Recovering Quantitative Remote Sensing Products Contaminated by Thick Clouds and Shadows Using Multitemporal Dictionary Learning
IEEE T. Geoscience and Remote Sensing(2014)
摘要
With regard to quantitative remote sensing products in the visible and infrared ranges, thick clouds and accompanying shadows are an inevitable source of noise. Due to the absence of adequate supporting information from the data themselves, it is a formidable challenge to accurately restore the surficial information underlying large-scale clouds. In this paper, dictionary learning is expanded into the multitemporal recovery of quantitative data contaminated by thick clouds and shadows. This paper proposes two multitemporal dictionary learning algorithms, expanding on their KSVD and Bayesian counterparts. In order to make better use of the temporal correlations, the expanded KSVD algorithm seeks an optimized temporal path, and the expanded Bayesian method adaptively weights the temporal correlations. In the experiments, the proposed algorithms are applied to a reflectance product and a land surface temperature product, and the respective advantages of the two algorithms are investigated. The results show that, from both the qualitative visual effect and the quantitative objective evaluation, the proposed methods are effective.
更多查看译文
关键词
remote sensing,quantitative data,infrared range,atmospheric techniques,land surface temperature product,land surface temperature (lst),quantitative remote sensing products,reflectance,shadows,compressed sensing (cs),dictionary learning,reflectance product,thick clouds,large-scale clouds,expanded ksvd algorithm,quantitative remote sensing (qrs) product,land surface temperature,visible range,multitemporal dictionary learning,multitemporal,noise source,correlation,dictionaries
AI 理解论文
溯源树
样例
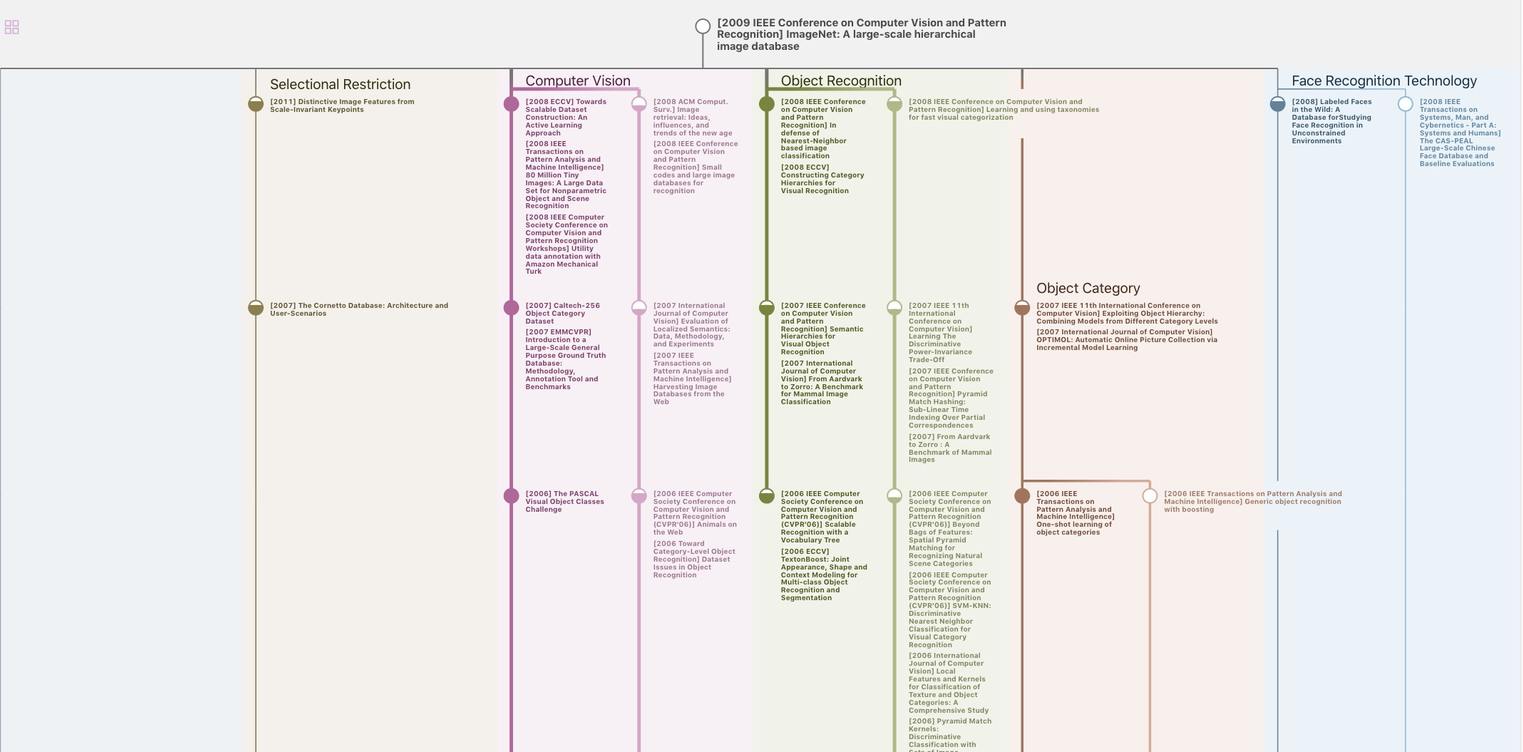
生成溯源树,研究论文发展脉络
Chat Paper
正在生成论文摘要