Constructing Low Star Discrepancy Point Sets with Genetic Algorithms
GECCO '13: Genetic and Evolutionary Computation Conference Amsterdam The Netherlands July, 2013(2013)
摘要
Geometric discrepancies are standard measures to quantify the irregularity of distributions. They are an important notion in numerical integration. One of the most important discrepancy notions is the so-called \emph{star discrepancy}. Roughly speaking, a point set of low star discrepancy value allows for a small approximation error in quasi-Monte Carlo integration. It is thus the most studied discrepancy notion. In this work we present a new algorithm to compute point sets of low star discrepancy. The two components of the algorithm (for the optimization and the evaluation, respectively) are based on evolutionary principles. Our algorithm clearly outperforms existing approaches. To the best of our knowledge, it is also the first algorithm which can be adapted easily to optimize inverse star discrepancies.
更多查看译文
关键词
Geometric discrepancy,Monte-Carlo methods,information-based complexity,search heuristics,genetic algorithms,algorithm engineering
AI 理解论文
溯源树
样例
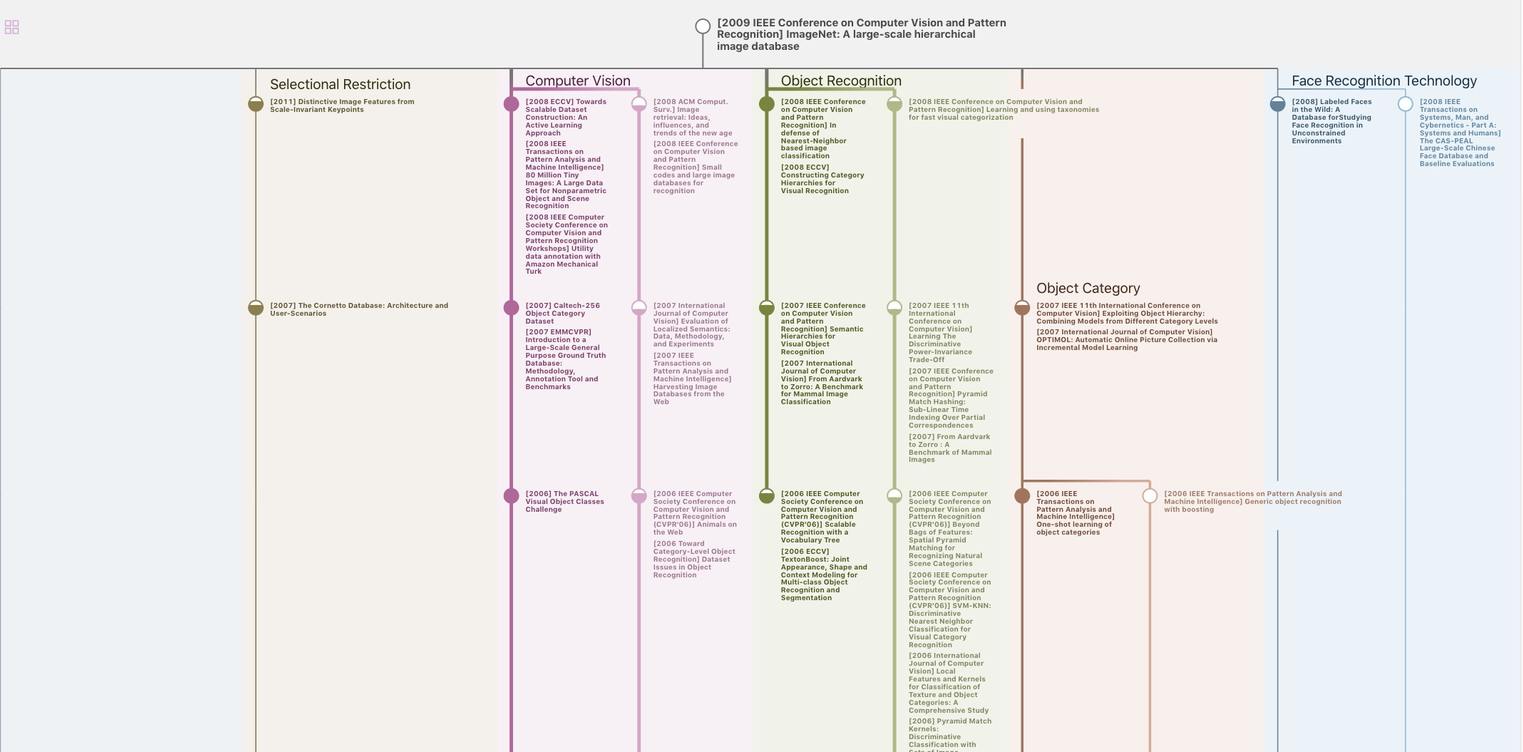
生成溯源树,研究论文发展脉络
Chat Paper
正在生成论文摘要