Forest Reranking through Subtree Ranking
EMNLP-CoNLL '12: Proceedings of the 2012 Joint Conference on Empirical Methods in Natural Language Processing and Computational Natural Language Learning(2012)
摘要
We propose the subtree ranking approach to parse forest reranking which is a generalization of current perceptron-based reranking methods. For the training of the reranker, we extract competing local subtrees, hence the training instances (candidate subtree sets) are very similar to those used during beam-search parsing. This leads to better parameter optimization. Another chief advantage of the framework is that arbitrary learning to rank methods can be applied. We evaluated our reranking approach on German and English phrase structure parsing tasks and compared it to various state-of-the-art reranking approaches such as the perceptron-based forest reranker. The subtree ranking approach with a Maximum Entropy model significantly outperformed the other approaches.
更多查看译文
关键词
subtree ranking approach,current perceptron-based reranking method,forest reranking,reranking approach,various state-of-the-art reranking,candidate subtree set,beam-search parsing,perceptron-based forest reranker,training instance,English phrase structure,Forest reranking
AI 理解论文
溯源树
样例
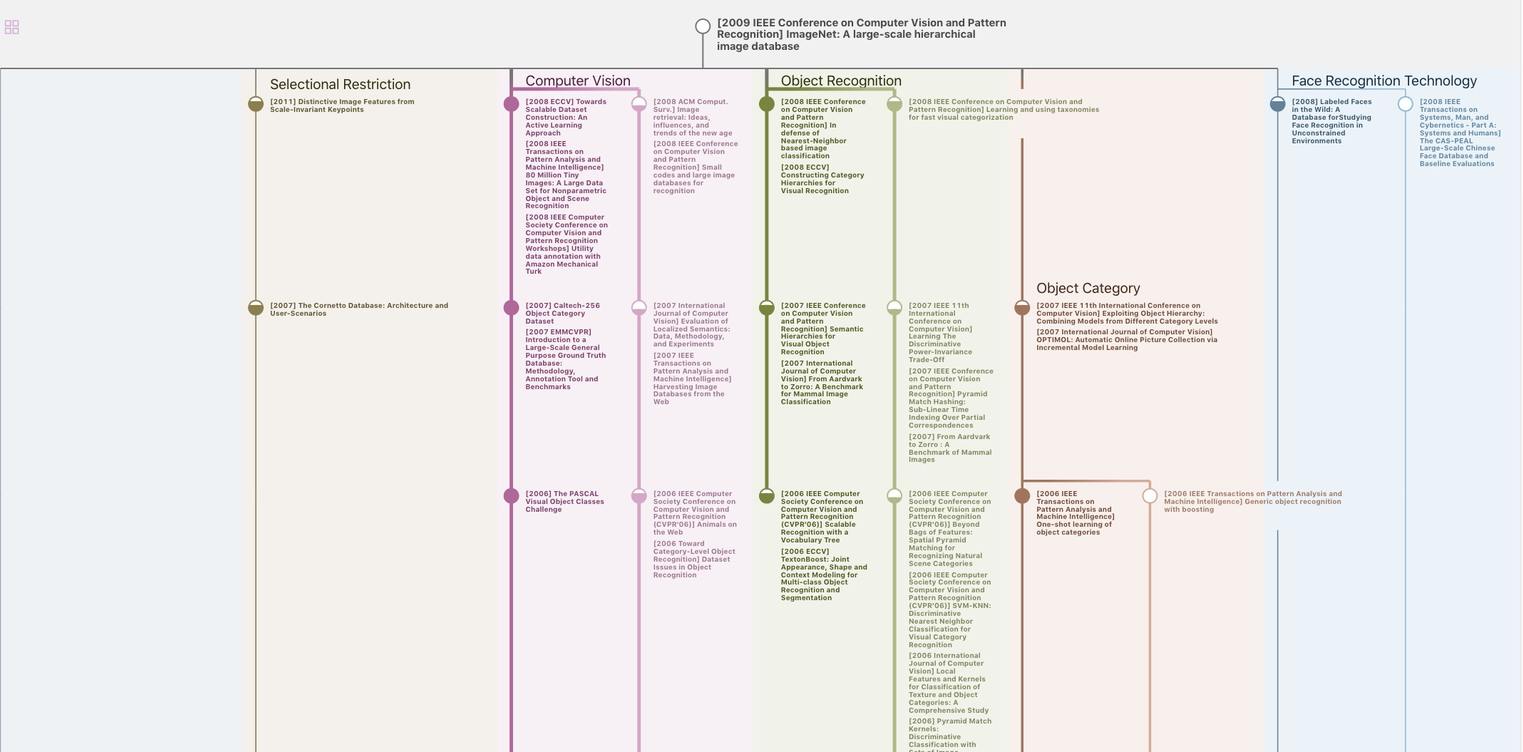
生成溯源树,研究论文发展脉络
Chat Paper
正在生成论文摘要