Automatically Generating Government Linked Data from Tables
national conference on artificial intelligence(2011)
摘要
Most open government data is encoded and published in structured tables found in reports, on the Web, and in spreadsheets or databases. Current approaches to gener- ating Semantic Web representations from such data re- quires human input to create schemas and often results in graphs that do not follow best practices for linked data. Evidence for a table's meaning can be found in its column headers, cell values, implicit relations between columns, caption and surrounding text but also requires general and domain-specific background knowledge. We describe techniques grounded in graphical models and probabilistic reasoning to infer meaning (seman- tics) associated with a table using background knowl- edge from the Linked Open Data cloud. We represent a table's meaning by mapping columns to classes in an appropriate ontology, linking cell values to literal con- stants, implied measurements, or entities in the linked data cloud (existing or new) and discovering or and identifying relations between columns.
更多查看译文
关键词
semantic web,probabilistic reasoning,graphical model,linked open data,linked data,best practice
AI 理解论文
溯源树
样例
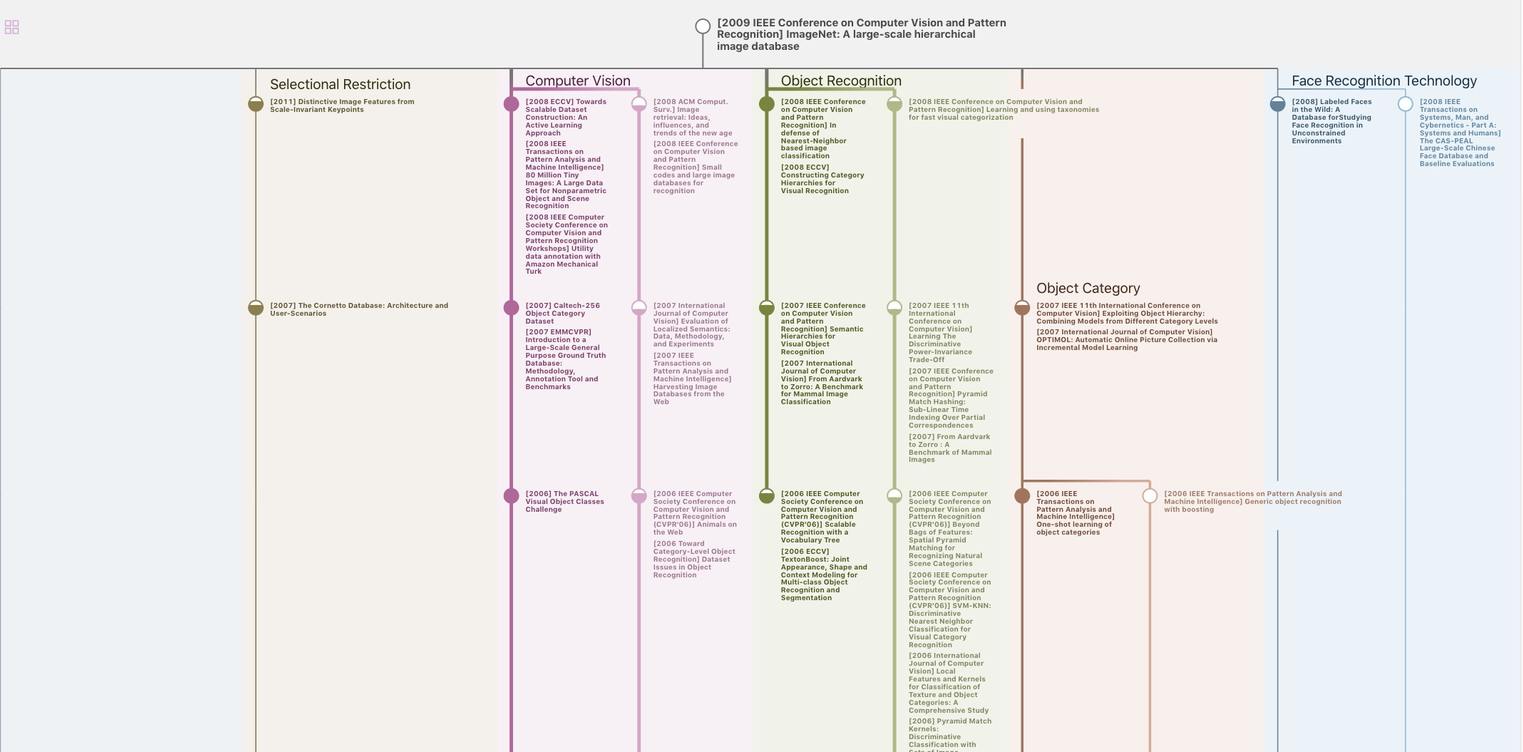
生成溯源树,研究论文发展脉络
Chat Paper
正在生成论文摘要