Unsupervised domain adaptation of virtual and real worlds for pedestrian detection
Pattern Recognition(2012)
摘要
Vision-based object detectors are crucial for different applications. They rely on learnt object models. Ideally, we would like to deploy our vision system in the scenario where it must operate. Then, the system should self-learn how to distinguish the objects of interest, i.e., without human intervention. However, the learning of each object model requires labelled samples collected through a tiresome manual process. For instance, we are interested in exploring the self-training of a pedestrian detector for driver assistance systems. Our first approach to avoid manual labelling consisted in the use of samples coming from realistic computer graphics, so that their labels are automatically available [12]. This would make possible the desired self-training of our pedestrian detector. However, as we showed in [14], between virtual and real worlds it may be a dataset shift. In order to overcome it, we propose the use of unsupervised domain adaptation techniques that avoid human intervention during the adaptation process. In particular, this paper explores the use of the transductive SVM (T-SVM) learning algorithm in order to adapt virtual and real worlds for pedestrian detection (Fig. 1).
更多查看译文
关键词
computer graphics,computer vision,driver information systems,object detection,pedestrians,support vector machines,unsupervised learning,virtual reality,T-SVM learning algorithm,adaptation process,computer graphics,dataset shift,driver assistance systems,human intervention,labelled samples collection,manual labelling,object model learning,object models,pedestrian detection,pedestrian detector,real worlds,self-training,tiresome manual process,transductive SVM learning algorithm,unsupervised domain adaptation,unsupervised domain adaptation techniques,virtual worlds,vision system,vision-based object detectors
AI 理解论文
溯源树
样例
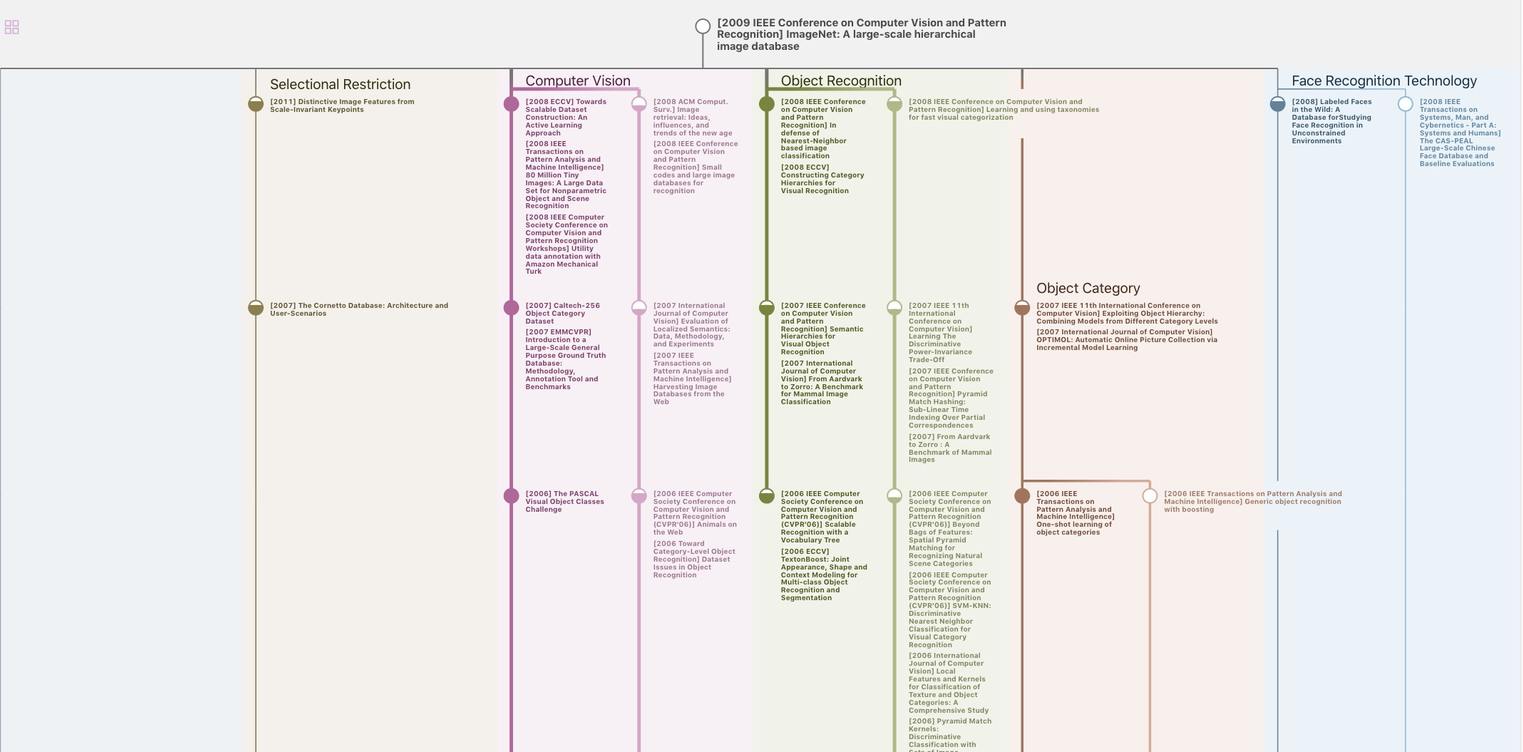
生成溯源树,研究论文发展脉络
Chat Paper
正在生成论文摘要