Subspace-Based Feature Representation And Learning For Language Recognition
13TH ANNUAL CONFERENCE OF THE INTERNATIONAL SPEECH COMMUNICATION ASSOCIATION 2012 (INTERSPEECH 2012), VOLS 1-3(2012)
摘要
This paper presents a novel subspace-based approach for phonotactic language recognition. The whole framework is divided into two parts: the speech feature representation and the subspace-based learning algorithm. First, the phonetic information as well as the contextual relationship, possessed by spoken utterances, are more abundantly retrieved by likelihood computation and feature concatenation through the decoding processed by an automatic speech recognizer. It is assumed that the extracted phone frames reside in a lower dimensional eigen-subspace, in which the structure of data can be approximately captured. Each utterance is further represented by a fixed-dimensional linear subspace. Second, to measure the similarity between two utterances, suitable non-Euclidean metrics are explored and applied to non-linear discriminant analysis in a kernel fashion, followed by a back-end classifier, such as the k-nearest neighbor (K-NN) classifier. The results of experiments on the OGI-TS database demonstrate that the proposed framework outperforms the well-known vector space modeling based method with relative reductions of 38.90% and 27.13% on the 1-to-50-second and 3-second data sets respectively in equal error rate (EER).
更多查看译文
关键词
language recognition,subspace-based learning
AI 理解论文
溯源树
样例
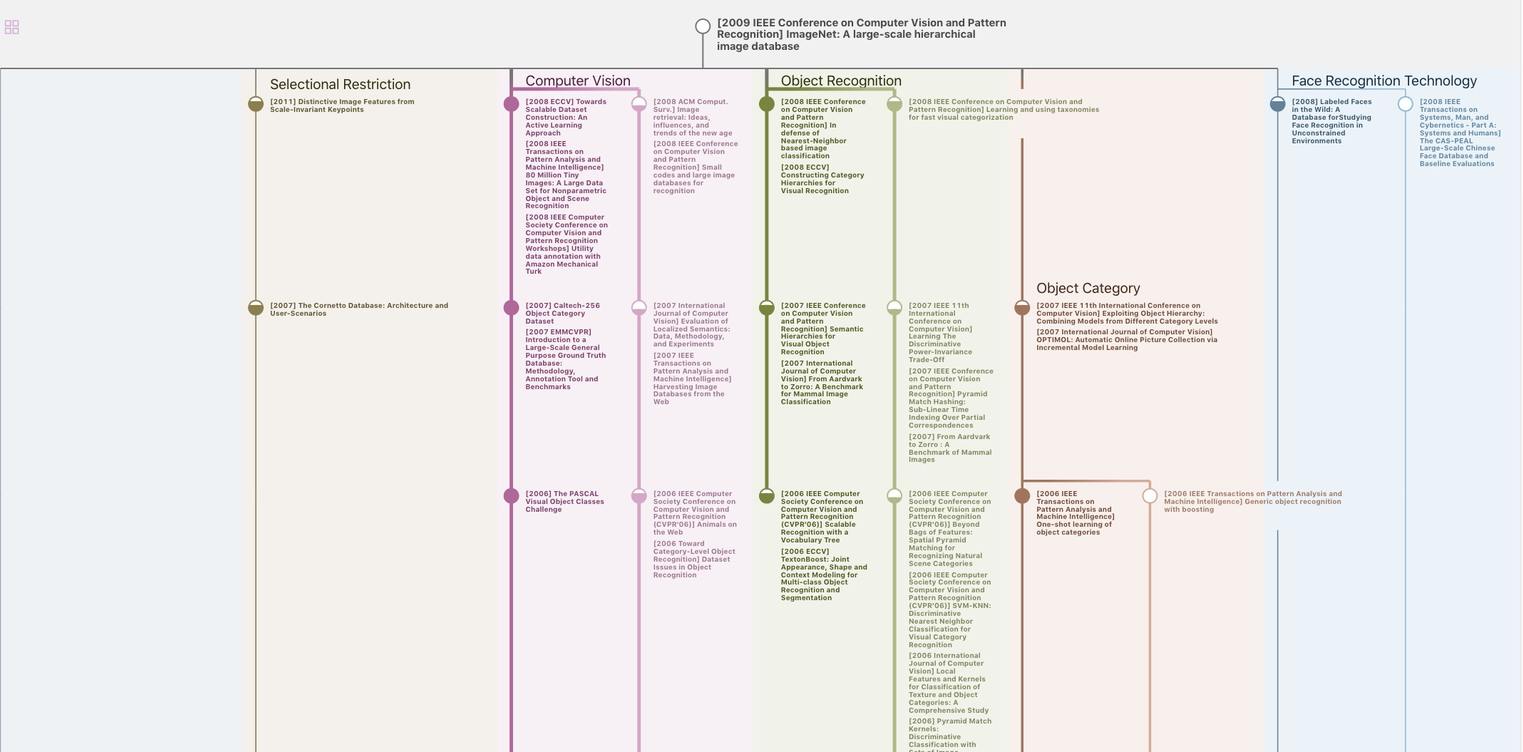
生成溯源树,研究论文发展脉络
Chat Paper
正在生成论文摘要