Learning Object Location Predictors with Boosting and Grammar-Guided Feature Extraction
BMVC(2009)
摘要
We present BEAMER: a new spatially exploitative approach to learning object detectors which shows excellent results when applied to the task of detecting objects in greyscale aerial imagery in the presence of ambiguous and noisy data. There are four main contributions used to produce these results. First, we introduce a grammar-guided feature extraction system, enabling the exploration of a richer feature space while constraining the features to a useful subset. This is specified with a rule-based generative grammar crafted by a human expert. Second, we learn a classifier on this data using a newly proposed variant of AdaBoost which takes into account the spatially correlated nature of the data. Third, we perform another round of training to optimize the method of converting the pixel classifications generated by boosting into a high quality set of (x, y) locations. Lastly, we carefully define three common problems in object detection and define two evaluation criteria that are tightly matched to these problems. Major strengths of this approach are: (1) a way of randomly searching a broad feature space, (2) its performance when evaluated on well-matched evaluation criteria, and (3) its use of the location prediction domain to learn object detectors as well as to generate detections that perform well on several tasks: object counting, tracking, and target detection. We demonstrate the efficacy of BEAMER with a comprehensive experimental evaluation on a challenging data set.
更多查看译文
关键词
feature space,performance,feature extraction,pattern recognition,rule based,forecasting,evaluation,exploration,spatial correlation
AI 理解论文
溯源树
样例
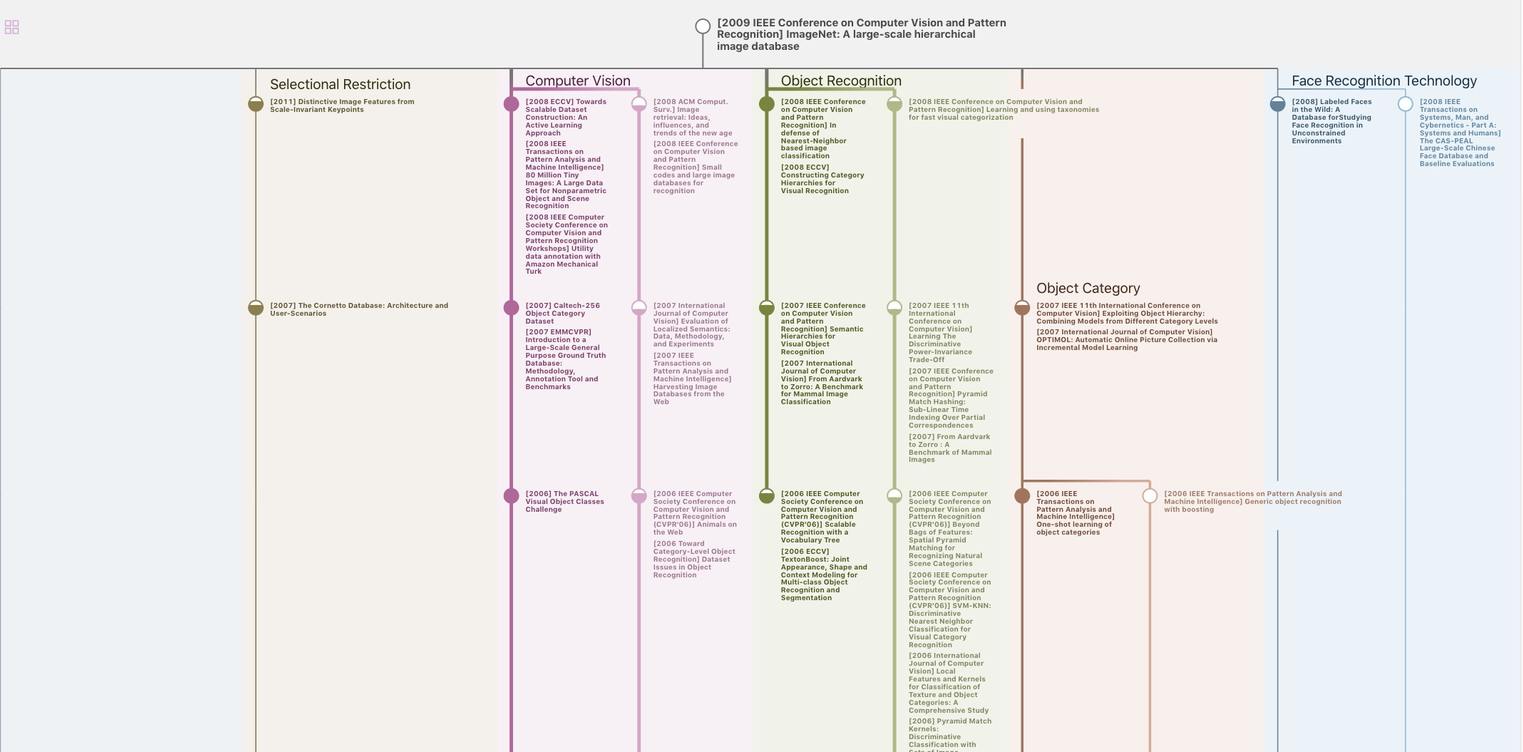
生成溯源树,研究论文发展脉络
Chat Paper
正在生成论文摘要