Stochastic Algorithm Computational Complexity Comparison on Test Functions
APPLIED SOFT COMPUTING TECHNOLOGIES: THE CHALLENGE OF COMPLEXITY(2006)
摘要
The Evolutionary Algorithms(EA), see [1] and [2], are stochastic techniques able to find the optimal solution to a given problem. The concept of optimal solution depends on the specific application, it could be the search of the global minimum of a complicated function. These algorithms
are based on Darwin theories about natural selection. Natural selection allows to survive only best individuals (that is individuals more suitable to fit environment changes);
in this way there is a generalized improvement of the entire population. Only the most performing individuals can transfer
their genotype to the descendants.In the EA the parameter measuring individuals performance (in literature known as individuals
fitness) is called fitness function. Time goes on by discrete steps. Starting by an initial population randomly generated, the process of evolution takes place.
The most used operators that allow to obtain the new generation are: Reproduction, Recombination, Mutation and Selection. Let’s to consider more formally these statements. Given a generic fitness function F defined in a N-dimensional parameters space, Y, and with values in an M-dimensional space Z:
更多查看译文
关键词
evolutionary algorithms,evolution strategies,differential evolution,particle swarm optimization
AI 理解论文
溯源树
样例
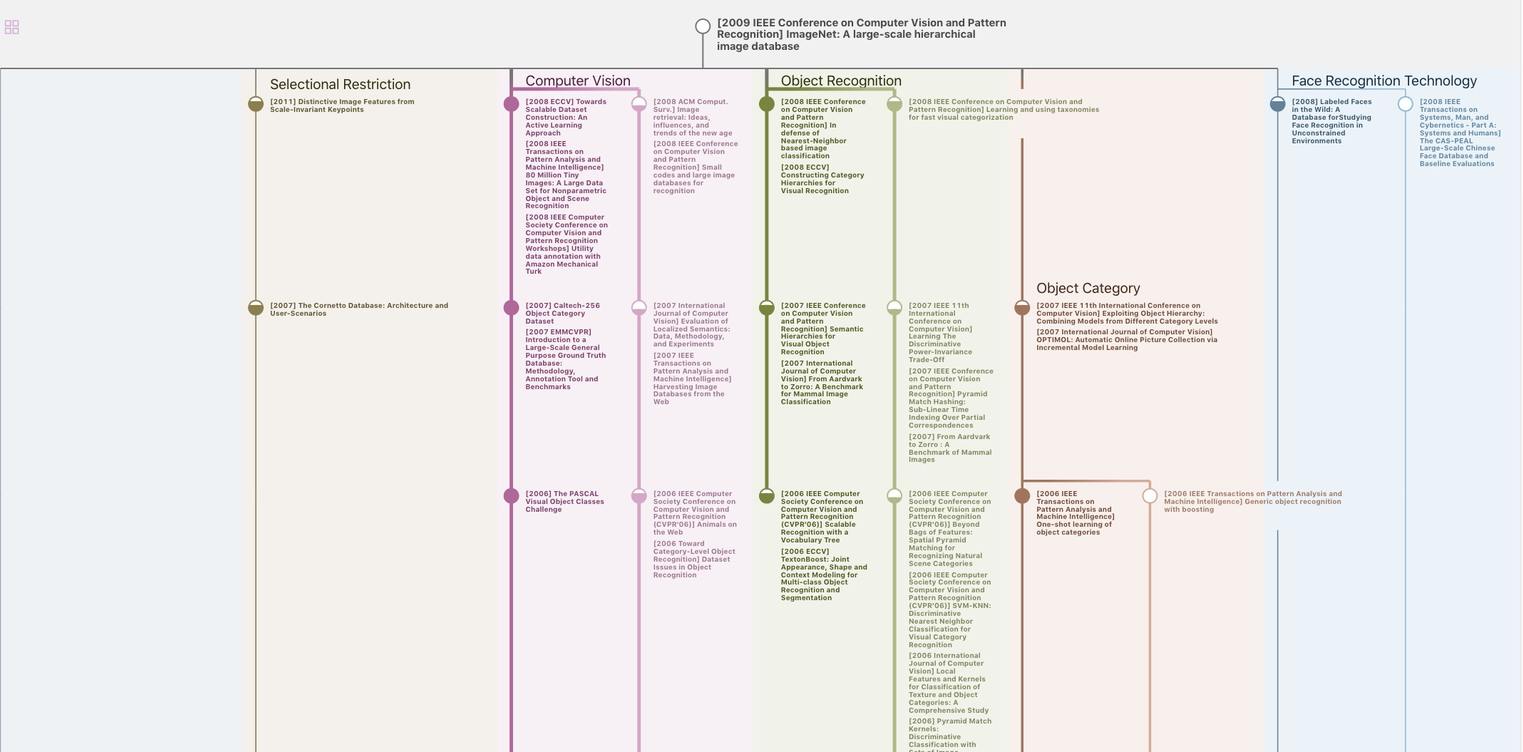
生成溯源树,研究论文发展脉络
Chat Paper
正在生成论文摘要