Improvements Of A Dual-Input Dbn For Noise Robust Asr
12TH ANNUAL CONFERENCE OF THE INTERNATIONAL SPEECH COMMUNICATION ASSOCIATION 2011 (INTERSPEECH 2011), VOLS 1-5(2011)
摘要
In previous work we have shown that an ASR system consisting of a dual-input Dynamic Bayesian Network (DBN) which simultaneously observes MFCC acoustic features and an exemplar-based Sparse Classification (SC) phoneme predictor stream can achieve better word recognition accuracies in noise than a system that observes only one input stream. This paper explores three modifications of SC input to further improve the noise robustness of the dual-input DBN system: 1) using state likelihoods instead of phonemes, 2) integrating more contextual information and 3) using a complete set of likelihood distribution. Experiments on AURORA-2 reveal that the combination of the first two approaches significantly improves the recognition results, achieving up to 29% (absolute) accuracy gain at SNR -5 dB. In the dual-input system using the full likelihood vector does not outperform using the best state prediction.
更多查看译文
关键词
ASR, noise robustness, sparse classification, dual-input DBN
AI 理解论文
溯源树
样例
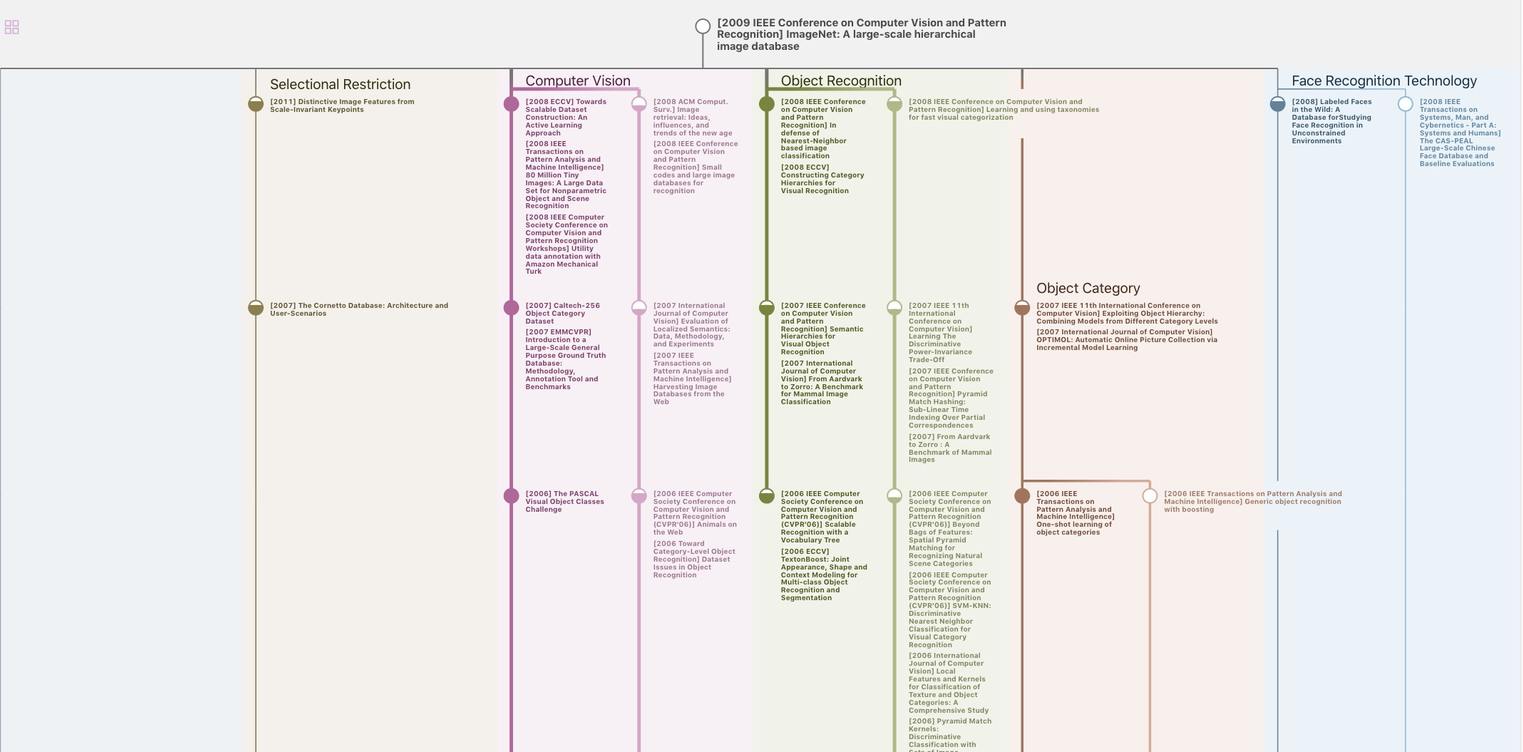
生成溯源树,研究论文发展脉络
Chat Paper
正在生成论文摘要