Real-time human detection based on gentle MILBoost with variable granularity HOG-CSLBP
Neural Computing and Applications(2012)
摘要
Sample misalignment exerts an important influence on training a rapid and accurate human detector, and it is a difficult problem to tackle with due to human articulation or manual annotation errors. Multiple instances learning method is an effective tool to deal with this difficulty without manual correction. In this paper, firstly, we propose a variable granularity HOG-CSLBP feature, which combines the human shape information with local texture information, and encodes spatial relationship in different granularity to improve its discriminative ability. Our new feature takes an advantage of the mutual complementarities of histogram of gradient and center-symmetric local binary patterns feature, which is adept at modeling human. Secondly, we present a Gentle MILBoost algorithm which utilizes the Newton update technique to get an optimal weak classifier that is able to discriminate complex distribution and is more stable in numerical computation. Experimental results based on INRIA, MIT-CBCL and TUD-Brussels datasets have showed superior performance of our method. Moreover, our method can achieve real-time speed in real application.
更多查看译文
关键词
Sample alignment,Human detection,HOG-CSLBP,Gentle MILBoost
AI 理解论文
溯源树
样例
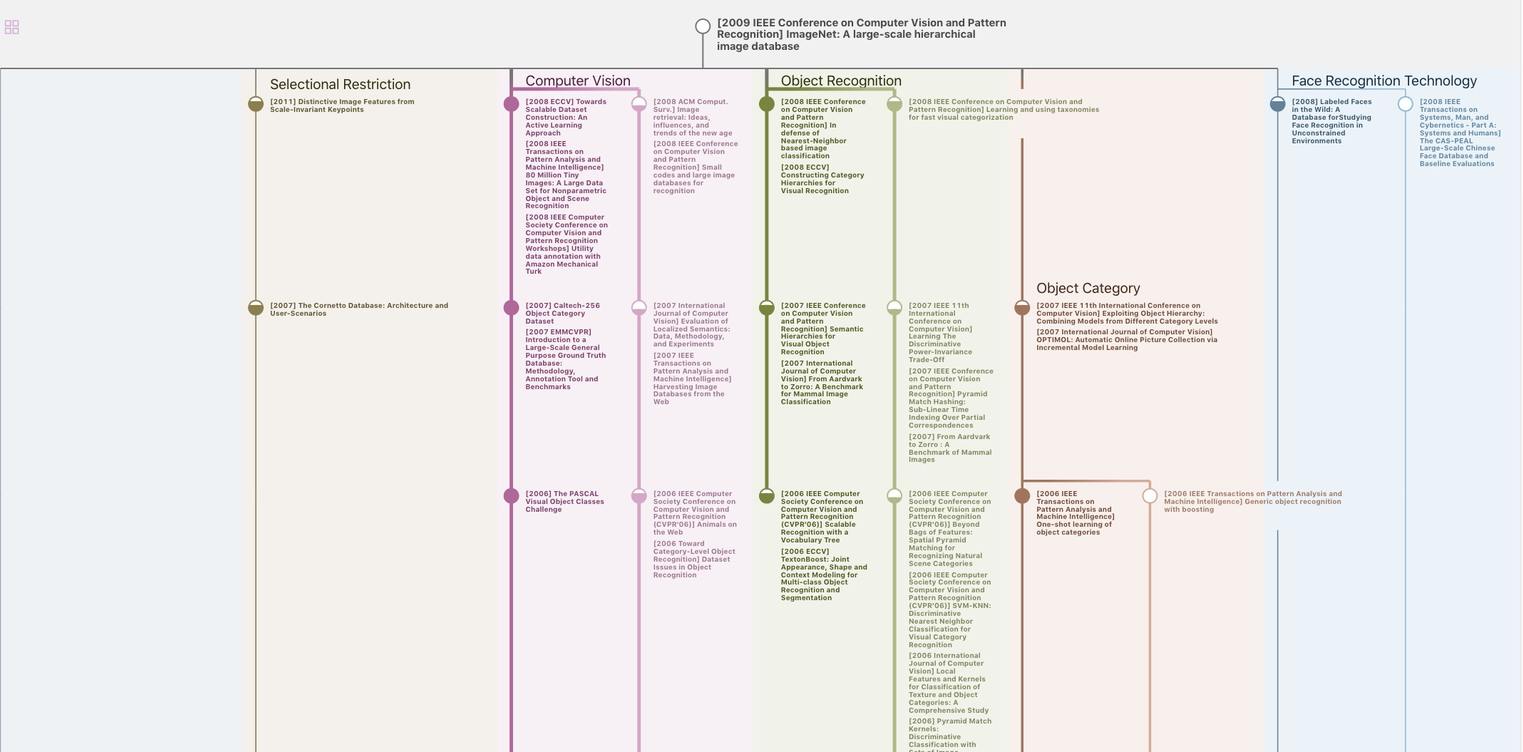
生成溯源树,研究论文发展脉络
Chat Paper
正在生成论文摘要