Weakly Correlated Multimodal Sentiment Analysis: New Dataset and Topic-Oriented Model
IEEE Transactions on Affective Computing(2024)
摘要
Existing multimodal sentiment analysis models focus more on fusing highly correlated image-text pairs, and thus achieves unsatisfactory performance on multimodal social media data which usually manifests weak correlations between different modalities. To address this issue, we first build a large multimodal social media sentiment analysis dataset RU-Senti which contains more than 100,000 image-text pairs with sentiment labels. Then, we proposed a topic-oriented model (TOM) which assumes that text is usually related to a certain portion of the image contents and significant variances exist in sentiment distribution across diverse topics. TOM learns the topic information from textual content and designs a topic-oriented feature alignment module to extract textual semantics correlated information from images, thus achieving the alignment between two modalities. Then, TOM utilizes a transformer encoder initialized with the parameters from a pre-trained vision-language model to fuse the multimodal features for sentiment prediction. According to the experiments over the public MVSA-Multiple dataset and our RU-Senti dataset, RU-Senti is of high suitability for studying weakly correlated multimodal sentiment analysis, and the proposed TOM model also largely outperforms the SOTA mulitimodal sentiment analysis methods and pre-trained vision-language models. The RU-Senti dataset and the code of TOM are available at
https://github.com/PhenoixYANG/TOM
.
更多查看译文
关键词
Image-text alignment,multimodal sentiment analysis,topic-oriented analysis,weak correlation
AI 理解论文
溯源树
样例
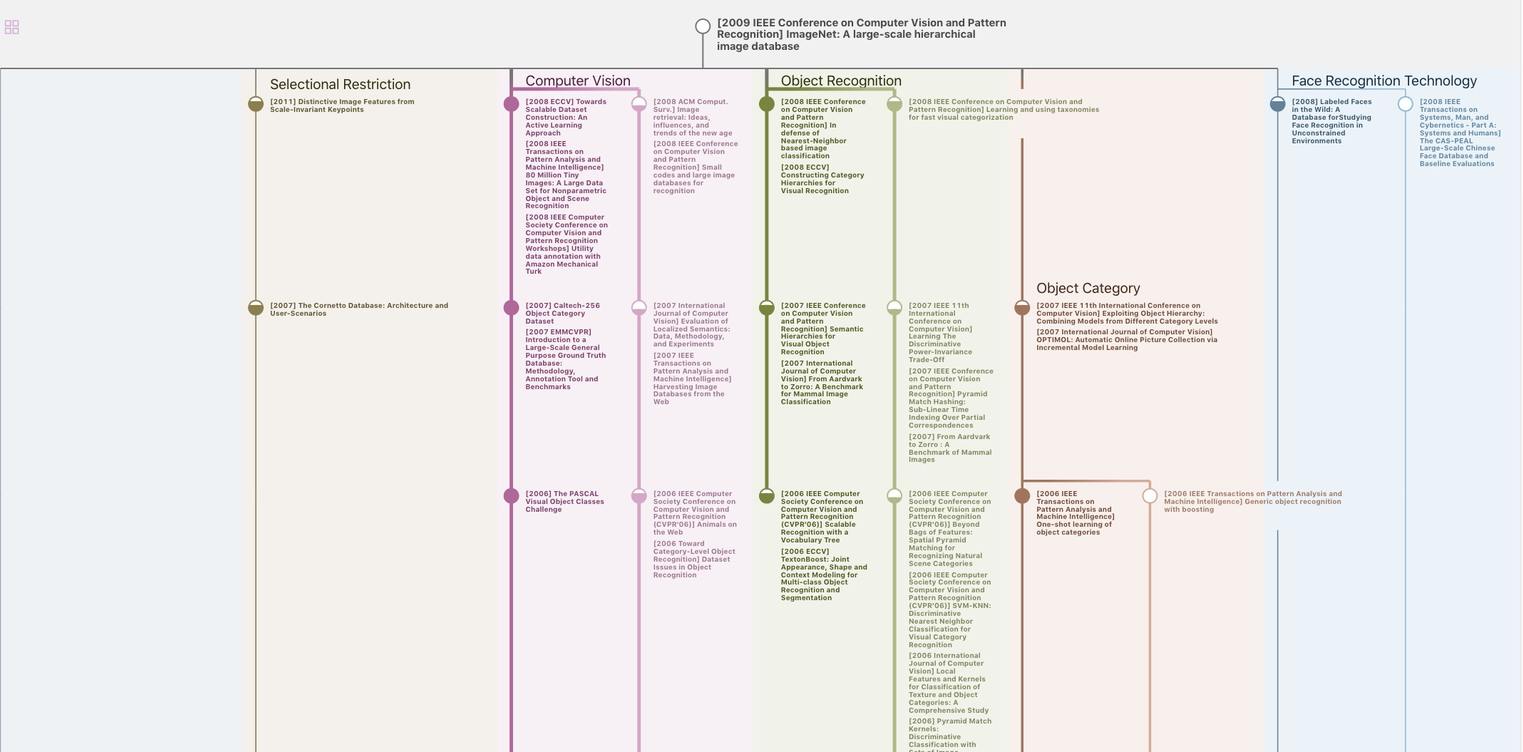
生成溯源树,研究论文发展脉络
Chat Paper
正在生成论文摘要