Empirically Testing Decision Making in TAC SCM
msra(2007)
摘要
Design decisions for TAC SCM agents are usually evaluated empirically by running complete agents against each other. While this approach is sufficient for many purposes, it be difficult to use it for running large-scale, controlled experiments to evaluate particular aspects of an agent’s design. This is true both for technical reasons (availability of other agent code, the trouble of setting up a TAC server, etc.) and especially because results can depend heavily on the experimenter’s choice of opponent agents. This paper introduces a novel model of the TAC SCM scheduling problem for use in such empirical evaluations. The model aims to reduce the experimental variability caused by the experimenter’s choice of opponent agents, replacing markets with stochastic processes that simulate them. These stochastic processes are designed by using machine learning to distill typical agent behaviors from real game logs taken from the TAC SCM finals. After describing the operation of our model, we validate it by showing that its predictions of opponent behavior are highly consistent with further game logs that were not used for building the model. Finally, we apply our model to investigate the performance of several integer/linear programming approaches for solving the delivery and scheduling subproblems in TAC SCM.
更多查看译文
AI 理解论文
溯源树
样例
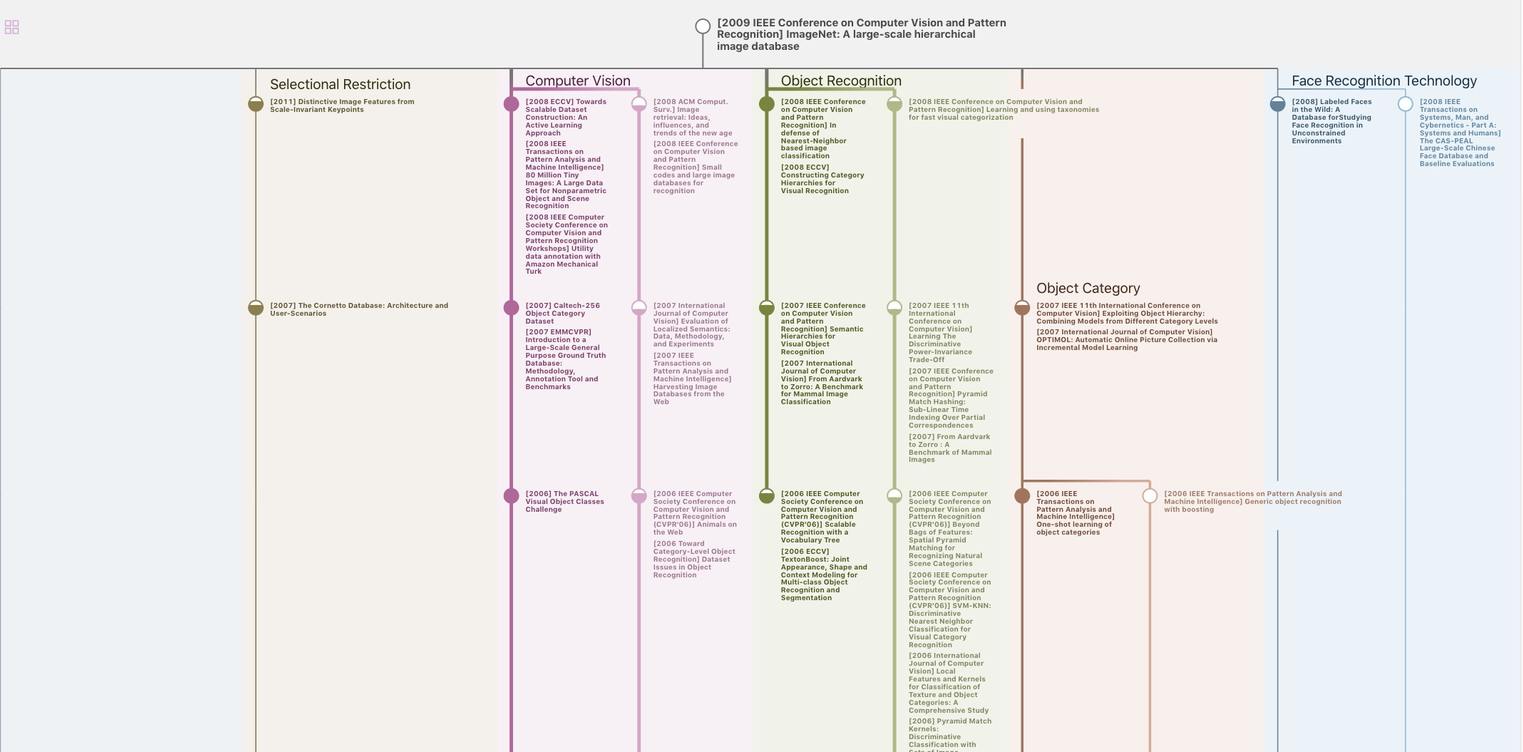
生成溯源树,研究论文发展脉络
Chat Paper
正在生成论文摘要