Classifying tag relevance with relevant positive and negative examples.
MM '13: ACM Multimedia Conference Barcelona Spain October, 2013(2013)
摘要
Image tag relevance estimation aims to automatically determine what people label about images is factually present in the pictorial content. Different from previous works, which either use only positive examples of a given tag or use positive and random negative examples, we argue the importance of relevant positive and relevant negative examples for tag relevance estimation. We propose a system that selects positive and negative examples, deemed most relevant with respect to the given tag from crowd-annotated images. While applying models for many tags could be cumbersome, our system trains efficient ensembles of Support Vector Machines per tag, enabling fast classification. Experiments on two benchmark sets show that the proposed system compares favorably against five present day methods. Given extracted visual features, for each image our system can process up to 3,787 tags per second. The new system is both effective and efficient for tag relevance estimation.
更多查看译文
AI 理解论文
溯源树
样例
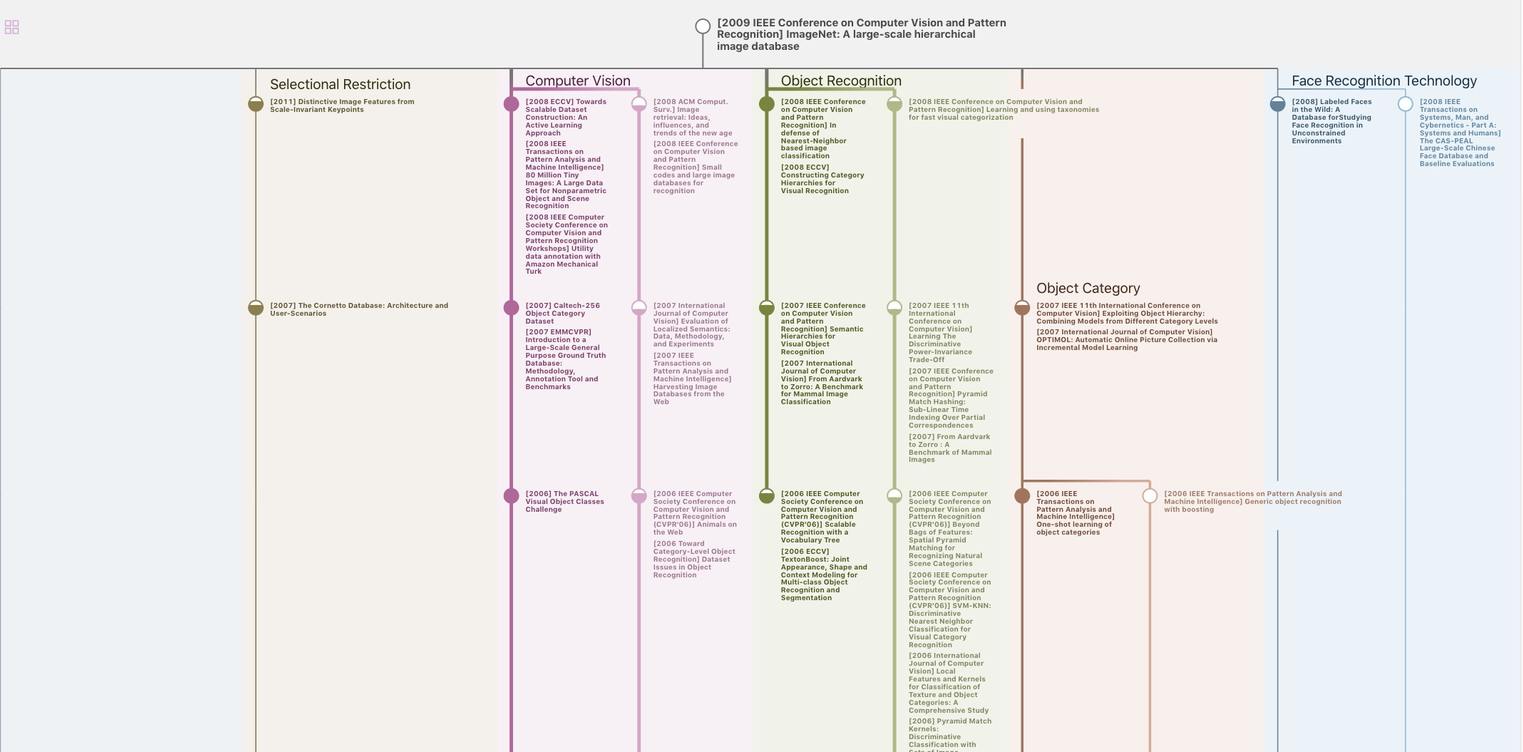
生成溯源树,研究论文发展脉络
Chat Paper
正在生成论文摘要