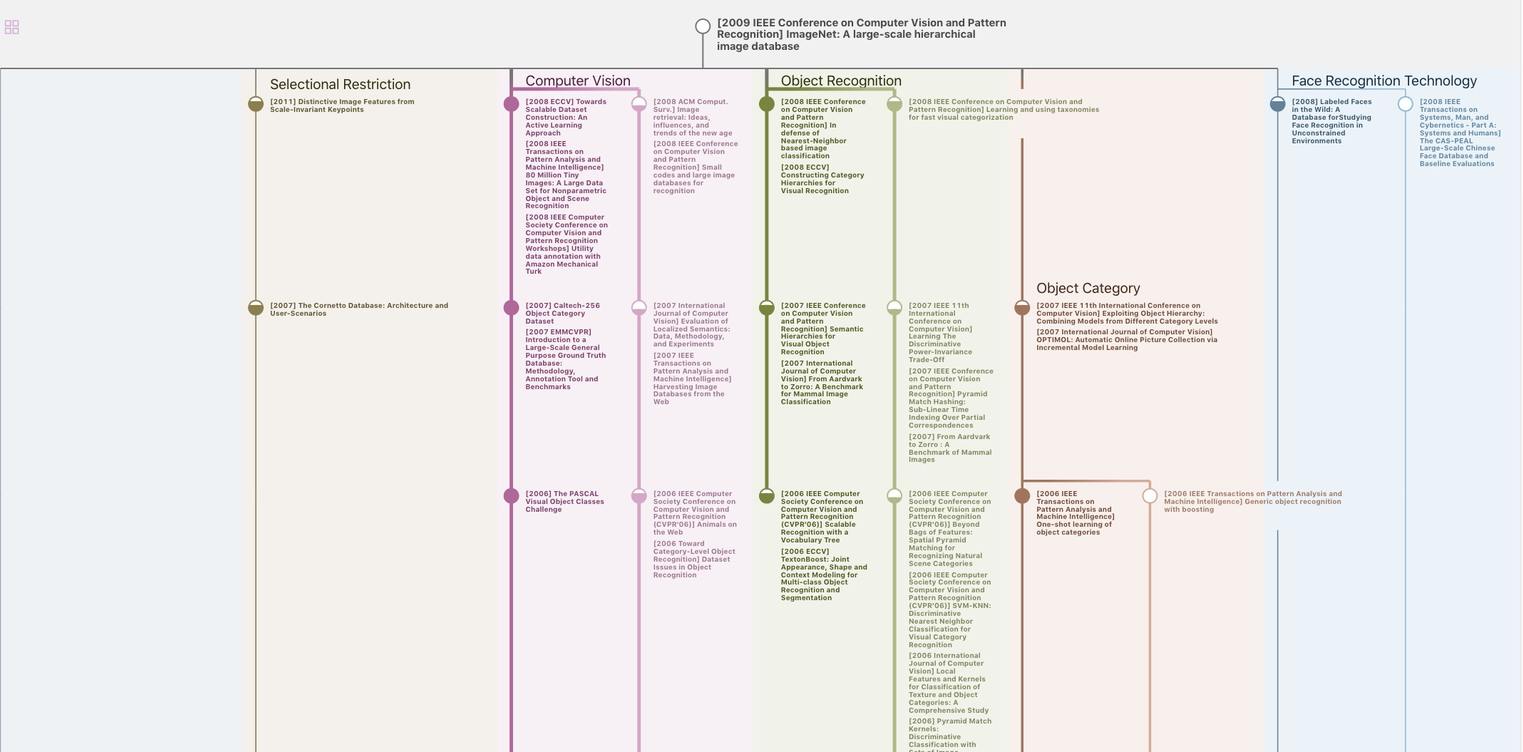
生成溯源树,研究论文发展脉络
Chat Paper
正在生成论文摘要