Learning-based deformable registration using weighted mutual information
ICPR(2012)
摘要
Deformable registration of multi-modality medical image remains a challenging research topic. The incorporation of prior information on the expected joint distribution has shown to noticeably improve registration accuracy and robustness. However, direct application of the learned joint histogram makes the algorithm sensitive to the difference between the training data and the test image. This paper explores a more intrinsic intensity mapping relationship using normalized pointwise mutual information, and integrates the learned relationship into the conventional mutual information (MI) to formulate a weighted mutual information (WMI). We further derive a closed-form expression of the first variation of WMI for non-parametric de-formable registration in a variational framework. Experiment results show that the proposed WMI is more accurate and robust than MI, and is less sensitive to discrepancies between the training and test images, compared to the method in [1]. In addition, our prior can be learned from only a subset of the image, and can be object-specific.
更多查看译文
关键词
variational techniques,nonparametric deformable registration,wmi,learning (artificial intelligence),learning-based deformable registration,test image,joint distribution,normalized pointwise mutual information,biomedical mri,joint histogram learning,variational framework,mri,weighted mutual information,multimodality medical image,prior information,image registration,intrinsic intensity mapping relationship,medical image processing,training data,learning artificial intelligence
AI 理解论文
溯源树
样例
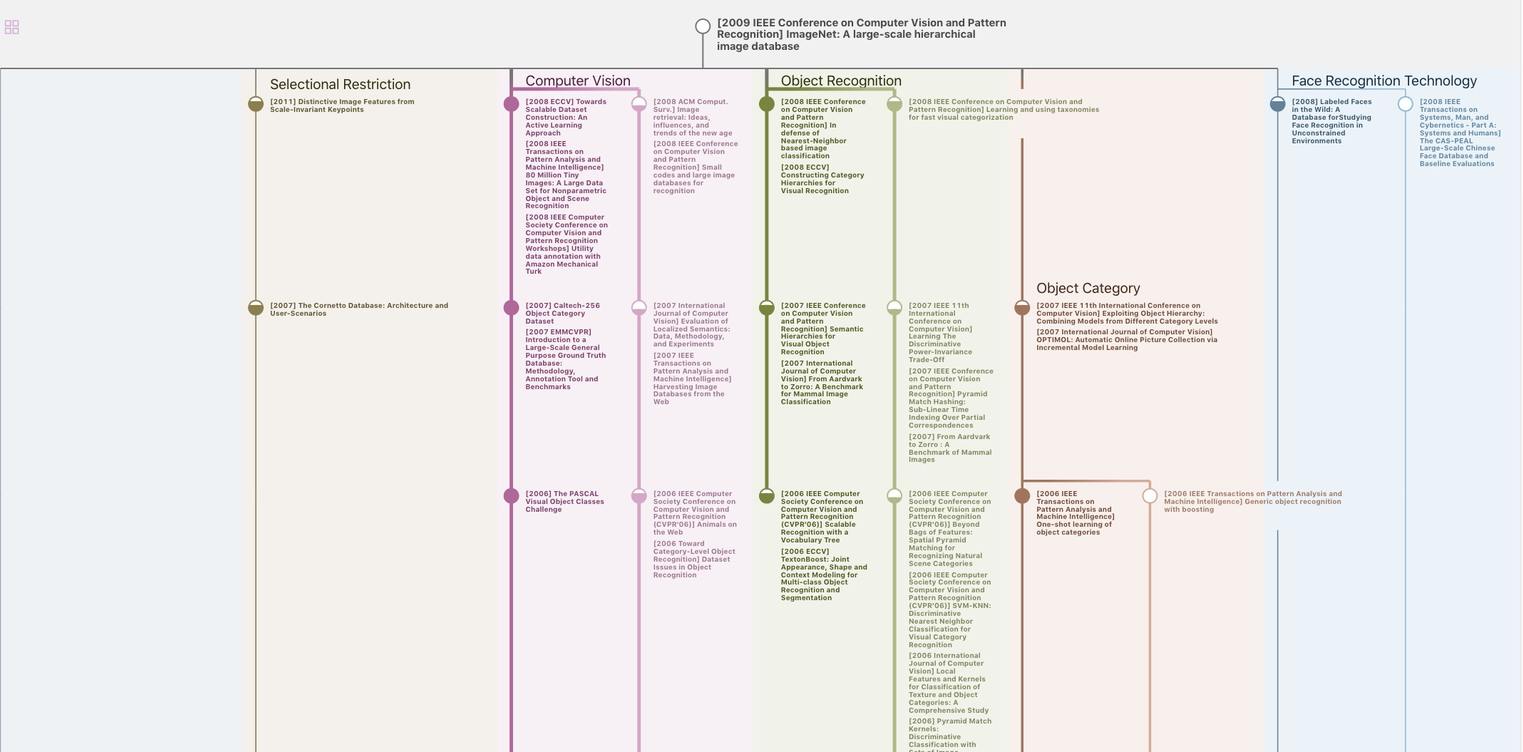
生成溯源树,研究论文发展脉络
Chat Paper
正在生成论文摘要