Boosting Shape Registration Algorithms Via Reproducing Kernel Hilbert Space Regularizers
IEEE ROBOTICS AND AUTOMATION LETTERS(2019)
摘要
The essence of most shape registration algorithms is to find correspondences between two point clouds and then to solve for a rigid body transformation that aligns the geometry. The main drawback is that the point clouds are obtained by placing the sensor at different views; consequently, the two matched points most likely do not correspond to the same physical point in the real environment. In other words, the point cloud is a discrete representation of the shape geometry. Alternatively, a point cloud measurement can be seen as samples from geometry, and a function can be learned for a continuous representation using regression techniques such as kernel methods. To boost registration algorithms, this work develops a novel class of regularizers modeled in the Reproducing Kernel Hilbert Space (RKHS) that ensures correspondences are also consistent in an abstract vector space of functions such as intensity surface. Furthermore, the proposed RKHS regularizer is agnostic to the choice of the registration cost function which is desirable. The evaluations on experimental data confirm the effectiveness of the proposed regularizer using RGB-D and LIDAR sensors.
更多查看译文
关键词
Three-dimensional displays, Kernel, Hilbert space, Shape, Laser radar, Image color analysis, Robot sensing systems, Range sensing, RGB-D perception, localization
AI 理解论文
溯源树
样例
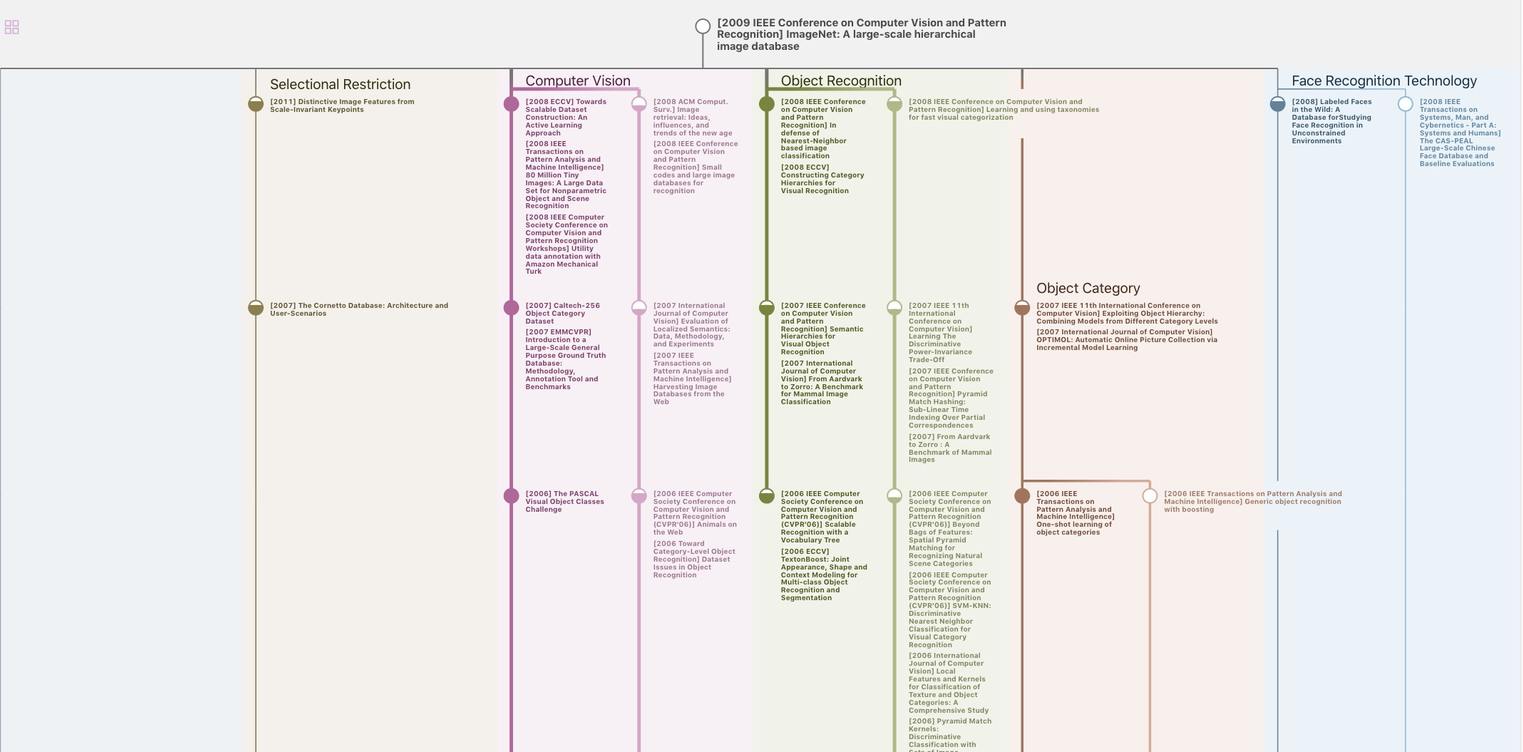
生成溯源树,研究论文发展脉络
Chat Paper
正在生成论文摘要