Design, analysis and comparison of robot learners
ROBOTICS AND AUTONOMOUS SYSTEMS(1998)
摘要
This paper outlines some ideas as to how robot learning experiments might best be designed. There are three principal findings: (i) in order to evaluate robot learners we must employ multiple evaluation methods together; (ii) in order to measure in any absolute way the performance of a learning algorithm we must characterise the complexity of the underlying decision task formed by the interaction of the agent, task and environment; (iii) that in fact this goal is too difficult to attain in practice, and progress in robot learning must rely on comparative work. Four methods for agent analysis are presented. These are used to analyse a robot that learns to push boxes from reinforcement. Using these techniques we have been able to show that Q(lambda)-learning outperforms one-step Q-learning on a typical robot task. The differences are statistically significant. We emphasise the importance of experimental design in order to integrate the various forms of evaluation. (C) 1998 Elsevier Science B.V. All right reserved.
更多查看译文
关键词
robot learning,reinforcement learning,Q-learning,experimental methodology
AI 理解论文
溯源树
样例
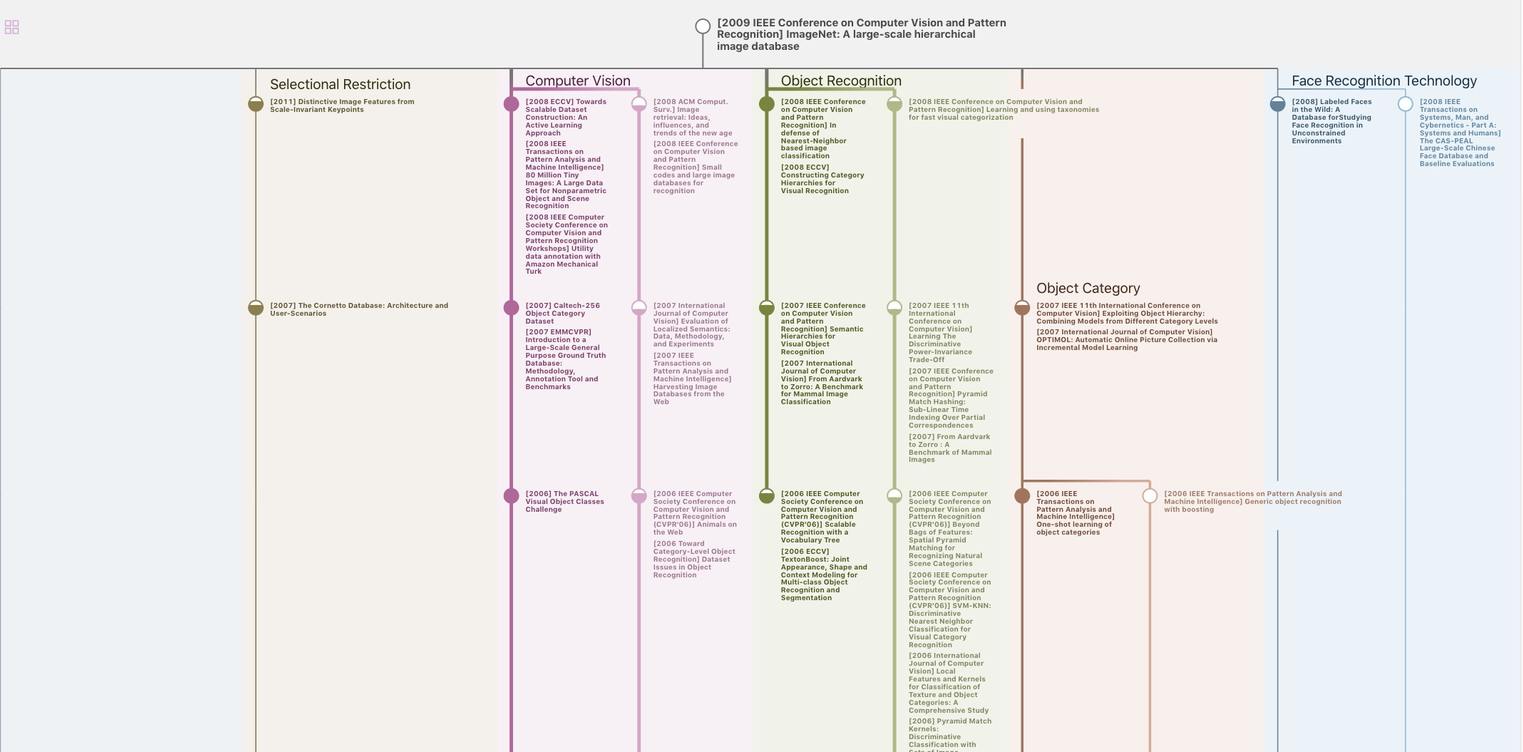
生成溯源树,研究论文发展脉络
Chat Paper
正在生成论文摘要