Pac-Bayes Bounds with Data Dependent Priors
Journal of machine learning research(2012)
摘要
This paper presents the prior PAC-Bayes bound and explores its capabilities as a tool to provide tight predictions of SVMs' generalization. The computation of the bound involves estimating a prior of the distribution of classifiers from the available data, and then manipulating this prior in the usual PAC-Bayes generalization bound. We explore two alternatives: to learn the prior from a separate data set, or to consider an expectation prior that does not need this separate data set. The prior PAC-Bayes bound motivates two SVM-like classification algorithms, prior SVM and ν-prior SVM, whose regularization term pushes towards the minimization of the prior PAC-Bayes bound. The experimental work illustrates that the new bounds can be significantly tighter than the original PAC-Bayes bound when applied to SVMs, and among them the combination of the prior PAC-Bayes bound and the prior SVM algorithm gives the tightest bound.
更多查看译文
关键词
PAC-Bayes bound,support vector machine,generalization capability prediction,classification
AI 理解论文
溯源树
样例
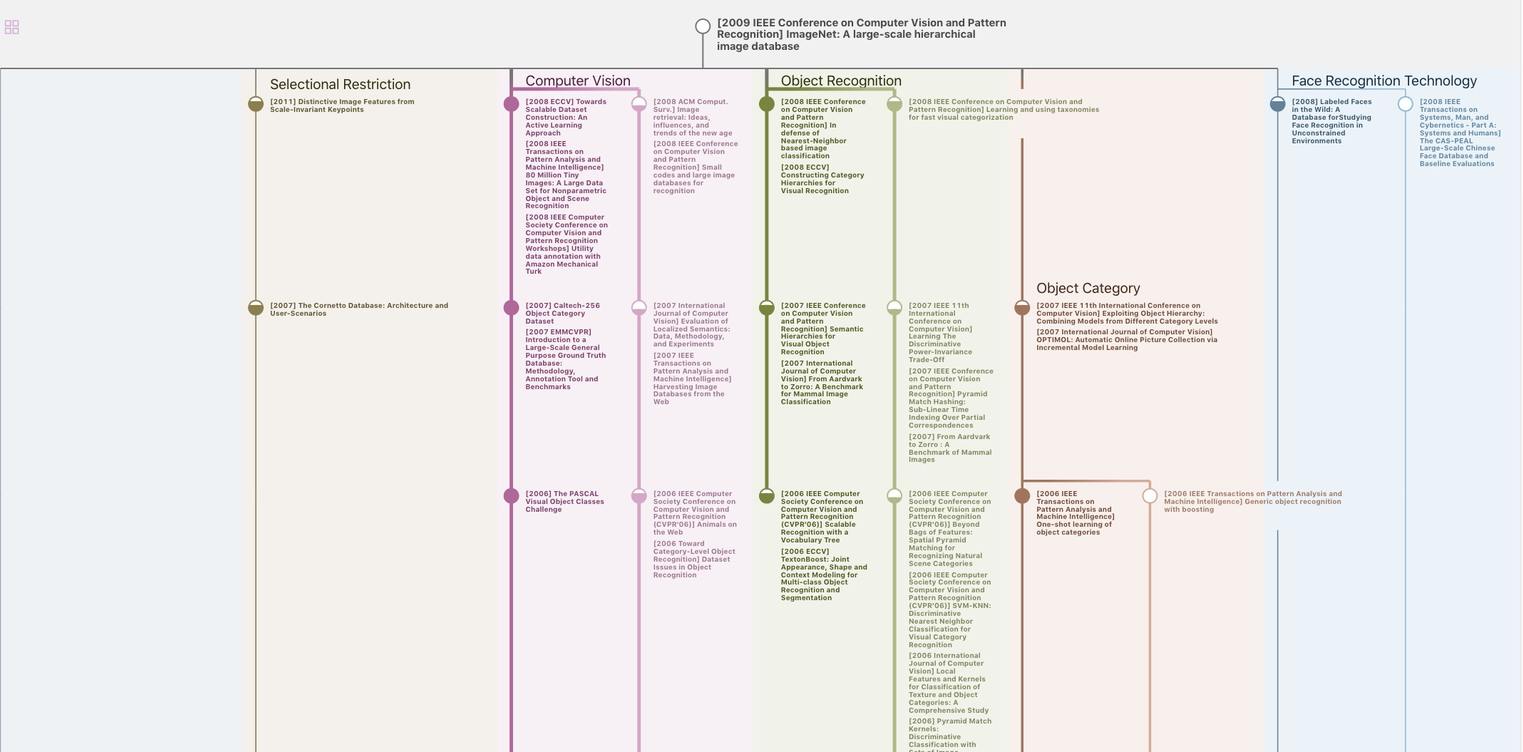
生成溯源树,研究论文发展脉络
Chat Paper
正在生成论文摘要