A Hierarchy of Recurrent Networks for Speech Recognition
msra(2009)
摘要
Generative models for sequential data based on directed graphs of Restricted Boltzmann Machines (RBMs) are able to accurately model high dimensional se- quences as recently shown. In these models, temporal dependencies in the input are discovered by either buffering previous visible variab les or by recurrent con- nections of the hidden variables. Here we propose a modificat ion of these models, the Temporal Reservoir Machine (TRM). It utilizes a recurrent artificial neural network (ANN) for integrating information from the input over time. This infor- mation is then fed into a RBM at each time step. To avoid difficu lties of recurrent network learning, the ANN remains untrained and hence can be thought of as a random feature extractor. Using the architecture of multi-layer RBMs (Deep Belief Networks), the TRMs can be used as a building block for complex hierar- chical models. This approach unifies RBM-based approaches f or sequential data modeling and the Echo State Network, a powerful approach for black-box system identification. The TRM is tested on a spoken digits task unde r noisy conditions, and competitive performances compared to previous models are observed.
更多查看译文
关键词
speech recognition
AI 理解论文
溯源树
样例
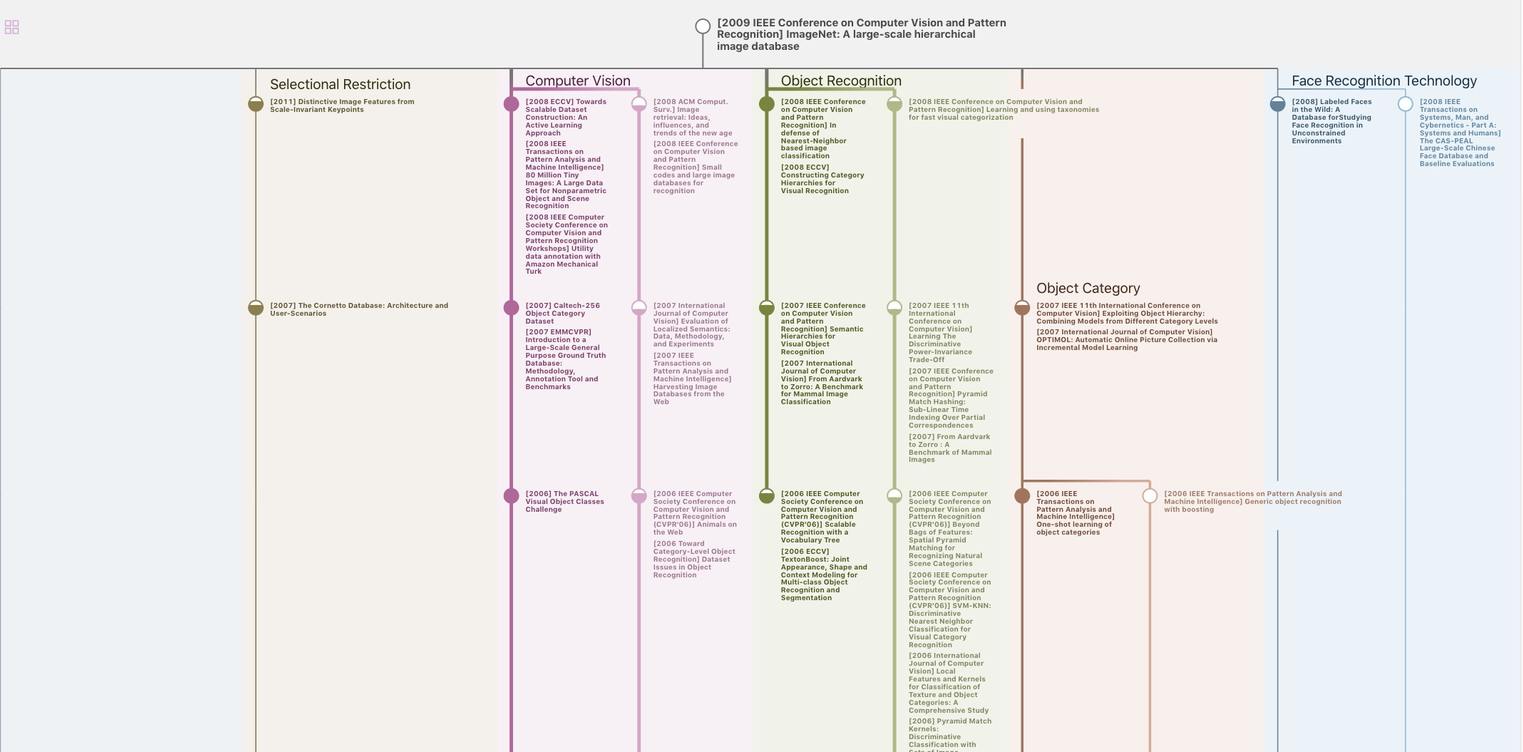
生成溯源树,研究论文发展脉络
Chat Paper
正在生成论文摘要