Learning related interactions among neuronal systems involved in memory processes
Journal of Physiology-Paris(2006)
摘要
Functional neuroimaging techniques using positron emission tomography (PET) and functional magnetic resonance imaging (fMRI) have provided new insights in our understanding of brain function from the molecular to the systems level. While subtraction strategy based data analyses have revealed the involvement of distributed brain regions in memory processes, covariance analysis based data analysis strategies allow functional interactions between brain regions of a neuronal network to be assessed. The focus of this chapter is to (1) establish the functional topography of episodic and working memory processes in young and old normal volunteers, (2) to assess functional interactions between modules of networks of brain regions by means of covariance based analyses and systems level modelling and (3) to relate neuroimaging data to the underpinning neural networks. Male normal young and old volunteers without neurological or psychiatric illness participated in neuroimaging studies (PET, fMRI) on working and episodic memory. Distributed brain areas are involved in memory processes (episodic and working memory) in young volunteers and show much of an overlap with respect to the network components. Systems level modelling analyses support the hypothesis of bihemispheric, asymmetric networks subserving memory processes and revealed both similarities in general and differences in the interactions between brain regions during episodic encoding and retrieval as well as working memory. Changes in memory function with ageing are evident from studies in old volunteers activating more brain regions compared to young volunteers and revealing more and stronger influences of prefrontal regions. We finally discuss the way in which the systems level models based on PET and fMRI results have implications for the understanding of the underlying neural network functioning of the brain.
更多查看译文
关键词
lPRC,lFPO,lDLPF,lPOC,lAC,lESC,lPAR,lPG,lTEMP,rPRC,rFPO,rDLPF,rPOC,rAC,rESC,rPAR,rPG,rTEMP
AI 理解论文
溯源树
样例
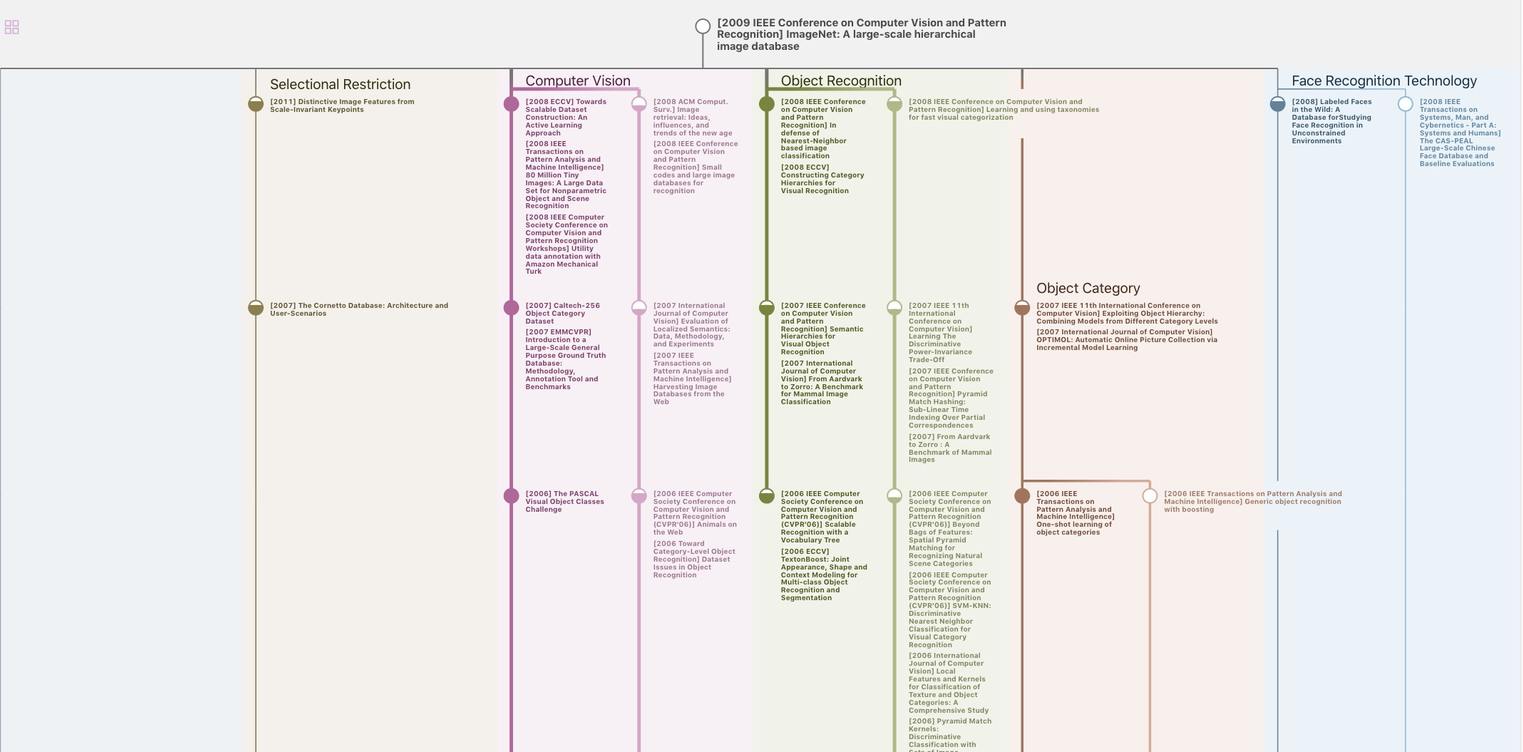
生成溯源树,研究论文发展脉络
Chat Paper
正在生成论文摘要