Information theoretic feature space slicing for statistical anomaly detection.
Journal of Network and Computer Applications(2014)
摘要
Anomaly detection accuracy has been a serious limitation in commercial ADS deployments. A main reason for this limitation is the expectation that an ADS should achieve very high accuracy while having extremely low computational complexity. The constraint of low computational cost has recently been relaxed with the emergence of cheap high-performance platforms (e.g., multi-core, GPU, SCC, etc.). Moreover, current ADSs perform anomaly detection on aggregate feature spaces, with large volumes of benign and close-to-benign feature instances that overwhelm the feature space and hence yield low accuracies. In this paper, we ask and address the following question: Can the accuracy of an ADS be improved if we slice ADS feature space at the cost of higher computational resource utilization? We first observe that existing ADSs are not designed to exploit better computational platforms to achieve higher accuracies. To mitigate this problem, we identify the fundamental accuracy limiting factors for statistical network and host-based ADSs. We then show that these bottlenecks can be alleviated by our proposed feature space slicing framework. Our framework slices a statistical ADS׳ feature space into multiple disjoint subspaces and then performs anomaly detection separately on each subspace by utilizing more computational resources. We propose generic information-theoretic methods for feature space slicing and for determining the appropriate number of subspaces for any statistical ADS. Performance evaluation on three independently-collected attack datasets and multiple ID algorithms shows that the enhanced ADSs are able to achieve dramatic improvements in detection (up to 75%) and false alarm (up to 99%) rates.
更多查看译文
关键词
Feature slicing,Conditional entropy,Information content,Clustering,Statistical anomaly detection
AI 理解论文
溯源树
样例
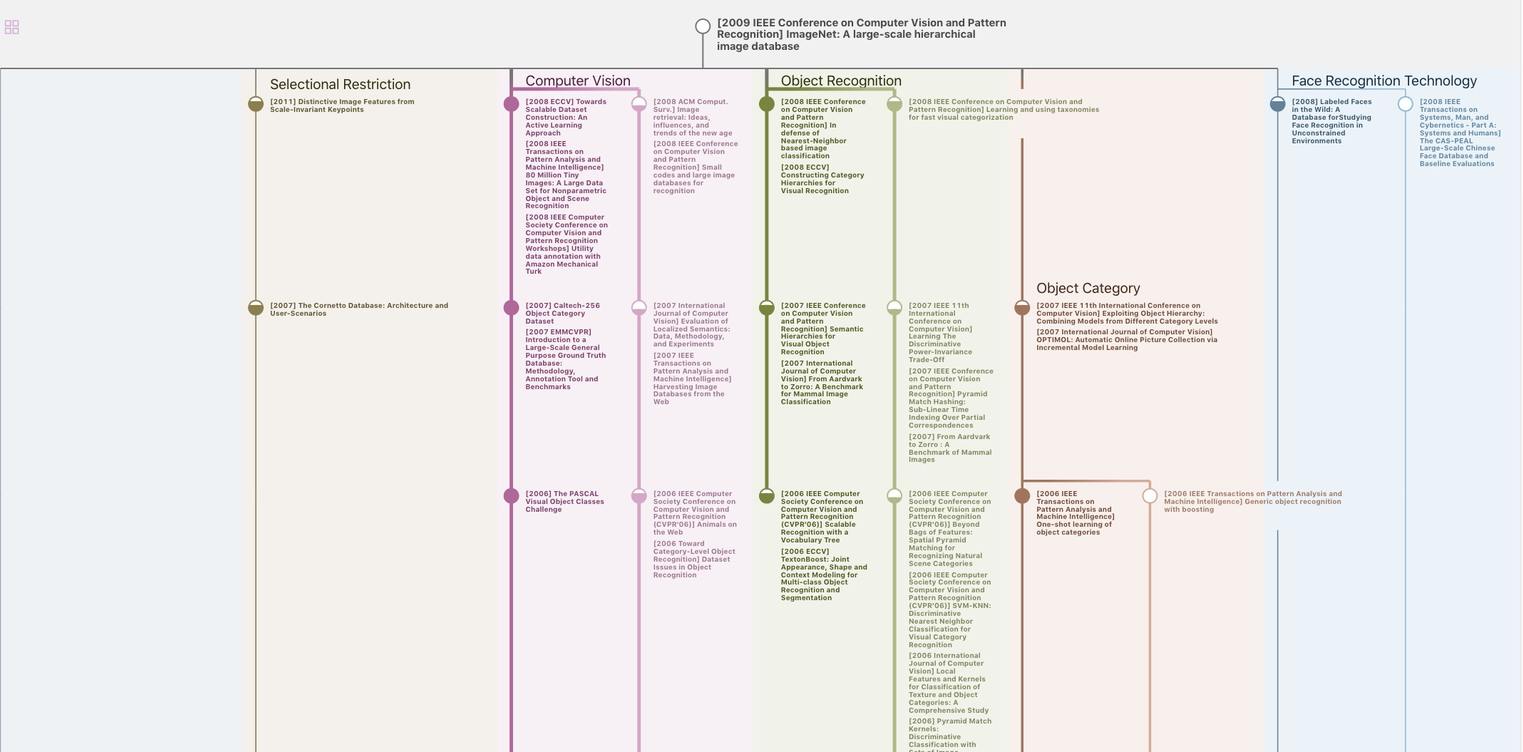
生成溯源树,研究论文发展脉络
Chat Paper
正在生成论文摘要