Know Your Enemy, Know Yourself: Block-Level Network Behavior Profiling and Tracking
IEEE Global Telecommunications Conference (Globecom)(2010)
摘要
Gaining a better knowledge of one's own network is crucial to effectively manage and secure today's large, diverse campus and enterprise networks. Because of the large number of IP addresses (or hosts) and the prevalent use of dynamic IP addresses, profiling and tracking individual hosts within such large networks may not be effective nor scalable. In this paper, we develop a novel methodology for capturing, characterizing, and tracking network activities at the block-level by carefully selecting a port feature vector and capturing the port activities of individual hosts within a block using a block-wise (host) port activity matrix (BPAM). Applying the SVD low-rank approximation technique, we obtain a low-dimensional subspace representation which captures the significant and typical host activities of the block. Using these subspace representations, we cluster and classify blocks to provide high-level descriptive labels to assist network operators and security analysts to gain insight into the network activities. We also develop novel methods to track and quantify changes in blocks' behaviors over time, and demonstrate how these methods can be utilized to identify major changes and anomalies within the network.
更多查看译文
关键词
web server,low rank approximation,indexes,security,feature vector
AI 理解论文
溯源树
样例
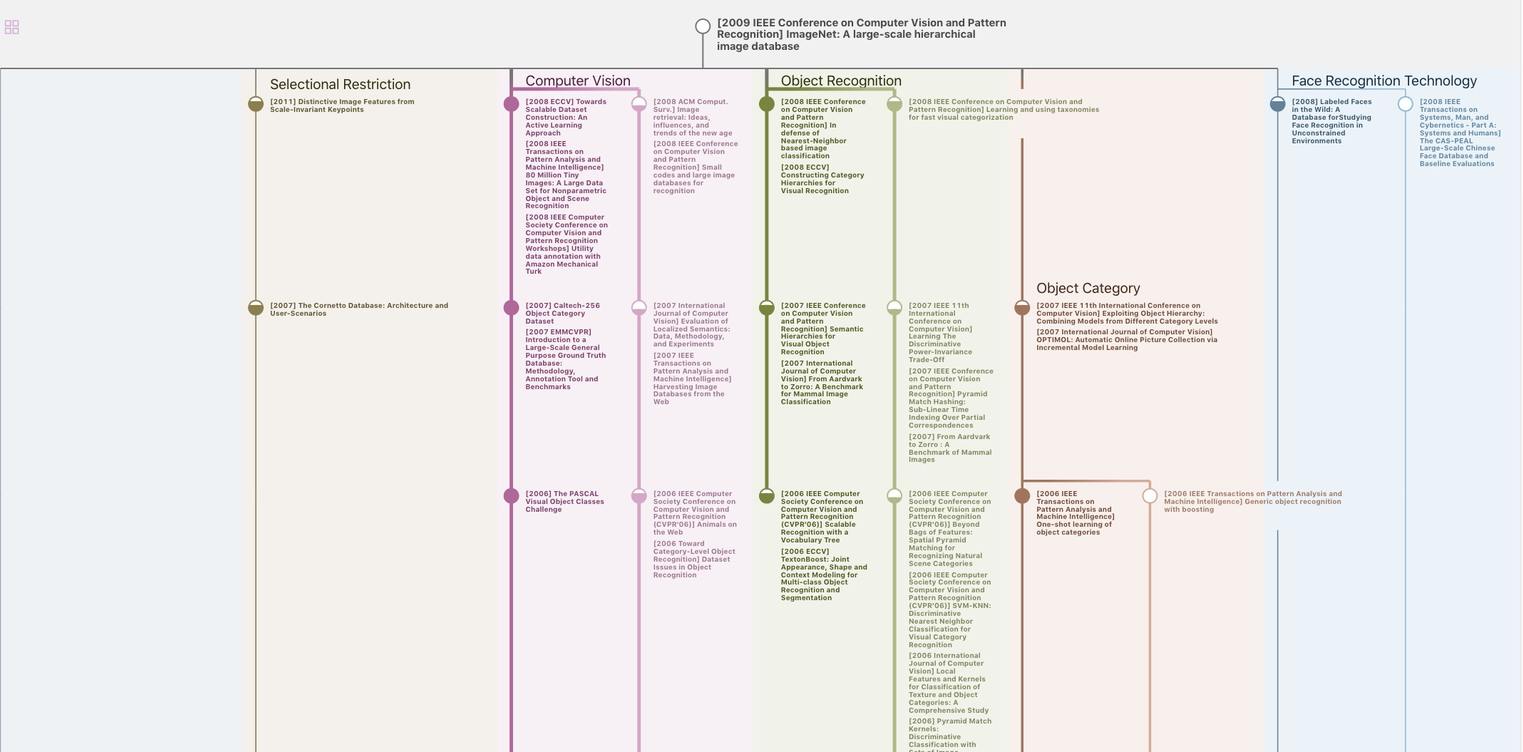
生成溯源树,研究论文发展脉络
Chat Paper
正在生成论文摘要