Collaborative filtering with adaptive information sources
ITWP'09: Proceedings of the 7th International Conference on Intelligent Techniques for Web Personalization & Recommender Systems - Volume 528(2009)
摘要
Collaborative filtering (CF) algorithms, which generate recommendations for web users by predicting user-item ratings, are often evaluated according to their predictions; in this context the problem of generating recommendations can be formulated as one of fitting a community of users to the best set of predictors. However, the data used to perform CF is sparse, and accuracy is limited by both the quantity and quality of information available. Mining the web has the potential to address these issues: the quality and quantity of ratings can be incremented by collecting external sources of rating information. In this work we introduce a method to perform CF with external data sources; furthermore, we show that a community of users can be partitioned according to what external source acts as a better predictor of each user's preferences. In particular, we find that a single kNN predictor can achieve remarkably high prediction accuracy if the data sources are selected optimally: designing a recommender system can thus be approached with the focus on data quality rather than algorithmic method.
更多查看译文
AI 理解论文
溯源树
样例
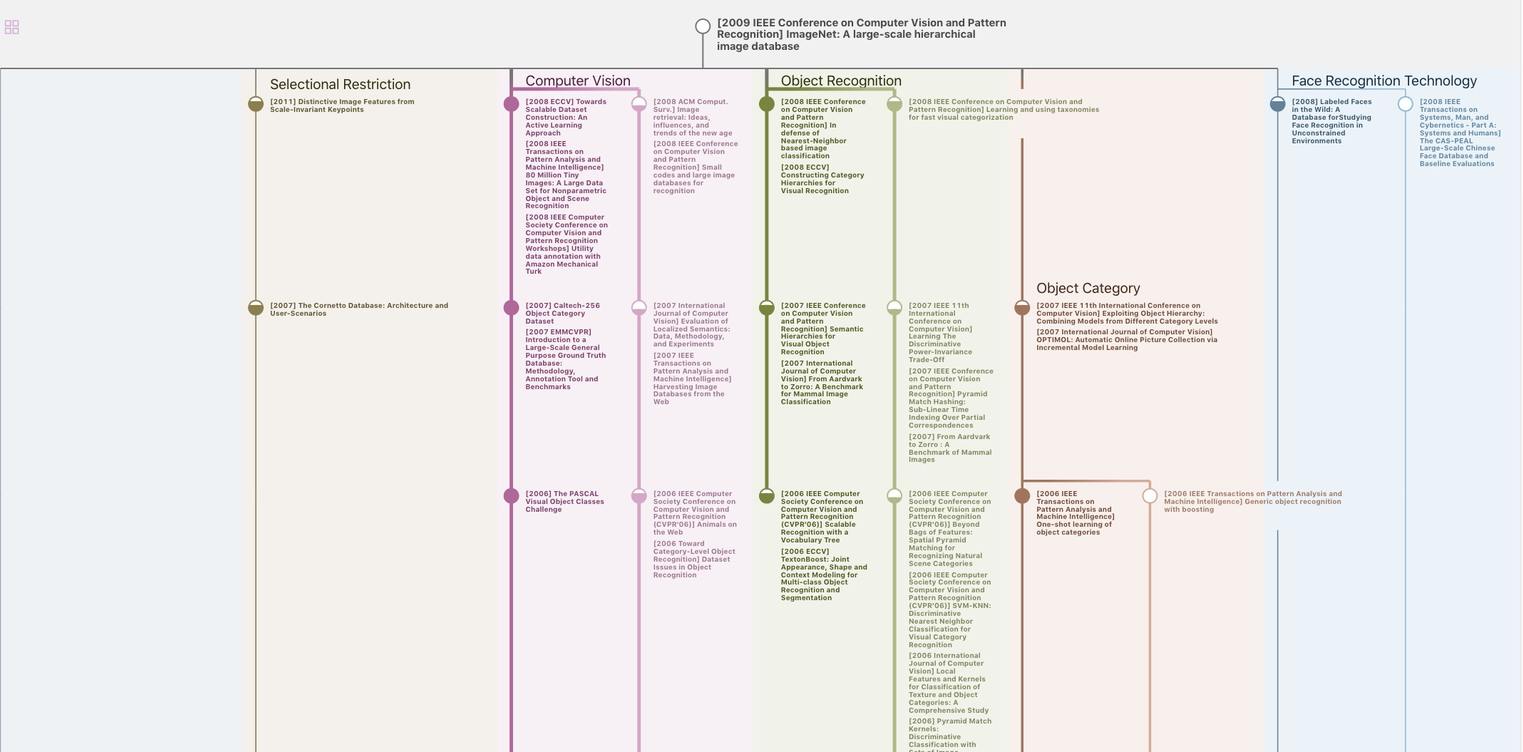
生成溯源树,研究论文发展脉络
Chat Paper
正在生成论文摘要