Transferring Visual Prior for Online Object Tracking
IEEE Transactions on Image Processing(2012)
摘要
Visual prior from generic real-world images can be learned and transferred for representing objects in a scene. Motivated by this, we propose an algorithm that transfers visual prior learned offline for online object tracking. From a collection of real-world images, we learn an overcomplete dictionary to represent visual prior. The prior knowledge of objects is generic, and the training image set does not necessarily contain any observation of the target object. During the tracking process, the learned visual prior is transferred to construct an object representation by sparse coding and multiscale max pooling. With this representation, a linear classifier is learned online to distinguish the target from the background and to account for the target and background appearance variations over time. Tracking is then carried out within a Bayesian inference framework, in which the learned classifier is used to construct the observation model and a particle filter is used to estimate the tracking result sequentially. Experiments on a variety of challenging sequences with comparisons to several state-of-the-art methods demonstrate that more robust object tracking can be achieved by transferring visual prior.
更多查看译文
关键词
particle filter,principal component analysis,image reconstruction,object recognition,dictionaries,bayesian inference,transfer learning,sparse coding,vectors,linear classifier,image classification,visualization,object tracking
AI 理解论文
溯源树
样例
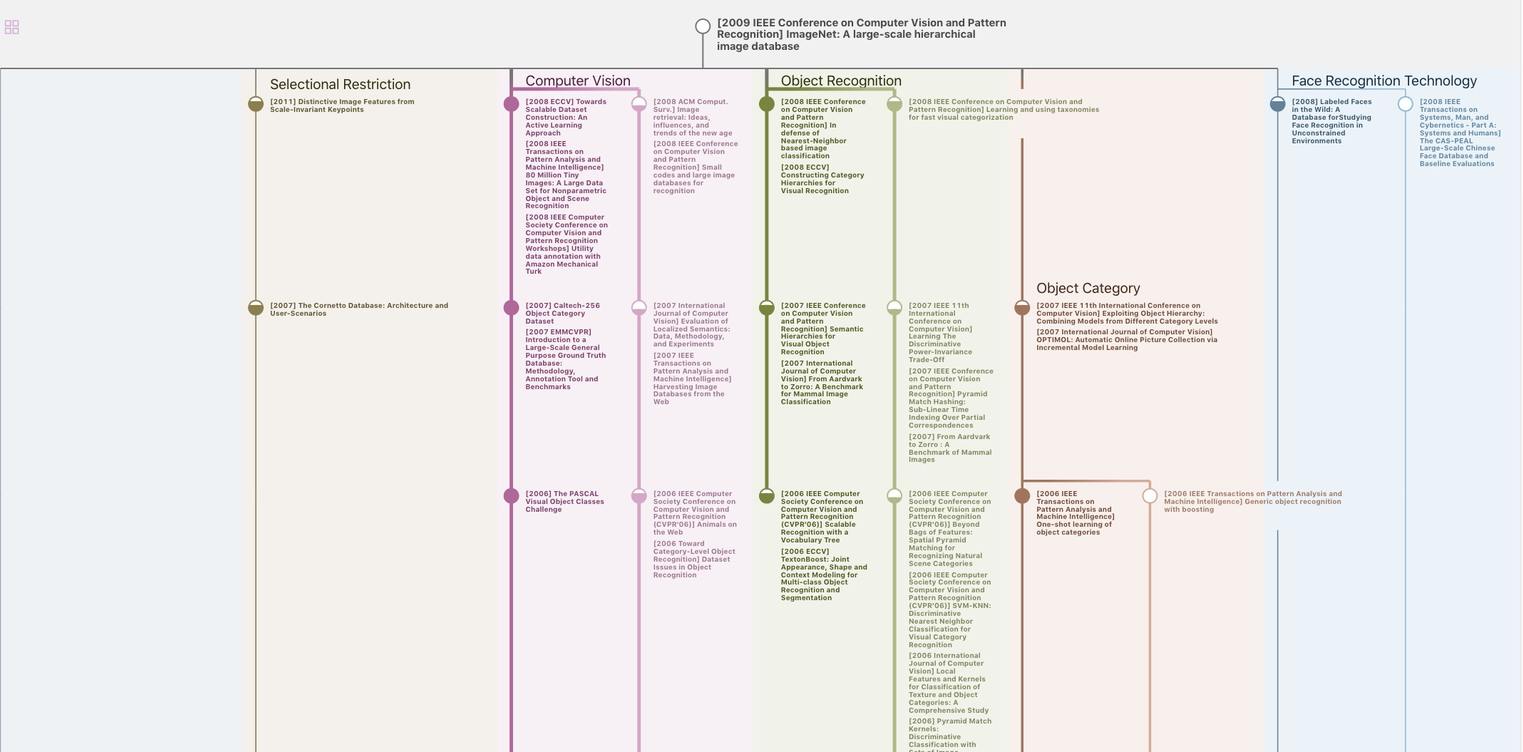
生成溯源树,研究论文发展脉络
Chat Paper
正在生成论文摘要