Evolutionary Learning of Goal Priorities in a Real-Time Strategy Game.
AIIDE'12: Proceedings of the Eighth AAAI Conference on Artificial Intelligence and Interactive Digital Entertainment(2012)
摘要
We present a drive-based agent capable of playing the realtime strategy computer game Starcraft. Success at this task requires the ability to engage in autonomous, goal-directed behaviour, as well as techniques to manage the problem of potential goal conflicts. To address this, we show how a case-injected genetic algorithm can be used to learn goal priority profiles for use in goal management. This is achieved by learning how goals might be re-prioritised under certain operating conditions, and how priority profiles can be used to dynamically guide high-level strategies. Our dynamic system shows greatly improved results over a version equipped with static knowledge, and a version that only partially exploits the space of learned strategies. However, our work raises questions about how a system must know about its own design in order to best exploit its own competences.
更多查看译文
AI 理解论文
溯源树
样例
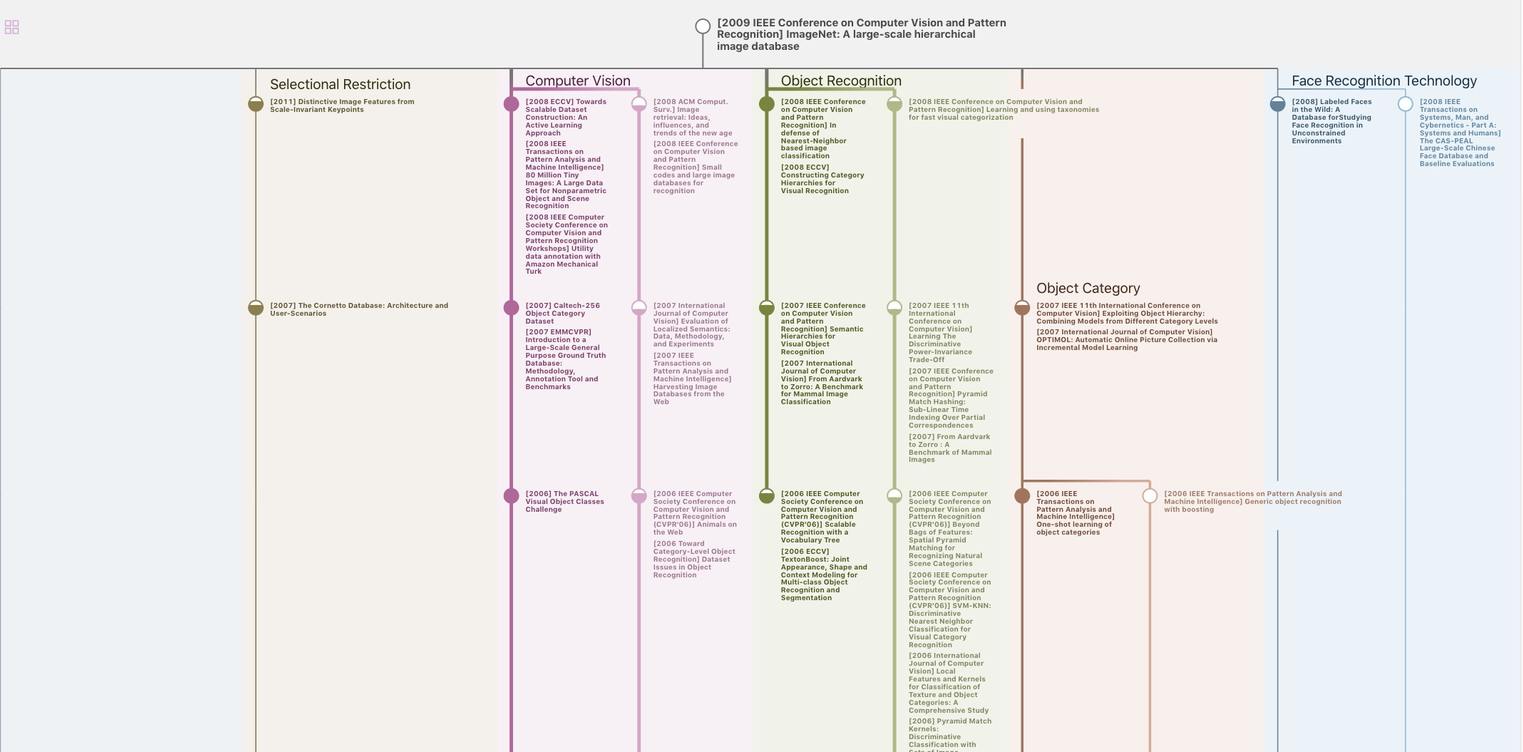
生成溯源树,研究论文发展脉络
Chat Paper
正在生成论文摘要