Model-Driven Data Acquisition in Sensor Networks
VLDB(2004)
摘要
Declarative queries are proving to be an attractive paradigm for in- teracting with networks of wireless sensors. The metaphor that "the sensornet is a database" is problematic, however, because sensors do not exhaustively represent the data in the real world. In order to map the raw sensor readings onto physical reality, a model of that reality is required to complement the readings. In this paper, we enrich interactive sensor querying with statistical modeling tech- niques. We demonstrate that such models can help provide answers that are both more meaningful, and, by introducing approximations with probabilistic confidences, significantly more efficient to com- pute in both time and energy. Utilizing the combination of a model and live data acquisition raises the challenging optimization prob- lem of selecting the best sensor readings to acquire, balancing the increase in the confidence of our answer against the communication and data acquisition costs in the network. We describe an expo- nential time algorithm for finding the optimal solution to this op- timization problem, and a polynomial-time heuristic for identifying solutions that perform well in practice. We evaluate our approach on several real-world sensor-network data sets, taking into account the real measured data and communication quality, demonstrating that our model-based approach provides a high-fidelity representation of the real phenomena and leads to significant performance gains ver- sus traditional data acquisition techniques.
更多查看译文
关键词
raw sensor reading,real phenomenon,real measured data,data acquisition cost,real world,real-world sensor-network data set,live data acquisition,sensor reading,model-driven data acquisition,sensor network,interactive sensor,traditional data acquisition technique,statistical model,data acquisition,polynomial time
AI 理解论文
溯源树
样例
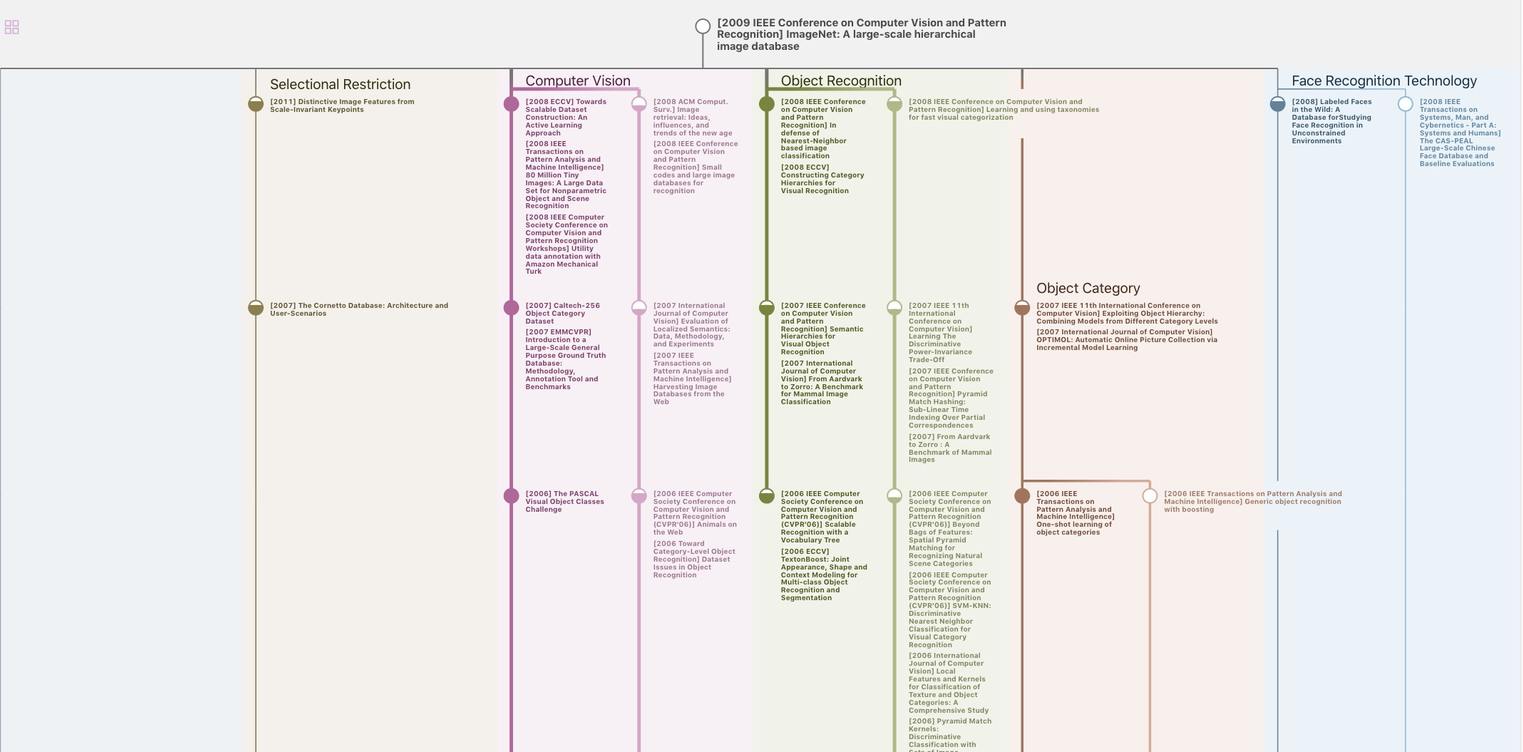
生成溯源树,研究论文发展脉络
Chat Paper
正在生成论文摘要