Adaptive Kernel Quantile Regression For Anomaly Detection
JOURNAL OF ADVANCED COMPUTATIONAL INTELLIGENCE AND INTELLIGENT INFORMATICS(2009)
摘要
In this paper, we study a problem of anomaly detection from time series-data. We use kernel quantile regression (KQR) to predict the extreme (such as 0.01 or 0.99) quantiles of the future time-series data distribution. It enables us to tell whether the probability of observing a certain time-series sequence is larger than, say, 1 percent or not. In this paper, we develop an efficient update algorithm of KQR in order to adapt the KQR in on-line manner. We propose a new algorithm that allows us to compute the optimal solution of the KQR when a new training pattern is inserted or deleted. We demonstrate the effectiveness of our methodology through numerical experiment using real-world time-series data.
更多查看译文
关键词
kernel machine, quantile regression and adaptive system
AI 理解论文
溯源树
样例
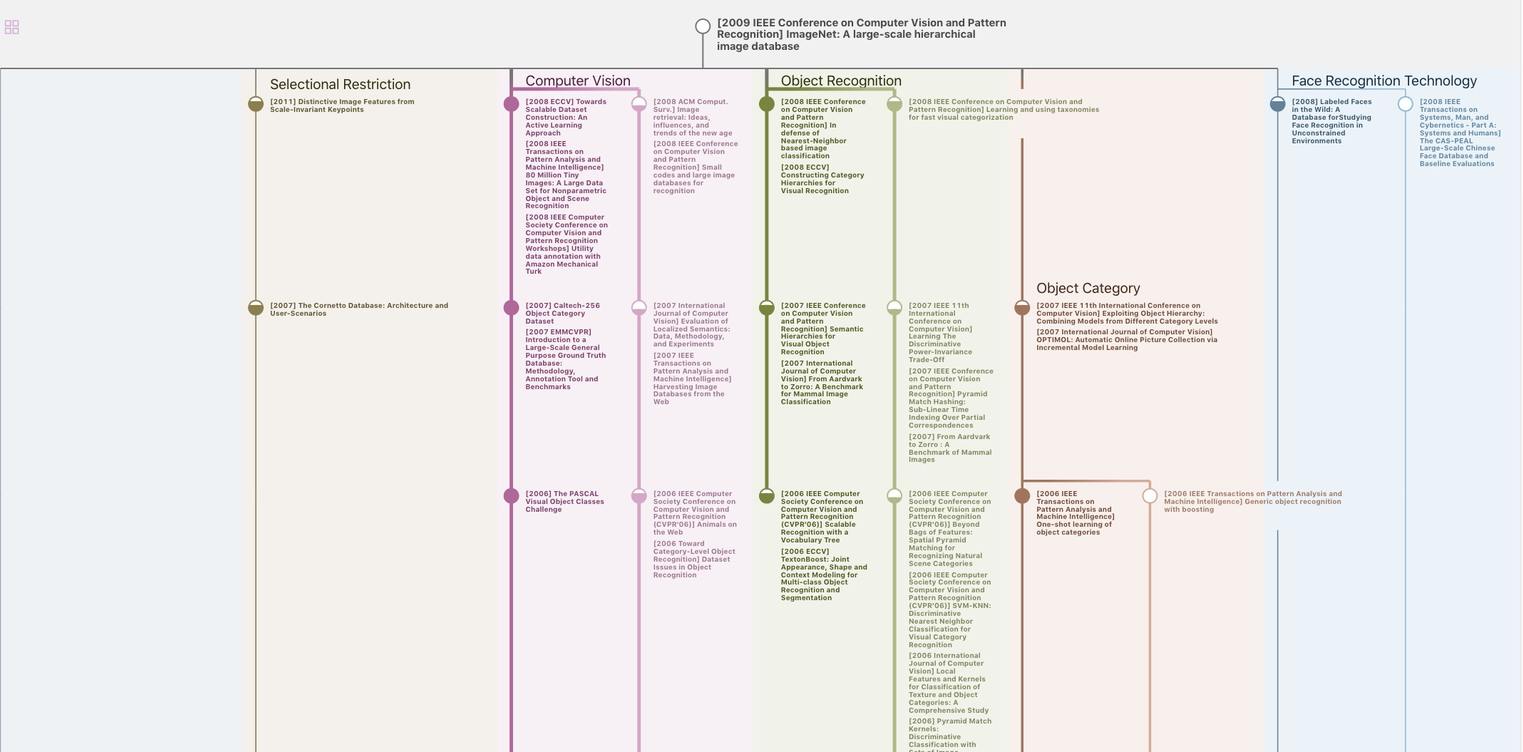
生成溯源树,研究论文发展脉络
Chat Paper
正在生成论文摘要