Image Compression by Learning to Minimize the Total Error
IEEE Transactions on Circuits and Systems for Video Technology(2013)
摘要
In this paper, we consider the problem of lossy image compression. Recently, machine learning techniques have been introduced as effective mechanisms for image compression. The compression involves storing only the grayscale image and a few carefully selected color pixel seeds. For decompression, regression models are learned with the stored data to predict the missing colors. This reduces image compression to standard active learning and semisupervised learning problems. In this paper, we propose a novel algorithm that makes use of all the colors (instead of only the colors of the selected seeds) available during the encoding stage. By minimizing the total color prediction error, our method can achieve a better compression ratio and better colorization quality than previous methods. The experimental results demonstrate the effectiveness of our proposed algorithm.
更多查看译文
关键词
image decompression,novel algorithm,colorization quality,grayscale image,compression ratio,semisupervised learning,image coding,learning (artificial intelligence),standard active learning problem,regression analysis,encoding stage,data compression,machine learning techniques,active learning,total color prediction error,regression models,color pixel seeds,semisupervised learning problem,lossy image compression,image compression,gray scale,learning artificial intelligence,vectors,kernel,machine learning,prediction algorithms
AI 理解论文
溯源树
样例
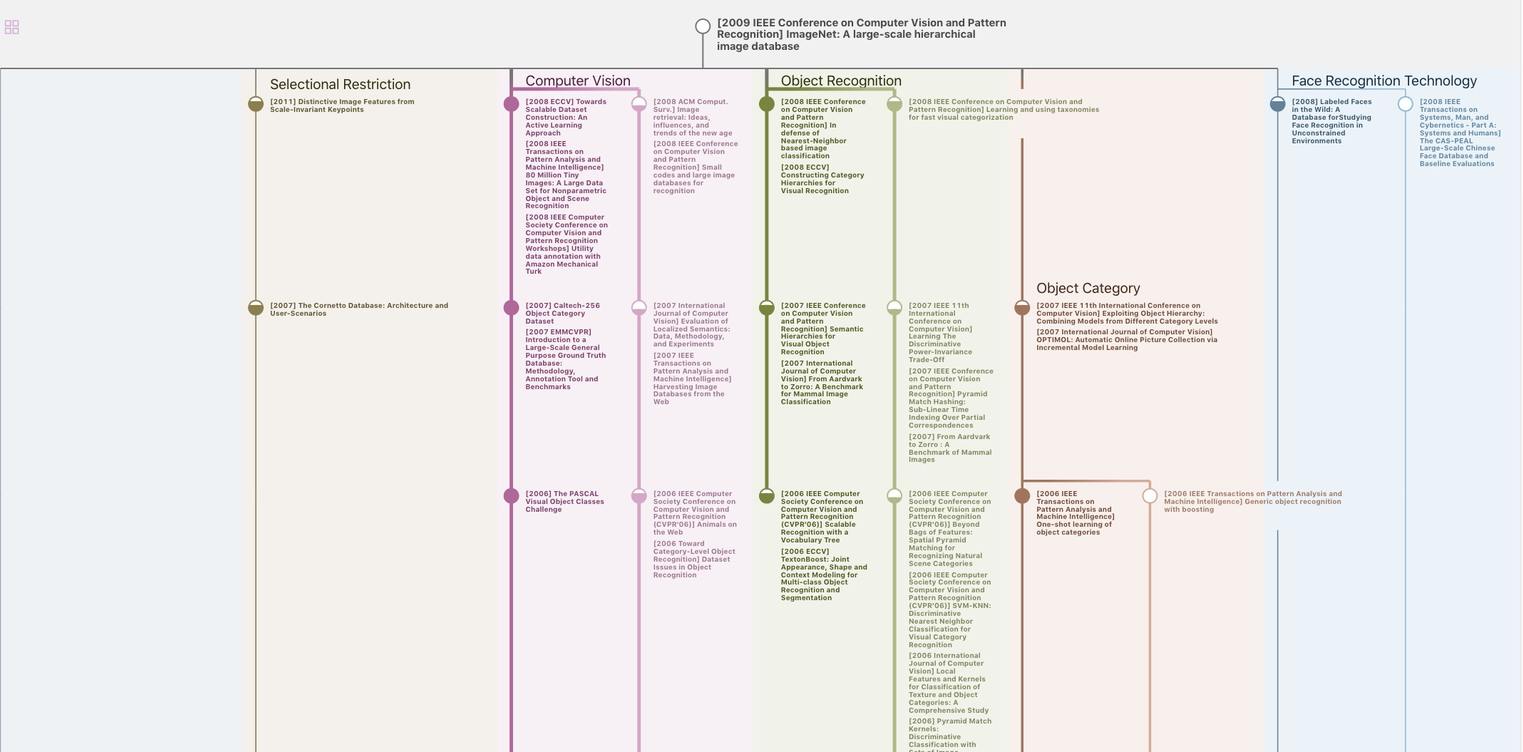
生成溯源树,研究论文发展脉络
Chat Paper
正在生成论文摘要