Compact Feedforward Sequential Memory Networks for Large Vocabulary Continuous Speech Recognition
17TH ANNUAL CONFERENCE OF THE INTERNATIONAL SPEECH COMMUNICATION ASSOCIATION (INTERSPEECH 2016), VOLS 1-5: UNDERSTANDING SPEECH PROCESSING IN HUMANS AND MACHINES(2016)
摘要
In acoustic modeling for large vocabulary continuous speech recognition, it is essential to model long term dependency within speech signals. Usually, recurrent neural network (RNN) architectures, especially the long short term memory (LSTM) models, are the most popular choice. Recently, a novel architecture, namely feedforward sequential memory networks (FSMN), provides a non-recurrent architecture to model long term dependency in sequential data and has achieved better performance over RNNs on acoustic modeling and language modeling tasks. In this work, we propose a compact feedforward sequential memory networks (cFSMN) by combining FSMN with low-rank matrix factorization. We also make a slight modification to the encoding method used in FSMNs in order to further simplify the network architecture. On the Switchboard task, the proposed new cFSMN structures can reduce the model size by 60% and speed up the learning by more than 7 times while the models still significantly outperform the popular bidirection LSTMs for both frame-level cross-entropy (CE) criterion based training and MMI based sequence training.
更多查看译文
关键词
feedforward sequential memory networks,compact FSMN,speech recognition,low rank factorization,sequence training
AI 理解论文
溯源树
样例
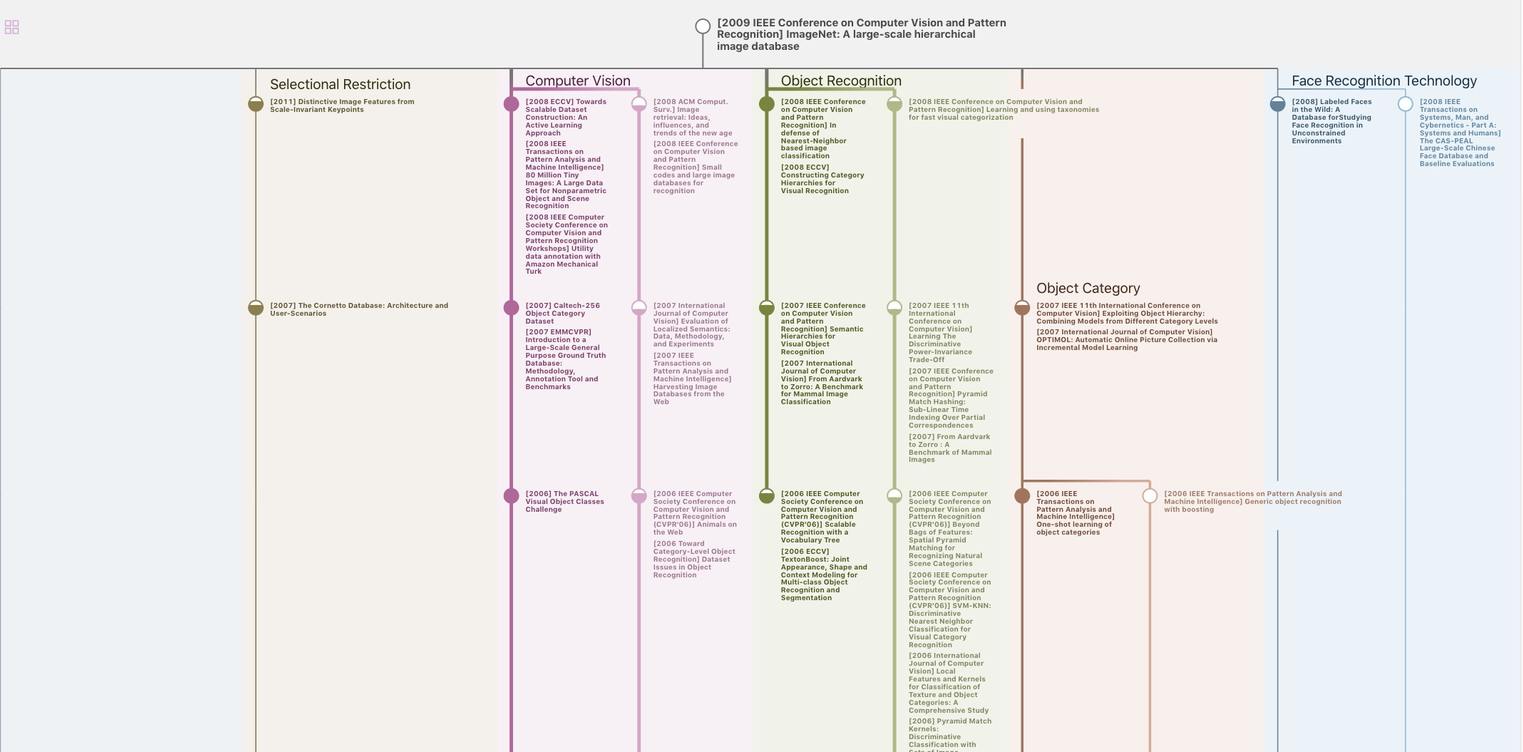
生成溯源树,研究论文发展脉络
Chat Paper
正在生成论文摘要