Highly Efficient Optimal K-Anonymity For Biomedical Datasets
2012 25TH INTERNATIONAL SYMPOSIUM ON COMPUTER-BASED MEDICAL SYSTEMS (CBMS)(2012)
摘要
K-anonymization is a wide-spread technique for the de-identification of biomedical datasets. To not render the data useless for further analysis it is often important to find an optimal solution to the k-anonymity problem, i.e., a transformation with minimum information loss. As performance is often a key requirement this paper describes an efficient implementation of a k-anonymization algorithm which is especially suitable for biomedical datasets. Although our basic implementation already offers excellent performance we present several further optimizations and show that these yield an additional speedup of up to a factor of five even for large datasets.
更多查看译文
关键词
optimization,databases,data analysis,lattices,measurement
AI 理解论文
溯源树
样例
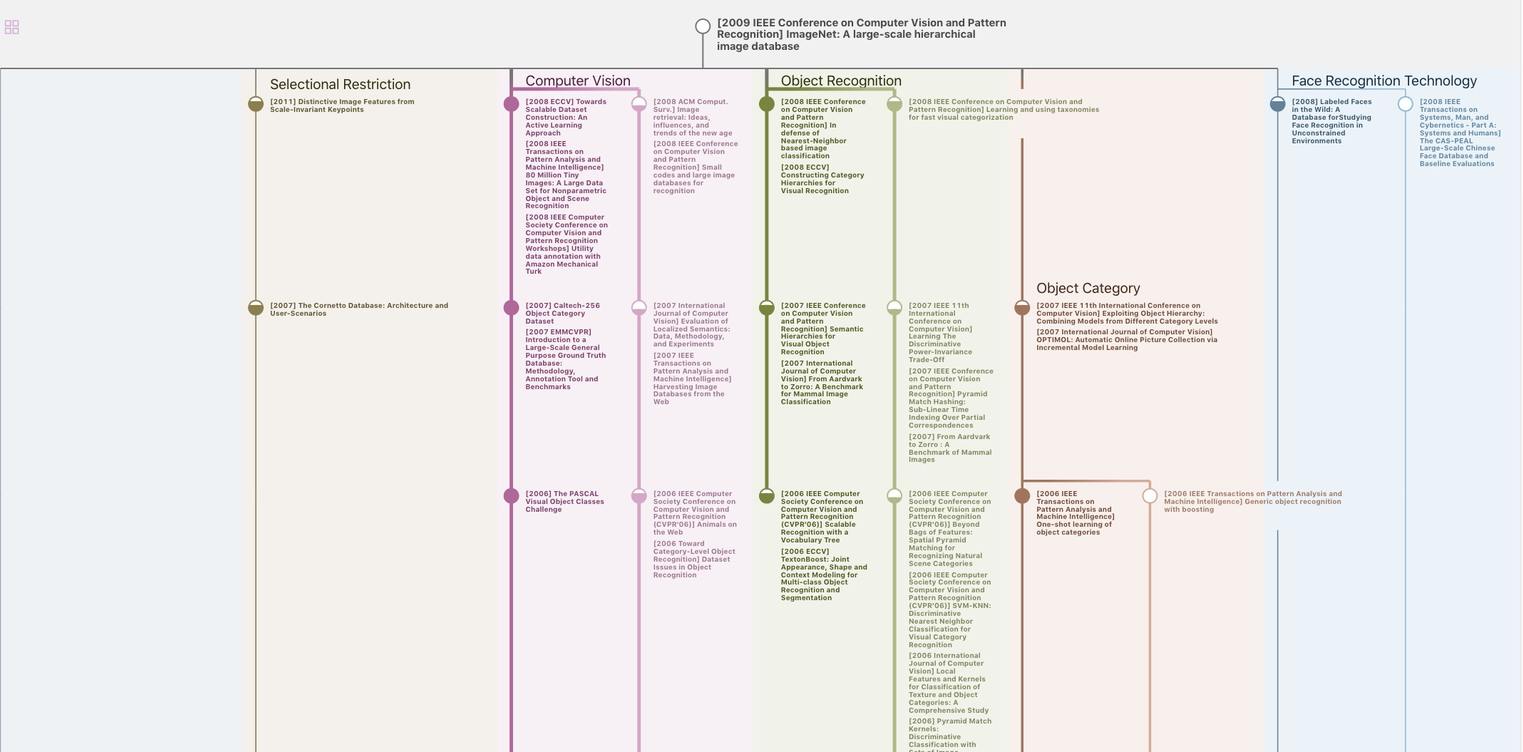
生成溯源树,研究论文发展脉络
Chat Paper
正在生成论文摘要