Sparse margin–based discriminant analysis for feature extraction
Neural Computing and Applications(2013)
摘要
The existing margin-based discriminant analysis methods such as nonparametric discriminant analysis use K-nearest neighbor (K-NN) technique to characterize the margin. The manifold learning–based methods use K-NN technique to characterize the local structure. These methods encounter a common problem, that is, the nearest neighbor parameter K should be chosen in advance. How to choose an optimal K is a theoretically difficult problem. In this paper, we present a new margin characterization method named sparse margin–based discriminant analysis (SMDA) using the sparse representation. SMDA can successfully avoid the difficulty of parameter selection. Sparse representation can be considered as a generalization of K-NN technique. For a test sample, it can adaptively select the training samples that give the most compact representation. We characterize the margin by sparse representation. The proposed method is evaluated by using AR, Extended Yale B database, and the CENPARMI handwritten numeral database. Experimental results show the effectiveness of the proposed method; its performance is better than some other state-of-the-art feature extraction methods.
更多查看译文
关键词
Dimensional reduction,Feature extraction,Sparse margin
AI 理解论文
溯源树
样例
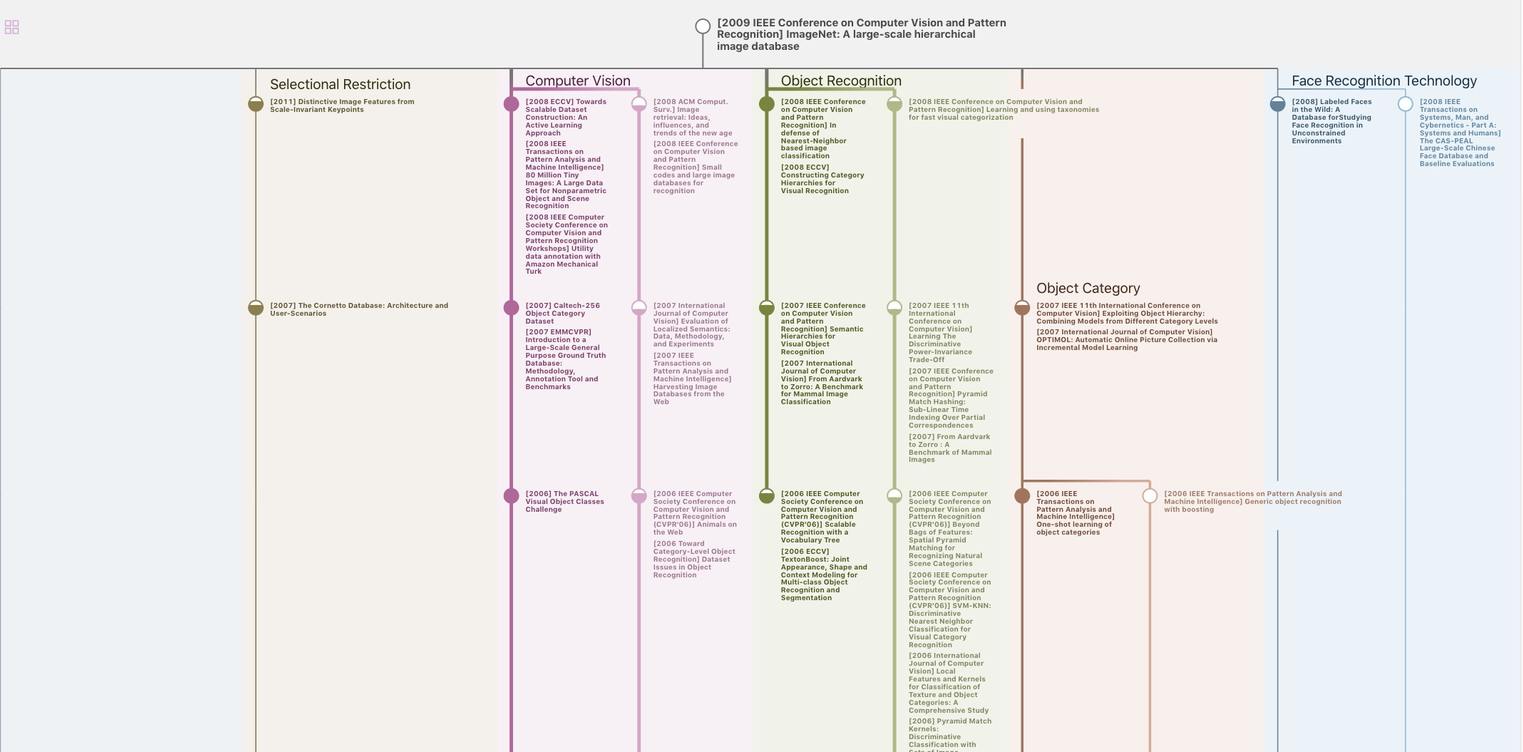
生成溯源树,研究论文发展脉络
Chat Paper
正在生成论文摘要