Optimizing Number Of Hidden Neurons In Neural Networks
AIAP'07: Proceedings of the 25th conference on Proceedings of the 25th IASTED International Multi-Conference: artificial intelligence and applications(2007)
摘要
In this paper, a novel and effective criterion based on the estimation of the signal-to-noise-ratio figure (SNRF) is proposed to optimize the number of hidden neurons in neural networks to avoid overfitting in the function approximation. SNRF can quantitatively measure the useful information left unlearned so that overfitting can be automatically detected from the training error only without use of a separate validation set. It is illustrated by optimizing the number of hidden neurons in a multi-layer perceptron (MLP) using benchmark datasets. The criterion can be further utilized in the optimization of other parameters of neural networks when overfitting needs to be considered.
更多查看译文
关键词
neural network,network optimization,function approximation,overfitting,signal-to-noise ratio figure
AI 理解论文
溯源树
样例
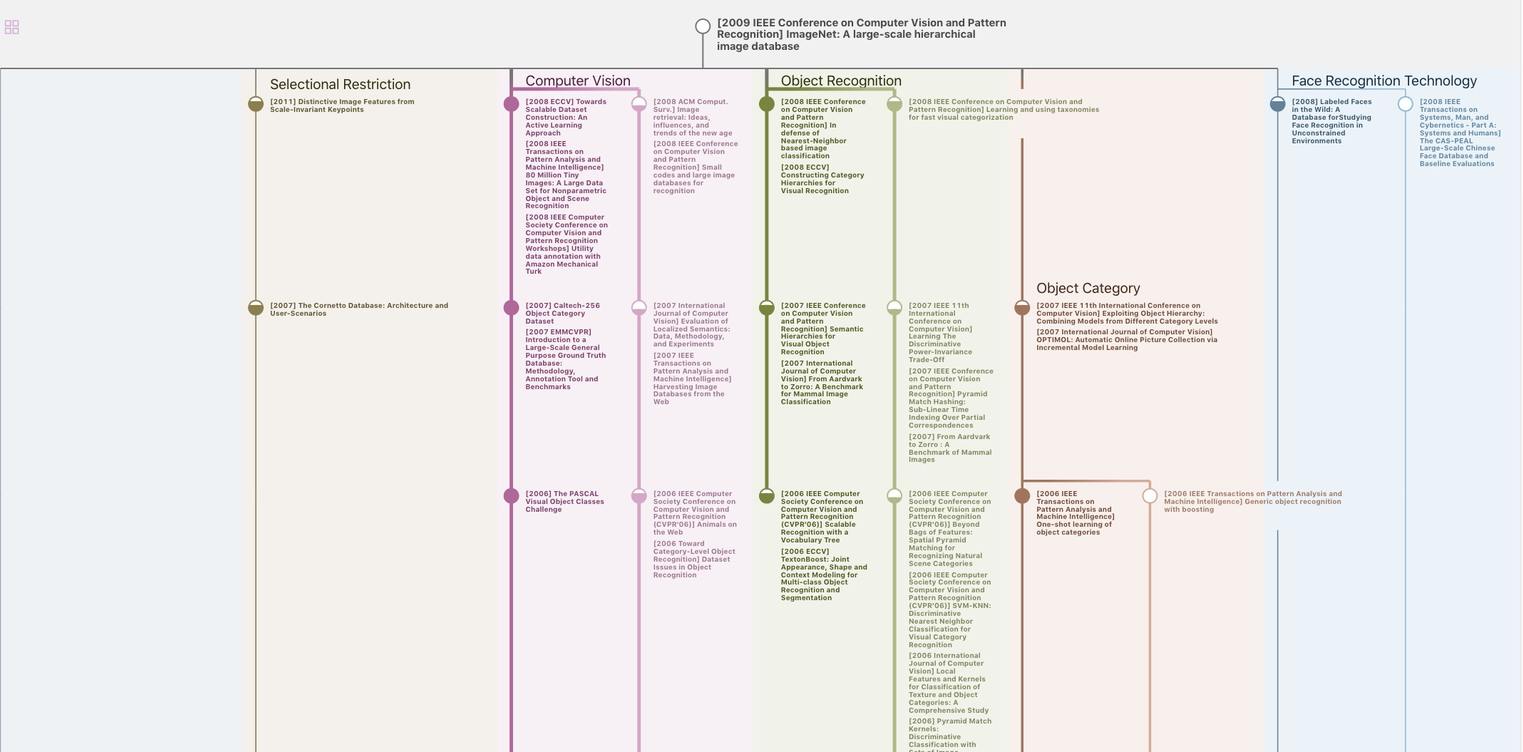
生成溯源树,研究论文发展脉络
Chat Paper
正在生成论文摘要