Application of Kernel C-Means Clustering and Dempster-Shafer Theory of evidence in power transformers fault diagnosis
FSKD(2010)
摘要
Transformers fault diagnosis plays a vital role in running security and reliability. The detected information is collected from the disperse sensor, which is lack of the data fusion analysis and easily lead to decision error and leak. A model of oil-immersed transformer fault diagnosis based on the collaborative method of Kernel C-Means Clustering (KCM) and multi-source information data fusion is presented. The basic idea is that the trained samples are clustered first by using KCM, and then the Dempster-Shafer theory of evidence Fusion method is used to train the chosed sample, and decide the transformer fault. The result of the method shows that the above method can reduce the uncertainty efficiently and have a good performance of transformer fault diagnosis.
更多查看译文
关键词
transformer oil,pattern clustering,dempster-shafer theory of evidence,power transformers fault diagnosis,oil-immersed transformer fault diagnosis,data fusion,fault diagnosis,kernel c-means clustering,d-s evidential theory,uncertainty handling,reliability,power transformer insulation,multi-source information data fusion,power transformers,power transformer,uncertainty,mathematical model,kernel
AI 理解论文
溯源树
样例
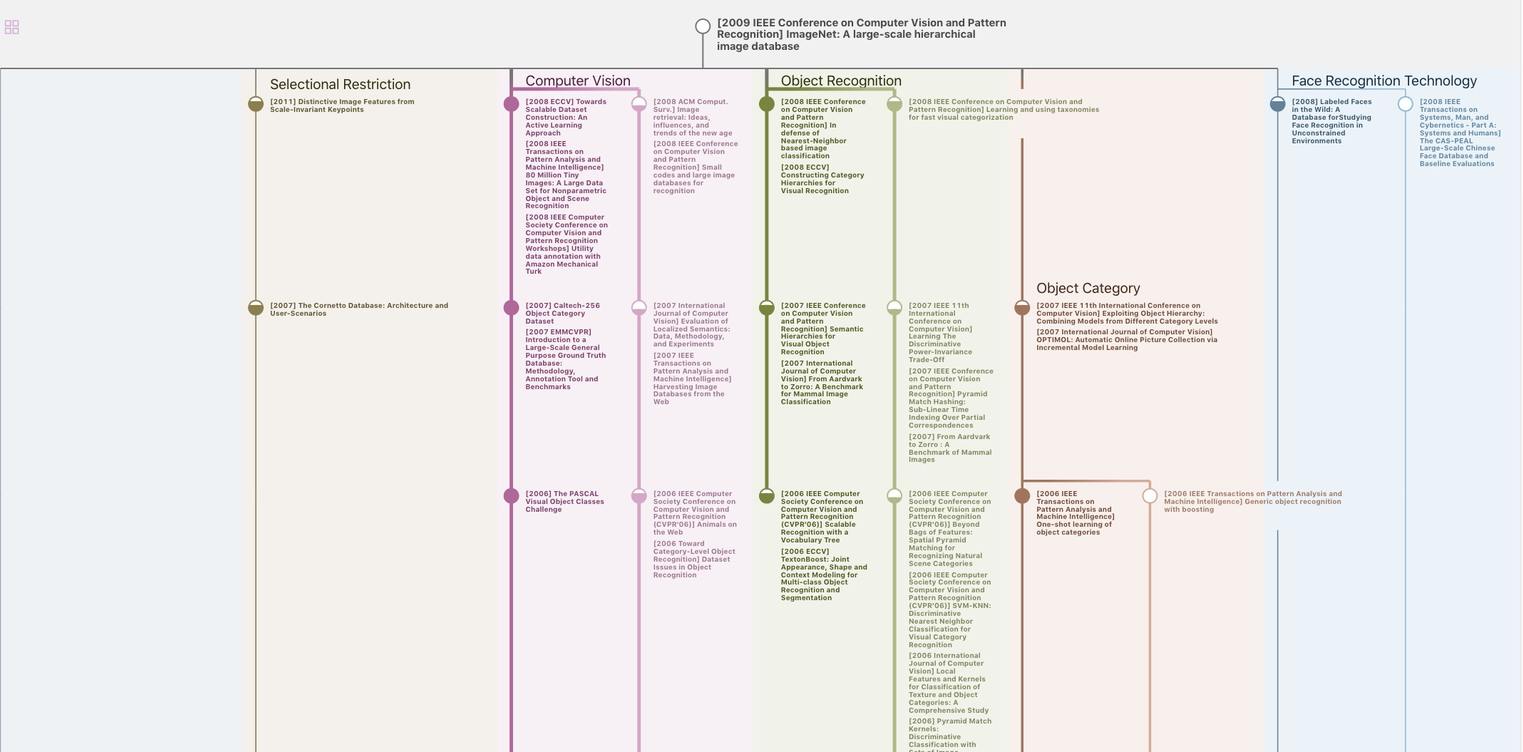
生成溯源树,研究论文发展脉络
Chat Paper
正在生成论文摘要