Optimal node perturbation in linear perceptrons with uncertain eligibility trace.
Neural Networks(2010)
摘要
Node perturbation learning has been receiving much attention as a method for achieving stochastic gradient descent. As it does not require direct gradient calculations, it can be applied to a reinforcement learning framework. However, in conventional node perturbation learning, the residual error due to perturbation is not eliminated even after convergence. Using infinitesimal perturbations suppresses the residual error, but such perturbations are less robust against uncertainty and noise in an eligibility trace, which is a memory of perturbation and input. We derive an optimal parameter schedule for node perturbation learning used with linear perceptrons with uncertainty in the eligibility trace. Our adaptive learning rule resolves the trade-off between robustness against the uncertainty and residual error reduction. The results obtained will be useful in designing learning rules and interpreting related biological knowledge.
更多查看译文
关键词
Node perturbation,Uncertainty,Linear perceptron,Learning curve,Residual error
AI 理解论文
溯源树
样例
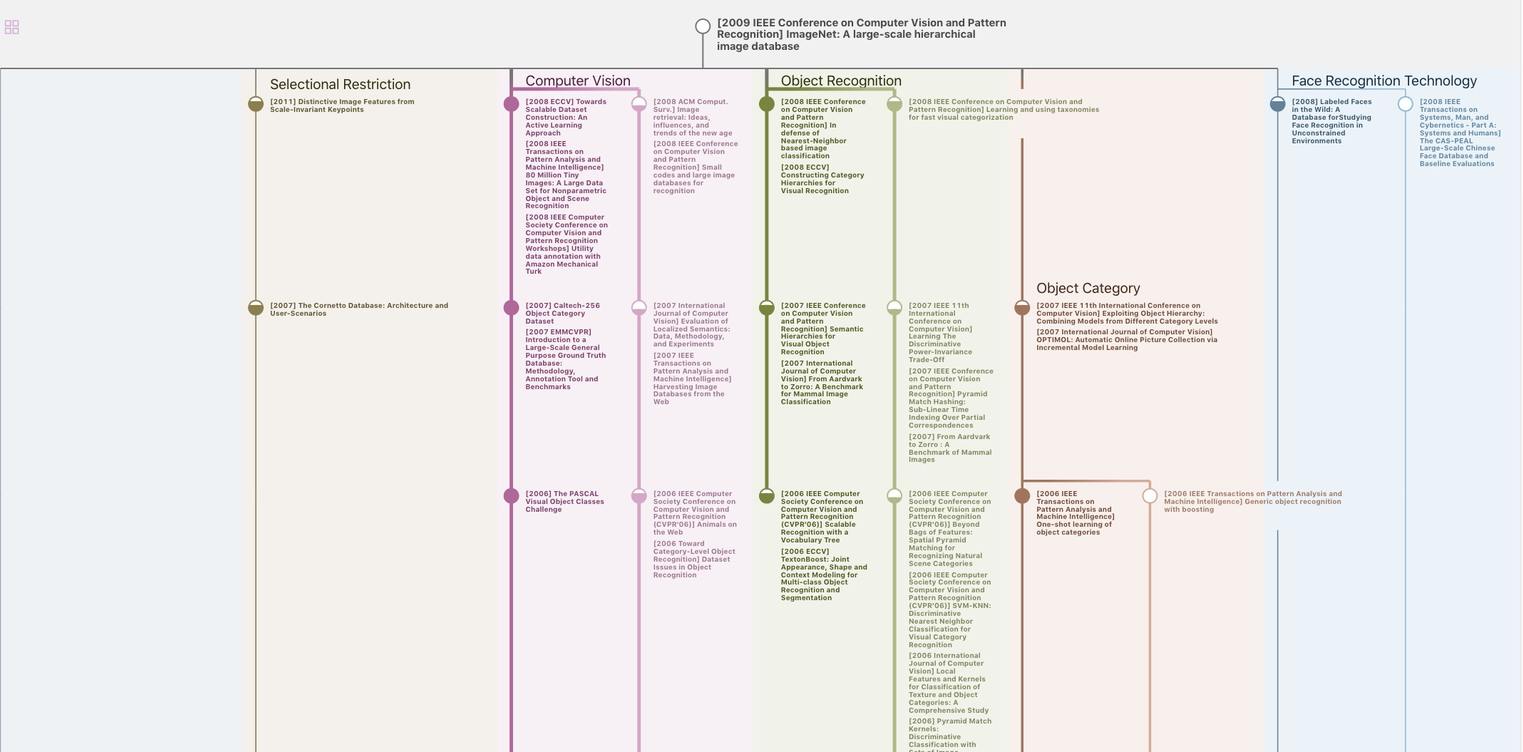
生成溯源树,研究论文发展脉络
Chat Paper
正在生成论文摘要