Constrained DTW preserving shapelets for explainable time-Series clustering
Pattern Recognit.(2023)
摘要
The analysis of time series is becoming ever more popular due to the proliferation of sensors. A well-known similarity measure for time-series is Dynamic Time Warping (DTW), which does not respect the axioms of a metric. These, however, can be reintroduced through Learning DTW-Preserving Shapelets (LDPS). This article extends LDPS and presents constrained DTW-preserving shapelets (CDPS). CDPS directs the time-series repre-sentation to captures the user's interpretation of the data by considering a limited amount of user knowledge in the from of must-link-cannot link constraints. Subsequently, unconstrained algorithms can be used to generate a clustering that respects the constraints without explicit knowledge of them. Out-of-sample data can be trans-formed into this space, overcoming the limitations of traditional transductive constrained-clustering algorithms. Furthermore, several Shapelet Cluster Explanation (SCE) approaches are proposed that explain the clustering and can simplify the representation while preserving clustering performance. State-of-the-art performance is demonstrated on multiple time-series datasets and an open-source implementation will be made publicly available upon acceptance.
更多查看译文
关键词
Shapelets,Semi-supervised learning,Constrained clustering,Time-series,Representation learning
AI 理解论文
溯源树
样例
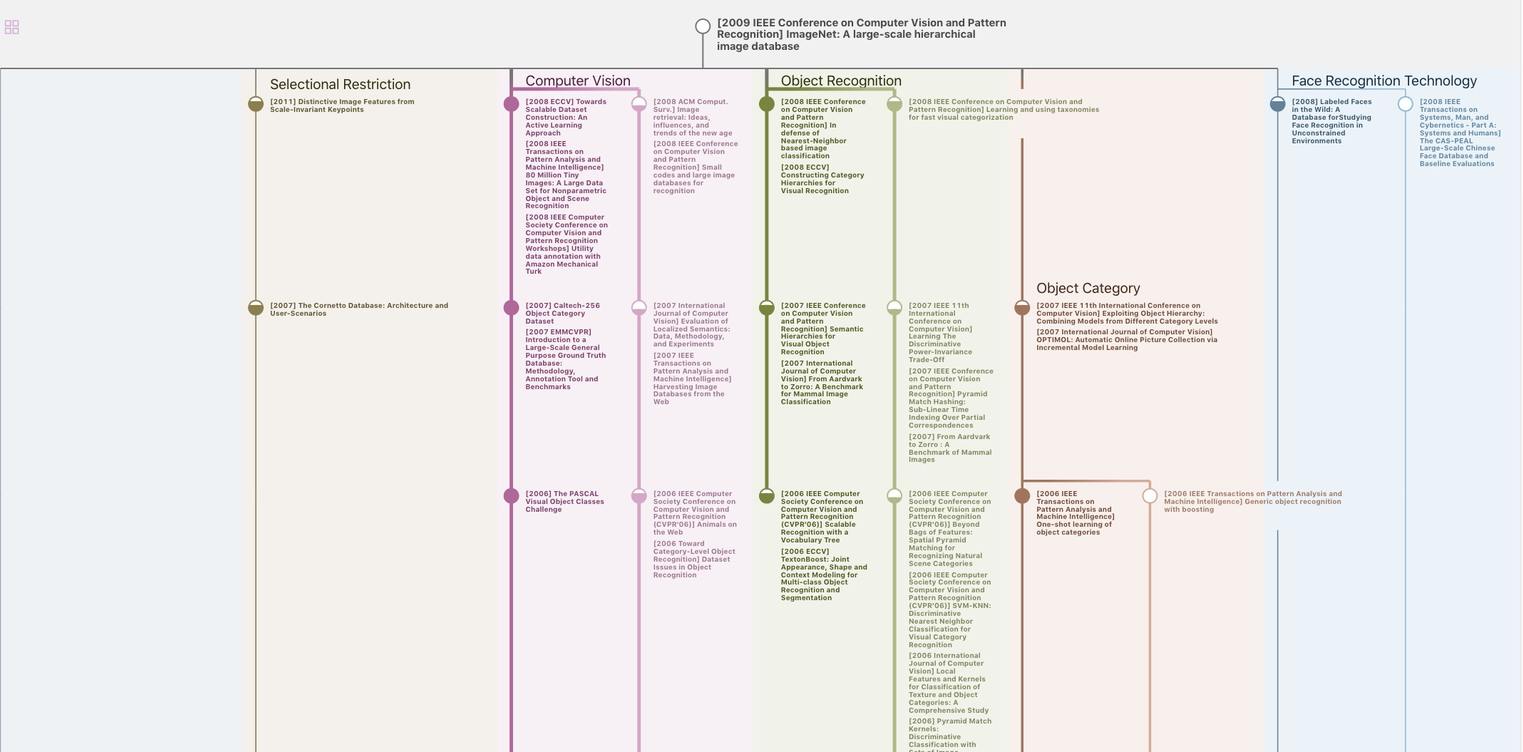
生成溯源树,研究论文发展脉络
Chat Paper
正在生成论文摘要