Multiclass Semi-Supervised Boosting Using Similarity Learning
2013 IEEE 13TH INTERNATIONAL CONFERENCE ON DATA MINING (ICDM)(2013)
摘要
In this paper, we consider the multiclass semi-supervised classification problem. A boosting algorithm is proposed to solve the multiclass problem directly. The proposed multiclass approach uses a new multiclass loss function, which includes two terms. The first term is the cost of the multiclass margin and the second term is a regularization term on unlabeled data. The regularization term is used to minimize the inconsistency between the pairwise similarity and the classifier predictions. It assigns the soft labels weighted with the similarity between unlabeled and labeled examples. We then derive a boosting algorithm, named CD-MSSBoost, from the proposed loss function using coordinate gradient descent. The derived algorithm is further used for learning optimal similarity function for a given data. Our experiments on a number of UCI datasets show that CD-MSSBoost outperforms the state-of-the-art methods to multiclass semi-supervised learning.
更多查看译文
关键词
Semi-Supervised Learning, Boosting, Multiclass classification, Similarity learning
AI 理解论文
溯源树
样例
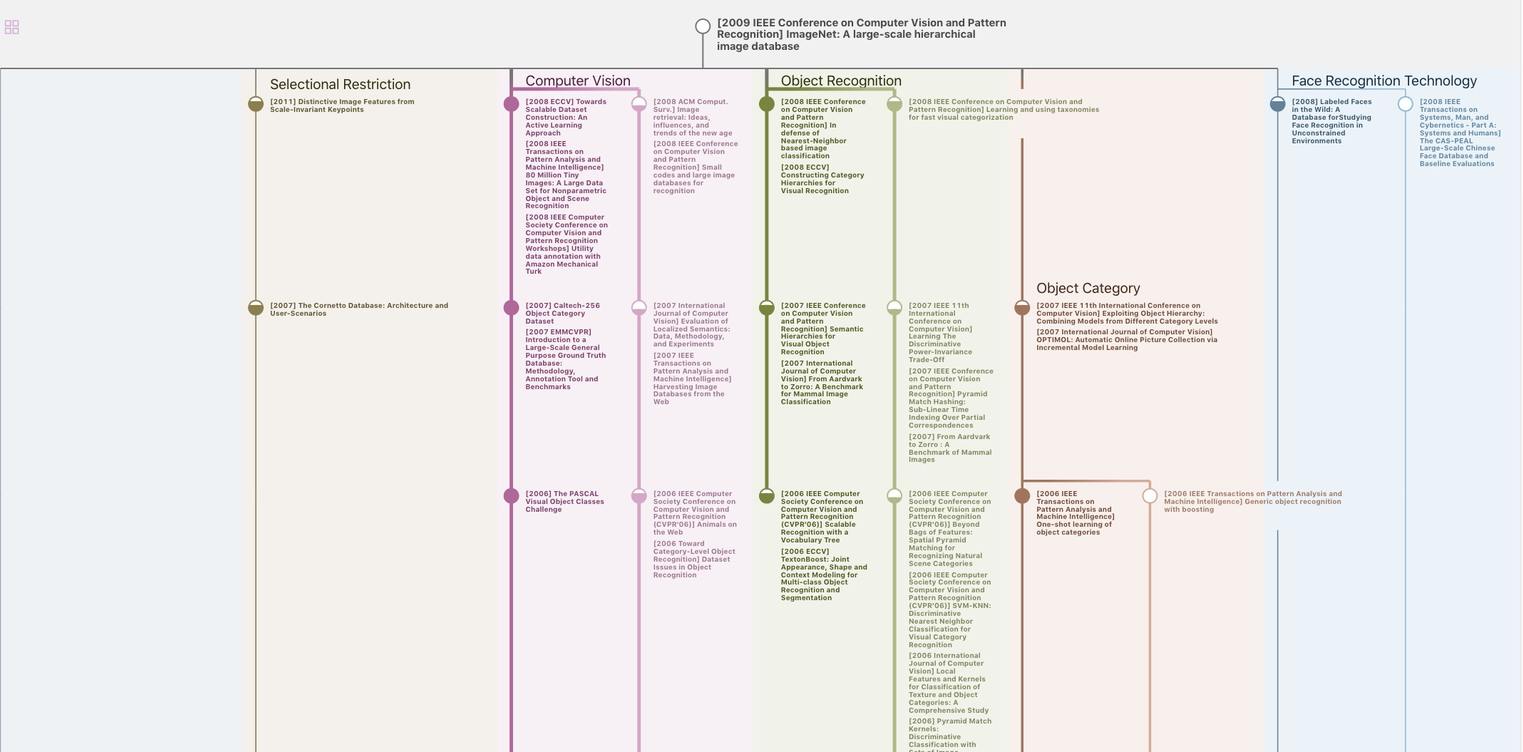
生成溯源树,研究论文发展脉络
Chat Paper
正在生成论文摘要