Generating Linked Data by Inferring the Semantics of Tables
VLDS(2011)
摘要
Vast amounts of information is encoded in structured ta- bles found in documents, on the Web, and in spreadsheets or databases. Integrating or searching over this information benets from understanding its intended meaning. Evidence for a table's meaning can be found in its column headers, cell values, implicit relations between columns, caption and sur- rounding text but also requires general and domain-specic background knowledge. We represent a table's meaning by mapping columns to classes in an appropriate ontology, link- ing cell values to literal constants, implied measurements, or entities in the linked data cloud (existing or new) and dis- covering or and identifying relations between columns. We describe techniques grounded in graphical models and prob- abilistic reasoning to infer meaning (semantics) associated with a table. Using background knowledge from the Linked Open Data cloud, we jointly infer the semantics of column headers, table cell values (e.g.,strings and numbers) and re- lations between columns and represent the inferred meaning as graph of RDF triples. We motivate the value of this approach using tables from the medical domain, discussing some of the challenges presented by these tables and describ- ing techniques to tackle them.
更多查看译文
关键词
linked data,linked open data,graphical model,database integration,information
AI 理解论文
溯源树
样例
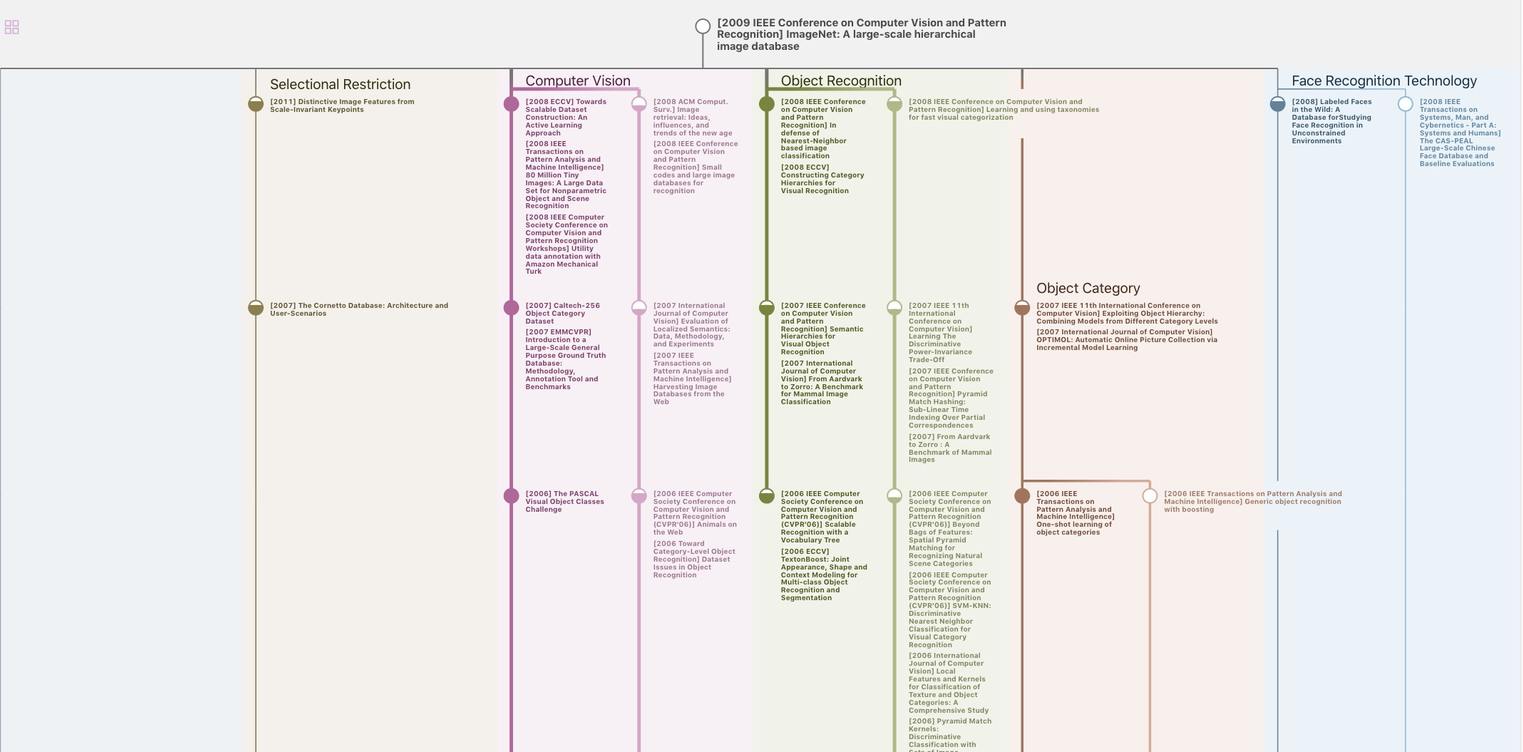
生成溯源树,研究论文发展脉络
Chat Paper
正在生成论文摘要