Faster statistical cell characterization using adjoint sensitivity analysis
CICC(2008)
摘要
With the adoption of statistical static timing analysis (SSTA), the characterization of standard cell libraries for delay variations and output transition time (output slew) variations, referred to as statistical characterization, is becoming essential. Statistical characterization of intra-cell mismatch variations as well as inter-chip variations need to be performed efficiently with acceptable accuracy as a function of process parameter variations. The conventional approach to this problem is to model these mismatch variations by characterizing each device variation separately. However, this entails a cost that is proportional to the product of the number of devices (nd) in the cell and the number of local statistical parameters (np), and characterization becomes infeasible. In this work, we propose an improved transient sensitivity analysis to accelerate statistical characterization. We compute sensitivities of node voltages with respect to any process/design parameters. These sensitivities are used to extract the sensitivities of delays and transition times. It is more critical to note the sparsity of the circuitpsilas dependence on the statistical parameters (i.e., any given parameter directly impacts only a small portion of the circuit, sometimes only one device). By exploiting this sparsity we obtain a method that is O(np), compared to O(nptimesnd ) of the conventional approach. As an example, for an AOI cell with 40 devices, the sensitivity analysis, compared to the standard approach using multiple simulations, results in more than 18X runtime improvements with better accuracy.
更多查看译文
关键词
standard cell libraries,statistical static timing analysis,statistical analysis,statistical cell characterization,delays,transient sensitivity analysis,local statistical parameters,intracell mismatch variations,integrated circuit design,delay variations,adjoint sensitivity analysis,chip,process design,sensitivity,sensitivity analysis,computational modeling,algorithm design and analysis
AI 理解论文
溯源树
样例
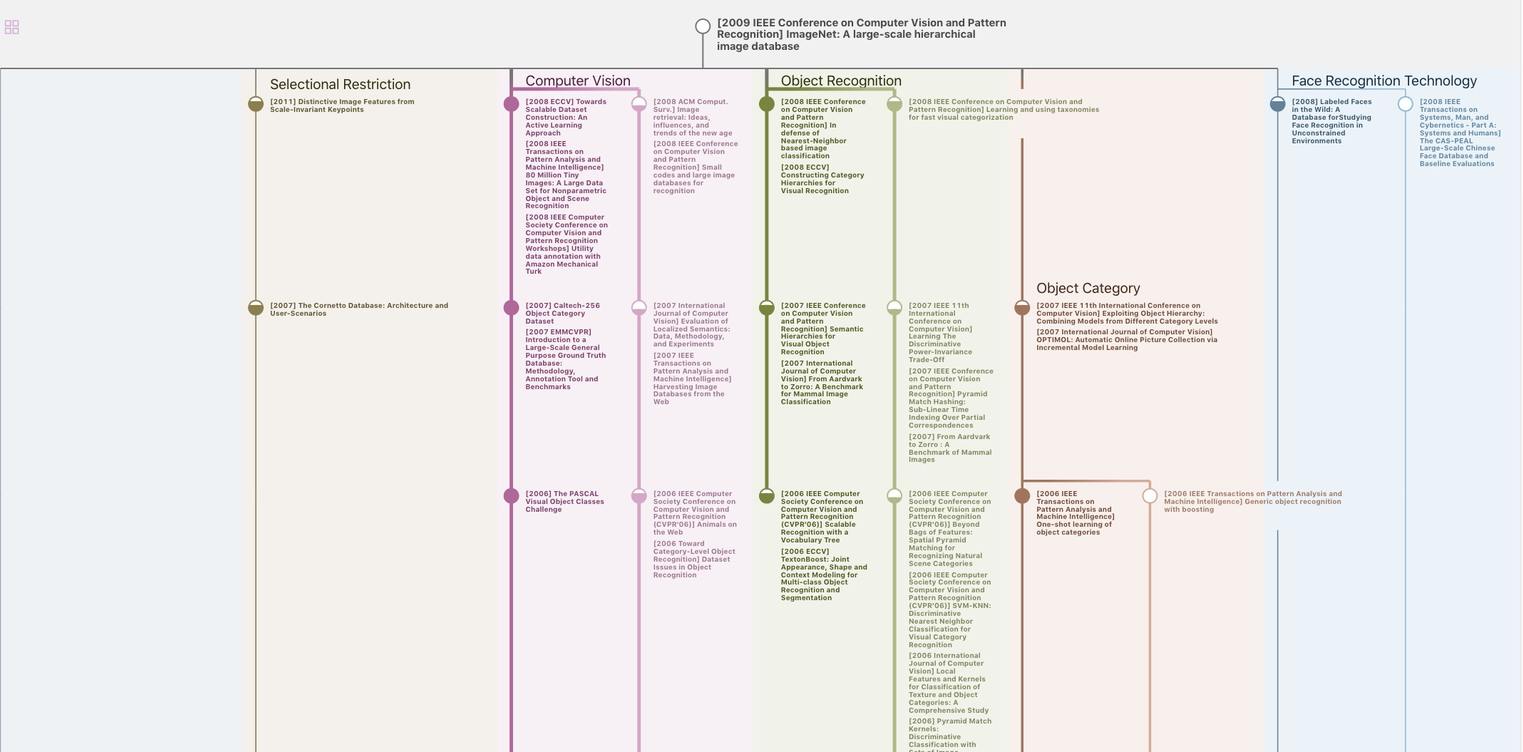
生成溯源树,研究论文发展脉络
Chat Paper
正在生成论文摘要